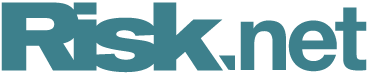
Podcast: Fries on Monte Carlo, Greeks and the future of AAD
Research on AAD is not complete until it becomes easier to implement, says quant
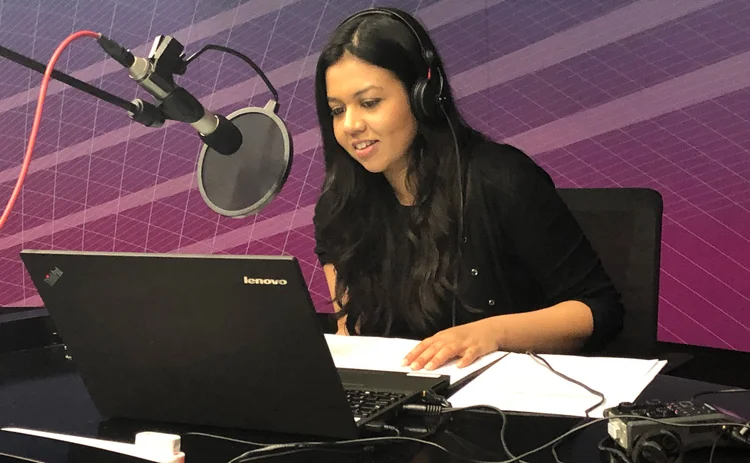
In this month’s Quantcast, Christian Fries, head of model development and methodology at DZ Bank in Frankfurt and a professor of mathematical finance at LMU University, talks about the qualities to look out for in algorithmic techniques, and the importance of simple and clean codes when developing good algorithms.
Fries introduces his new paper, Automatic backward differentiation for American Monte Carlo, which applies adjoint algorithmic differentiation (AAD) to calculate the sensitivities of Bermudan options and valuation adjustments in a quicker way.
Two key advantages of his technique include the ability to avoid a cumbersome regression step in the calculation of the sensitivities, and the fact that it is easy to code.
Fries says he doesn’t like debates around which algorithm is faster than the other, and that the more important results to look out for are whether an algorithm manages to perform within a given order of magnitude and whether it is straightforward to implement.
“What matters is if an algorithm performs within seconds, minutes, hours or days. It’s not so important if it performs within one second or four seconds…code complexity is a big issue. For example, in the industry, if you are heading a team, it is very important the code is clean, easy to understand and does not have many complicated special cases.”
He adds there are still outstanding issues with AAD in that it is difficult to code and not easy to develop on legacy systems.
“When I started to look at AAD I thought everything was done there, and to my surprise, [found that] there is a little bit more that can be done,” says Fries.
He added that in the future he will be working on a paper on obtaining forward sensitivities for calculating margin valuation adjustment using AAD, and another follow-up paper looking at how to approximate those sensitivities without the use of AAD.
Index
00:00 Introduction
02:30 Fundamental Review of the Trading Book
03:00 Discussion of previous paper on Monte Carlo method
04:24 Forthcoming paper
08:42 How AAD is adapted to Greeks calculation
11:40 Improvements brought by the methodology
14:26 Model performance
17:10 Where does the technique apply?
18:30 Option price boundaries
23:00 How the model is used at the bank
23:40 Future research
25:54 AAD’s potential still to be discovered
To hear the full interview, listen in the player above, or download. Future podcasts in our Quantcast series will be uploaded to Risk.net. You can also visit the main page here to access all tracks or go to the iTunes store to listen and subscribe.
Only users who have a paid subscription or are part of a corporate subscription are able to print or copy content.
To access these options, along with all other subscription benefits, please contact info@risk.net or view our subscription options here: http://subscriptions.risk.net/subscribe
You are currently unable to print this content. Please contact info@risk.net to find out more.
You are currently unable to copy this content. Please contact info@risk.net to find out more.
Copyright Infopro Digital Limited. All rights reserved.
You may share this content using our article tools. Printing this content is for the sole use of the Authorised User (named subscriber), as outlined in our terms and conditions - https://www.infopro-insight.com/terms-conditions/insight-subscriptions/
If you would like to purchase additional rights please email info@risk.net
Copyright Infopro Digital Limited. All rights reserved.
You may share this content using our article tools. Copying this content is for the sole use of the Authorised User (named subscriber), as outlined in our terms and conditions - https://www.infopro-insight.com/terms-conditions/insight-subscriptions/
If you would like to purchase additional rights please email info@risk.net
More on Markets
Canada’s triparty repo launch aims to fill C$60bn void
Test trades on TMX/Clearstream platform represent “quantum leap” for creaking funding markets
SG trader dismissals shine spotlight on intraday limit controls
Risk experts say many banks rely on daily reports and can’t effectively monitor intraday limits in real time
Hedge funds’ pricing often trumps other buy-siders – SNB
Research shows “advantageous” prices result in outperformance of 139bp trading USD/CHF
Softer FX rules for China QFIs set to boost CNY competition
Freedom to circumvent local custodians a plus for pricing and best execution – State Street
Allianz Life drops single-name CDS positions in Q4
Counterparty Radar: US life insurance industry volumes sink to lowest level in two years
Sustainable bond markets miss an options trick
A derivatives mindset could boost lagging sustainability-linked market, argues climate think-tank
FX dealers face end-of-day trading stress from T+1 shift
Experts say switch to using overnight swaps could be “problematic” and lead to wider spreads
Consortium backs BGC’s effort to challenge CME
Banks and market-makers – including BofA, Citi, Goldman, Jump and Tower – will have a 26% stake in FMX
Most read
- For a growing number of banks, synthetics are the real deal
- Podcast: Olivier Daviaud on P&L attribution for options
- SG trader dismissals shine spotlight on intraday limit controls