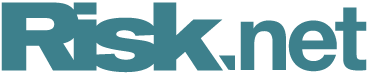
This article was paid for by a contributing third party.More Information.

All along the watchtower – Surveillance tools against market abuse
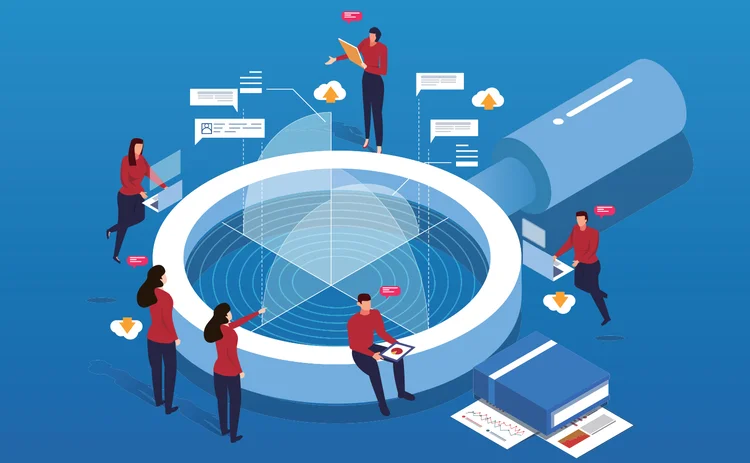
Surveillance tools against market abuse are enjoying a technological revolution in analytics, while anxious supervisors are also closing in on market practices. Risk.net hosted a webinar in association with NICE Actimize to analyse the threats and opportunities
The panel
- Daniel Fernandez, Technical product manager, Compliance, NICE Actimize
- Gautam Sachdev, Managing director, Risk management group, Macquarie
- Moderator: Steve Marlin, Staff writer, Risk management, Risk.net
Insider trading and market abuse shine a spotlight on banks’ surveillance efforts to protect the financial system from crime. Regulators on both sides of the Atlantic are placing the sector’s compliance efforts under increased scrutiny.
From the perspective of risk managers, data and analytics tools used to meet financial, regulatory and reputational threats are being fuelled by advancing technology, bringing about a revolution in capabilities.
Financial firms are piloting nascent technologies, such as machine learning, behavioural analytics and natural language processing, to transform surveillance of suspicious transactions and communications.
Smarter surveillance is being deployed to identify who puts the firm at risk, detect known forms of market abuse, flag suspicious communications, map previously undetectable patterns and reduce the persistent problem of false positives.

Watchdogs let loose
Will the watchdogs approve of industry efforts? Authorities have stepped up their expectations in recent years. In the US, the Commodity Futures Trading Commission filed 83 enforcement actions in 2018’s fiscal year – a 25% increase over the prior three years – racking up $900 million in civil monetary penalties across 10 cases.
“The regulators have gotten a lot more savvy,” said a senior manager responsible for market abuse surveillance at a large European bank. “They’ve been around long enough to have visited the firms they regulate and to get a good idea of what’s going on in the industry. The holiday is over – if there ever was one – and things have become more serious.”
The legislative landscape the regulators are enforcing has also toughened up in recent years. In Europe, the European Union’s Market Abuse Regulation (MAR) directive is now bedded in and represents an important development for compliance teams.
“One of the challenges it poses to financial institutions is that their processes not only need to identify risks as soon as they occur, or when an actual issue materialises, but they also need to be able to identify intent,” said Daniel Fernandez, technical product manager for compliance at NICE Actimize.
“Therefore, even if an employee is unable to complete a transaction, they need to have technology or processes in place to identify patterns that could demonstrate a financial market participant’s willingness to engage in or benefit from performing a trade or transaction. That means not only looking into possibly unknown patterns of transactions, but also correlating communications at different stages throughout the lifecycle,” Fernandez added.
Today’s rules and models are good at identifying concrete patterns confirmed over time. Machine learning can detect new and alternative patterns, whether in communications or transactions – which would require much longer for human-powered surveillance to notice, if at all.
“Machine learning is a complement to enhance the existing surveillance processes. In the long term, it may start to replace the rules-based models and systems in place today,” Fernandez said.
The previously mentioned bank surveillance manager noted that MAR has already outlined “a whole raft of suspicious behaviours” to scrutinise, but added that this list is neither exhaustive nor finished. “At any time the regulator could come up with new behaviours based on what it has witnessed in the market, and give these new names,” he said.
He pointed to a recent Market Watch newsletter from the UK’s Financial Conduct Authority (FCA), which focused on clamping down on the potential for market abuse arising from publishing incorrect volume data – so-called ‘flying prices’ and ‘printing trades’.
The FCA has said it is concerned about improper use of brokers’ chat systems and trading platforms to advertise prices not supported by a client order (flying), and communicating trades at a volume or price that they claim have been executed, but are in reality fictitious (printing).

The honeymoon is over
Gautam Sachdev, managing director of Macquarie’s risk management group, ties in the FCA’s concerns with the new European regulation since 2016.
“I would agree that the honeymoon is over,” said Sachdev. “The intention to manipulate orders and markets has been front and centre for MAR. This requires firms to move from vanilla surveillance of transactions into more sophistication across multiple data types; it has been a game-changer.”
He pointed to the problem of policing against risks – such as the printing and flying practices that worry the FCA – for over-the-counter (OTC) market transactions, where order management systems are less simple than on exchange platforms.
“That definitely brings an additional layer of challenge,” said Sachdev. “There is increasing expectation to answer the question of how we can co-mingle information between electronic orders, trades and communications to generate a more meaningful output, as opposed to looking at these in separate silos.”
The FCA has also warned about consistency in market surveillance practices – that there is too much of it on display in the form of out-of-the-box alert settings at many banks.
The UK regulator has noted that firms with vendor-supplied systems were using out-of-the-box industry standard settings to calibrate their alert parameters. This could fall foul of MAR demands that each firm’s surveillance procedures are appropriate and proportionate to its scale and the nature of its business.
“There’s no one-stop solution for trade surveillance, even across different asset class types,” Sachdev said. “For equities and futures markets, there are products that are tried and tested, and blessed by the exchanges. The maturity scale is quite different for non-listed OTC products.”
Regulators’ expectations have also moved beyond what was once seen as being ‘enough’. Simple lexicon-based alerts are no longer sufficient to satisfy the leading watchdogs. Behavioural analytics for pattern detection are currently in demand.
Learning machine learning
The bank surveillance manager suggested most firms were yet to get beyond the sandbox stage of developing artificial intelligence (AI), and worried that industry understanding is still too low, with little knowledge or clarity of definitions for the buzzwords ‘AI’ and ‘machine learning’, which are often used interchangeably.
“Brush up on all that before you let it anywhere near your surveillance systems,” the source said. “Some firms are working with the same vendors that promised them alert-based surveillance and that didn’t work out. It’s very much in its infancy, and a case of buyer beware.”
Sachdev agreed the vendor market for AI and machine learning products is still evolving, and that data quality is a potential barrier for any firm investing in state-of-the-art technology.
“A common denominator is the quality of internal data within an organisation and in external market data. Normalising data across asset classes to be machine-understandable is going to be a challenge,” he said.
Fernandez advised standing back and taking a strict problem-solving approach: “To implement any technology – not just machine learning – it’s all about trying to identify what kinds of problems we’re trying to solve, what exactly it is we need to achieve, and then decide whether that particular technology feels useful for it or not,” he said.
Two common industry misconceptions, he stressed, are that machine learning will immediately replace human input, and that the knowledge a compliance analyst has acquired over the years can make quicker or better decisions than an algorithm, and that is why they complement each other.
Instead, an increasing number of transactions, new asset classes, business functions and their users to surveil, the sheer volume of data in play, and the increased demands of regulators, all mean surveillance demands are moving beyond human capabilities, Fernandez explained. A server farm is more adept at processing millions of communications or trade transactions.
Machines are necessary to fill the yawning gap, he said. “The way we see this evolving over time is that machine learning is to become a supplement to the existing process and a way to enhance the workflow of that compliance analyst. It’s about letting humans make the decisions they’re good at, and making machines focus on the task they’re best at,” Fernandez said.
“It’s important to identify use cases where we can say that a machine learning algorithm can, for instance, identify anomalous patterns. Then the analysts can complement that initial step with their institutional expertise to make better decisions while avoiding a focus on reviewing reams of raw data,” he added.
The alternative is increasingly like looking for a needle in a haystack, Sachdev warned. “It’s not going to give 100% true hits, but deploying machine learning would reduce the size of the haystack, making it easier to find the issues firms are looking for, provided they understand their data structure and adopt the best-suited solution, accordingly,” he said.
False positives
High false-positive rates repeatedly come out on top as the biggest problem of banks’ existing surveillance methods. Asked by poll, 60% of the webinar audience chose this issue as their biggest bugbear.
For this reason, the FCA has stressed the importance of calibration and has fined firms for not having well-tuned thresholds, the bank surveillance manager said. Cutting false positives is not an impossible task, but requires courage, he suggested.
“Reducing false positives is a by-product of increased effective surveillance alerts. You should not be tuning to reduce false positives, you should be tuning to increase quality, and you do that by being brave because fortune favours the brave,” he said.
“Test alerts aggressively within the safe space conditions of the sandbox conditions that vendors provide. Get the analysts’ brains to work on analysing alerts so you have thresholds you can believe in, and have those to show before getting sign-off for something that can’t be believed,” the surveillance manager added.
False positive rates vary by asset class, as well as the strategy and scale of an organisation. Less market data transparency increases risk. Equities tend to produce fewer false positives than non-exchange business, for instance, Sachdev noted.
“Lack of market data around pricing contributes to false positives,” the bank surveillance manager added, “such as for OTC derivatives, compared to vanilla exchange-traded stocks.”
Data quality is therefore the start of any solution. Like any other system, the quality of the output is dependent on the data being being fed into it. “Also vital is the ability to centralise data sources so they can be analysed in context,” Fernandez said.
“You can reduce false positives if you’re able to add more context, whether it’s to the rules or the logic or the models that you’re implementing. The industry is moving away from the event-based alerts and transitioning towards an entity-based type of monitoring or alerting,” he continued.
“Instead of analysing each transaction or communication for issues as they come into the engine, consolidating the patterns allow creating a trader-level or employee-level view. By having more context and by combining historical events of previous alerts, we can greatly reduce false positives, because you’re combining them instead of looking at these events in isolation.”
Machine learning can be used to tune parameters more finely over time, representing a good use test for the technology, Fernandez suggested. In this way, it can identify the most problematic areas within a model for producing false positives and recommend changes in tuning.
“Another way is to use machine learning models – particularly supervised – to do a second pass on an initial alert generated by a traditional engine,” he continued. “Such a second model review can look at the historical data, and assign a higher priority to the issue, based on the consistency of a particular individual or trading desk over time.”
For firms keen to harness resources across the organisation, so-called ‘holistic surveillance’ was highlighted by polled audience members as a leading factor in improving compliance programmes in 2019. The bank surveillance manager warned that this buzzword seems to lack definition and that bringing poorly co‑ordinated disparate parts of an organisation together for surveillance purposes could be a recipe for disappointment.
“It sounds suspiciously like ‘one ring to rule them all’ and it’s going to be called ‘holistic surveillance’,” the senior manager responsible for market abuse surveillance said. “It’s definitely doable, but we learned from the banking crisis that if you mix a good bank with a bad bank, you tend to end up with a worse bank. Similarly, if you want to mix in many different components, you’ve got to make sure those components work individually before you start mixing them up in the hope that you’re going to get something better than the sum of its parts.”
Sachdev offered further support. “It’s like a bullseye, and the question concerns the number of concentric circles around it that you want to pull into your holistic surveillance definition,” he said.
For an analyst to action a trade surveillance alert, being able to consider e-commerce, voice communications, order data and an employee profile can provide welcome context towards a better decision, he suggested.
Combining multiple channels of surveillance side by side – particularly trades and communications – is a worthy mission for machine learning technology to ensure it is done properly over time, Fernandez emphasised.
“The goal is to be able to say ‘the system will only raise an alert if it can identify issues in both communications and trading at the same time’, which is a more medium-term objective from a technology perspective,” he said.
What regulators want
Effective market abuse surveillance is what the regulator wants. The means to that end is largely a challenge of data and is very much the company’s business, the bank surveillance manager suggested.
“I know that they’d like innovative ideas, because on the fintech side they run workshops to support exactly that. They want some of the innovation to spill over into the banks,” the source said. “The other side of that coin is to make sure – when selecting a vendor and employing a technology – that it’s appropriate, sustainable and it isn’t some fly-by-night company.”
In the US, the Securities and Exchange Commission has been public about using machine learning algorithms for its own internal tools. Regulators in the US have gone further than most to encourage innovation, publicly stating their desire to see more of it.
Still, like a good school pupil taking a mathematics test, firms would be better off if they consistently explain their workings to authorities. Important decisions – such as why one issue was flagged, whereas another was not – should come with supporting evidence for why decisions were taken.
“Explainability is a key item to highlight,” Fernandez said. “When these technologies are implemented, it’s vital that the financial institutions using them are able to explain clearly how they work, and they will need to have some predictability of how these new technologies work as well.”
Amid industry frustration with false positives, the risk of false negatives is also relevant as the aim of the technology being brought to bear is to reduce the total number of alerts, the bulk of which have historically been false positives.
“Even one false negative could be very costly,” Fernandez said. “It’s a matter of risk tolerance for the compliance department. If you are making a transition towards using machine learning technology or even just enhancements to existing models, it’s important to do careful testing to detect those risks.
Looking at previous cases that have been raised and using them as benchmarks for a new system seems sensible, according to the surveillance manager. “If we’re moving to a new system or a new way of doing something, it’s because we want to make improvements,” he said.
“You can’t make an omelette without breaking a few eggs. I don’t subscribe to the idea that, by moving and improving, we risk losing what we’re already capturing, because that implies things are in a great state at the moment – which they aren’t. Otherwise, the regulator wouldn’t be worried about the calibration of our existing surveillance” he added.
Moving the needle
Whatever the problems of introducing the technology, none of the panel were in any doubt about the need for change. Firms’ frustrations at false positives, regulators’ enthusiasm for innovation and dissatisfaction with existing calibrations, and the opportunities afforded by the revolutionary technology available all point towards the need for change.
“The needle has moved,” said Sachdev. “Looking at the traditional surveillance model and the way the programme has been running in the past, we’re already moving towards more of a holistic approach to surveillance. A joined-up approach is what is needed to address the issues in front of us.”
Technology will make the difference in this strategy, he suggested. “We need to embrace technology and bring it into the fold, which means more budget and some hard dollars being spent on vendor licences, and investment in our own teams within the surveillance spectrum, to ensure they have the right knowledge and skill set to succeed.”
The challenge remains a tough one. “It’s primarily a data challenge with a regulatory edge to it,” the surveillance manager concluded. “It’s vital that the data is clean, and – in the vendors you choose and the direction you decide to take – that you understand the risks prevalent within your business, that you can show them to the regulators when they come calling, and satisfy them with your own risk assessment. That’s it in a nutshell.”
The panellists were speaking in a personal capacity. The views expressed by the panel do not necessarily reflect or represent the views of their respective institutions.
Sponsored content
Copyright Infopro Digital Limited. All rights reserved.
As outlined in our terms and conditions, https://www.infopro-digital.com/terms-and-conditions/subscriptions/ (point 2.4), printing is limited to a single copy.
If you would like to purchase additional rights please email info@risk.net
Copyright Infopro Digital Limited. All rights reserved.
You may share this content using our article tools. As outlined in our terms and conditions, https://www.infopro-digital.com/terms-and-conditions/subscriptions/ (clause 2.4), an Authorised User may only make one copy of the materials for their own personal use. You must also comply with the restrictions in clause 2.5.
If you would like to purchase additional rights please email info@risk.net