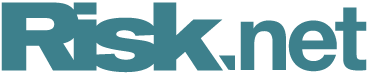
Risk Technology Awards 2019: Making machines more helpful
Machine learning can be too efficient; now, vendors are looking for ways to make it more accurate. Clive Davidson looks at the stories behind this year’s Risk Technology Awards
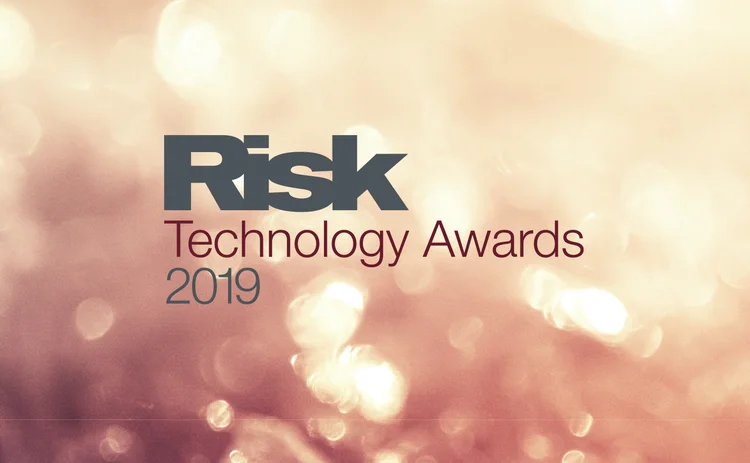
Machine learning can be too efficient; now, vendors are looking for ways to make it more accurate. Clive Davidson looks at the stories behind this year’s Risk Technology Awards
One of the attractive things about machine learning, in theory, is that it approaches tasks in a more human way – checking new information against past experience and getting smarter as it goes – while also having a superhuman capacity to process that data. It can be too efficient, though.
When applied in the world of risk monitoring, the always-on, always-willing, never-tired machines can generate more alerts than their human colleagues are able to investigate. Some of the winning vendors in this year’s Risk Technology Awards (RTAs) have been trying to solve this problem, creating something of a cognitive revolution, where humans and machines work side by side to tackle some of the biggest challenges to the safe and stable operations of financial markets and services.
Full list of winners
Bank ALM system of the year
Moody’s Analytics
Best vendor for innovation
IBM
Best vendor for systems support and implementation
Quantifi
Credit data provider of the year
Moody’s Analytics
Credit stress-testing product of the year
Prometeia
Cyber risk/security product of the year
Gurucul
Enterprise-wide stress-testing product of the year
Moody’s Analytics
Financial crime product of the year
IBM
GRC product of the year
MetricStream
IFRS 9 – ECL modelling solution of the year
Moody’s Analytics
IFRS 9 – enterprise solution of the year
Moody’s Analytics
Managed support services provider of the year
Broadridge Financial
Market surveillance product of the year
Eventus Systems
Model validation service of the year
Yields.io
Op risk modelling vendor of the year
The Analytics Boutique
Regulatory capital calculation product of the year
IHS Markit
Regulatory reporting system of the year
Wolters Kluwer
Risk dashboard software of the year
The Technancial Company
Wholesale credit modelling software of the year
Moody’s Analytics
One example comes from the world of central limit order book trading, where market participants are under pressure to identify and catch spoofing and layering – strategies in which traders issue non-genuine orders to mislead others as to the level of supply or demand.
“As an industry, we are having more spoofing incidents in both electronic and manual high-touch trading,” remarked one member of the RTA judging panel.
The problem with spoofing and layering is that they are complex and evolving behaviours that cannot be identified with a simple single check. The Validus platform from Texas-based Eventus Systems provides tens of parameters that can help identify spoofing and other illicit behaviours, but this isn’t a cure-all – setting the parameters widely enough to capture spoofing and other forms of manipulation can generate thousands of alerts a day, overwhelming the ability of most institutions to follow up. So, Eventus has trained machines to sift through the alerts and prioritise those that need the most urgent attention.
Eventus uses the results of investigations of alerts by its clients’ human analysts to continuously train a machine learning model to spot likely manipulators. “The model will look through the alerts that have been generated by the rules and come up with the top 10 or 20 that need further investigation, along with confidence levels,” says Travis Schwab, chief executive of Eventus, which won the Market surveillance product of the year award.
Using machines to shortlist candidates for investigation hugely improves efficiency, while generating the original alerts from human-devised rules enables institutions to understand why they are targeting particular traders or firms and explain this to regulators who will not accept ‘black-box’ solutions.
In a recent example, an Eventus client decided to review activity on its trading platform with the view to clean up any undesirable behaviour. It set wide parameters on the Validus system, which then produced thousands of alerts a day – far more than the platform’s three compliance staff could deal with manually. However, the machine learning module was able to identify the most suspect cases, which the compliance staff were able to investigate quickly and terminate a few accounts where there was dubious activity. The result was an immediate drop in the number of alerts.
Insider danger
The combination of artificial intelligence (AI) and data from multiple sources is all about putting events and other pieces of information in context, says Craig Cooper, chief operating officer of California-based Gurucul, which won the Cyber risk/security product of the year award. This enables organisations to see patterns and spot anomalies, and thereby identify – and even predict – risky behaviour. “Traditional analytics tends to be rule-based solutions, focused on known transactional patterns. The power of today’s analytics allows businesses a much wider contextual view, which can be used to identify both known and unknown risky behaviour patterns. This approach results in fewer false positives and reduces investigation time significantly,” says Cooper.
One area where this approach is proving its worth is identifying insider risks. By continuously monitoring employee activity across a number of internal systems, institutions can establish baseline behaviour patterns, or profiles, for individuals and then raise alerts when anomalies show up. This could include suspicious loan approvals, transaction overwrites, emails to competitor domains or unusual physical access to sensitive areas. One Gurucul user was recently able to predict the departure of a disgruntled individual and – potentially – prevent the person from stealing data, committing fraud or sabotaging systems. “An organisation’s insiders – especially those with privileged access to sensitive systems and data – can pose a serious risk to a financial operation,” says Cooper.
Behaviour profiling is an approach IBM also uses in its financial crime solution. IBM Safer Payments can link in all systems through which an organisation interacts with its customers, plus any other relevant sources of information. Safer Payments monitors the data and activity in real time and – using IBM’s Watson AI technology – builds a picture of the behaviour of a customer, or other entity, across all the organisation’s channels of interaction, brands and payment types. The system can take in a wide variety of data, including payments, non-monetary events, and authentication and security data from multiple sources without requiring it to be converted to a fixed format.
“Valuable information is usually lost in these data transformations, which affects both the system’s analytical performance as well as analysts’ ability to effectively work the alerts cases,” says Austin Wells, Watson financial crimes offering manager at IBM, which won the Financial crime product and Most innovative vendor of the year awards. The Safer Payments system is able to piece together both transactional and non-transactional elements from each channel and learns about behaviours over time, using AI as a ‘virtual analyst’ to assist human experts in finding threats and optimising defences against fraud. “But rather than generating a black-box model, the system generates easily readable scenarios as suggestions that the user can choose to deploy,” says Wells.
Back-office bots
The combination of AI and human intelligence is also beginning to find its way beyond the front and middle office.
New York-based Broadridge, which won the Managed support services provider of the year award, expects operations to evolve to the point where experienced and expert humans, performing client-facing roles that can differentiate a company’s services, work alongside machines – or ‘bots’ – of various levels of sophistication that automate repetitive non-differentiating activities.
“We expect these bots to work in either an ‘attended’ fashion with their human co-workers, or unattended, while still being monitored by human co-workers,” says Mike Alexander, head of North American wealth and capital markets solutions for Broadridge. “As bots mature, we expect to see a quantum leap in how services are rendered, from account opening and tax services to trade settlements, money movement and international operations.”
Broadridge has already taken a step in this direction with a product for trade allocations that uses human-assisted machine learning to take in non-standard trade allocations in various formats, such as PDFs, comma-separated value files or emails, and applies pattern-matching algorithms to convert them to allocations its middle-office system can recognise and process. “As more instances of this product are instantiated across our client base we expect to be able to get these machines to share patterns across each other rather than being assisted by human labour,” says Alexander.
Technology providers are also starting to investigate a cognitive approach to systems implementation and support. “We are looking to use AI to automate and provide a better service for both support and operations,” says Rohan Douglas, chief executive at New Jersey-based Quantifi, which won the Best vendor for systems support and implementation category. This could include AI tools for implementation configurations, as well as automation to provide quick responses to system issues, and system monitoring for pre-emptive actions to avoid issues. “The idea is to keep the personal support, but supplement it with tools to make the people more effective,” says Douglas.
But there are inherent dangers in the current enthusiasm for AI-based modelling, especially where there is a close coupling of models and their developers, warns Jos Gheerardyn, chief executive and co-founder of Yields.io, which won the Model validation service of the year award. With an abundance of commercial and open-source analytical and AI tools now available, it can be quick and easy to develop models for a variety of applications. However, he warns the industry not to forget the statistician’s aphorism that ‘all models are wrong; some are useful’, and to prioritise model risk management.
To highlight the issue, Gheerardyn points to the interdependency between humans and models often found in front offices. “Many front-office quant teams in banks often become an indivisible part of their own analytics. These teams are constantly needed to fix issues as they appear, to fine-tune calibrations and perform small modifications. When the quants are gone, the models have to shut down,” says Gheerardyn.
This hybrid ‘human-algo’ approach is not sustainable given the rapid growth of models in financial institutions. What is needed is a methodology that from the outset takes into account that a model will at some point fail. “To manage that risk, the design of the model should focus on risk management, studying data quality, quantifying model risk and determining the feasibility of monitoring,” says Gheerardyn.
These factors should be weighed against the potential benefits of the model, as well as the risk appetite of the bank, enabling the institution to choose the most appropriate solution – a complex model, a simple one, or no model at all. “This exercise at the beginning of the cycle will yield a design that allows for models to be deployed in a robust fashion with clearly defined limits that can be monitored and managed in a completely automated fashion.”
The combination of machines, models and big data means institutions now have not only tools for automating mundane repetitive tasks, but tools to aid seeing the big picture and prioritising where human expertise can be most productively directed. But as machines take on more responsibility, they must be subject to the same rigours of risk management as their human colleagues.
‘R’ is for modelling
This year’s awards also provided further evidence of the shift towards open-source technology – including the increasing use of the R programming language for modelling.
“While IT departments looking for an end-user computing language tend to prefer Python – which is developer-led and has established tool chain support – R remains the language of choice for the majority of statisticians and is often used by desk quants,” says Ian Green, an independent consultant and member of the RTAs judging panel. “Over recent years R has developed significantly and now offers a powerful platform for [transforming], analysing, charting and presenting data.”
New York-based AxiomSL supports R along with other modelling tools including Excel and Java for use with its risk and compliance management systems. “We have had native R functionality for over seven years. We can easily push model parameters and information into and out of R code while maintaining traceability of data,” says Richard Moss, global product manager for capital and liquidity, at AxiomSL.
In a recent implementation of its ControllerView platform system for International Financial Reporting Standard 9 compliance, a bank required its suite of Excel models for probability of default, loss given default and macroeconomic scenarios to be migrated to R. “The bank was looking for a flexible, industry standard code base that can scale well and be easily repurposed,” says Moss. AxiomSL’s implementation team was able to migrate the bank’s models to produce the same results as under Excel, with full documentation that met the local regulator’s approval.
Migration to R proved to have further benefits. “In AxiomSL’s R-based environment, the model assumptions and calculations, previously hidden in the deeply layered Excel environment and lacking documentation, suddenly became obvious and accessible. The opportunity to examine the migrated models with fresh eyes led the bank to re-evaluate its assumptions and make improvements that can enhance expected credit loss steering and the bank’s overall strategic and operational decision-making,” says Moss.
The adoption of R is part of a trend away from costly proprietary tools towards more open software, says AxiomSL. “Banks are moving toward open-source tools that have strong statistical libraries, data fundamentals and performance optimisation,” says Moss.
Moody’s Analytics has also recognised that more institutions are choosing to develop models in R, and its Scenario Analyzer stress-testing framework supports models developed in a number of scripting languages.
“Several organisations have supplemented the models developed in the Scenario Analyzer framework with R or Python script models. As a result, banks gain the flexibility to use existing investments in technology, as well as their own organisational capabilities, while adhering to their internal technology policies,” says Steve Tulenko, executive director, enterprise risk solutions at Moody’s Analytics, which won six awards including Enterprise-wide stress-testing product of the year. Moody’s Analytics is also now using R and the R Shiny development package for some of its own product developments.
Green adds that R can also now be used for hosting interactive websites, accessing cloud databases, writing HTTP servers and running machine learning algorithms that require parallelisation.
Methodology
Technology vendors were invited to pitch their products and services in 23 enterprise, credit and operational risk categories. Candidates were required to answer a set of questions within a maximum word count about how their technology met industry needs, its differentiating factors and recent developments. A total of 133 entries were received.
A panel of 10 industry experts and Risk.net editorial staff reviewed the shortlisted entries, with judges recusing themselves from categories or entries where they had a conflict of interest or no direct experience. The judges scored and commented on the shortlisted entrants. The majority of the judges met to review the scores and, after robust discussion, made final decisions on the winners. Where there was no credible winning candidate, the category was scrapped. In total, 19 awards were given this year.
The judges
Amit Lakhani, Head of operational risk controls for information communication technology and third-party management for corporate and institutional banking, BNP Paribas
Deborah Hrvatin, Managing director and global head of institutional clients group operational risk management, Citi
Glenna Hagopian, Chief conduct officer and head of enterprise risk management (ERM), Citizens Financial Group
Hugh Stewart, Adviser, Chartis Research
Ian Green, Chief executive, eCo Financial Technology
Matt Sulkey, Managing director and head of ERM framework and governance, TIAA
Peter Quell, Head of portfolio analytics for market and credit risk, DZ Bank
Sid Dash, Research director, Chartis Research
Clive Davidson, Contributing editor, Risk.net
Duncan Wood, Global editorial director, Risk.net
Only users who have a paid subscription or are part of a corporate subscription are able to print or copy content.
To access these options, along with all other subscription benefits, please contact info@risk.net or view our subscription options here: http://subscriptions.risk.net/subscribe
You are currently unable to print this content. Please contact info@risk.net to find out more.
You are currently unable to copy this content. Please contact info@risk.net to find out more.
Copyright Infopro Digital Limited. All rights reserved.
You may share this content using our article tools. Printing this content is for the sole use of the Authorised User (named subscriber), as outlined in our terms and conditions - https://www.infopro-insight.com/terms-conditions/insight-subscriptions/
If you would like to purchase additional rights please email info@risk.net
Copyright Infopro Digital Limited. All rights reserved.
You may share this content using our article tools. Copying this content is for the sole use of the Authorised User (named subscriber), as outlined in our terms and conditions - https://www.infopro-insight.com/terms-conditions/insight-subscriptions/
If you would like to purchase additional rights please email info@risk.net
More on Awards
Joining the dots: banks leverage tech advancements for the future of regulatory reporting
The continued evolution of regulatory frameworks is creating mounting challenges for capital markets firms in achieving comprehensive and cost-effectiveawa compliance reporting. Regnology discusses how firms are starting to use a synthesis of emerging…
Markets Technology Awards 2024 winners' review
Vendors spy opportunity in demystifying and democratising – opening up markets and methods to new users
Derivatives house of the year: JP Morgan
Risk Awards 2024: Response to regional banking crisis went far beyond First Republic
Risk Awards 2024: The winners
JP Morgan wins derivatives house, lifetime award for El Karoui, Barclays wins rates
Best product for capital markets: Murex
Asia Risk Awards 2023
Technology vendor of the year: Murex
Asia Risk Awards 2023
Best structured products support system: Murex
Asia Risk Awards 2023
Energy Risk Asia Awards 2023: the winners
Winning firms demonstrate resiliency and robust risk management amid testing times
Most read
- Basel Committee reviewing design of liquidity ratios
- Breaking out of the cells: banks’ long goodbye to spreadsheets
- Too soon to say good riddance to banks’ public enemy number one