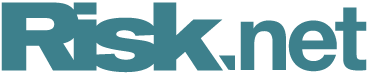
Allocation models that know their unknowns
Quants say probabilistic programming beats machine learning in balancing strategies
Quant firms are buzzing with ideas about algorithms that can ‘learn’ how best to adjust the mix of assets and strategies they trade. But another technology – probabilistic programming – may yet prove equally valuable in answering that question.
The approach allows firms in their portfolio allocation to build models incorporating the kind of market savvy that traders take for granted and also to construct a probabilistic view of future returns, according to Thomas Wiecki, head of research at hedge fund Quantopian. Both are areas where voguish machine learning models now being employed in allocation can struggle.
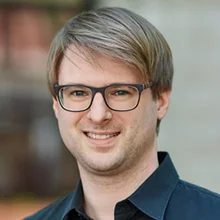
Portfolio allocation decisions hinge on how markets are primed to move and how linkages between strategies or assets are likely to evolve. Allocation calls often shape fund performance more than security-level choices, particularly for a fund such as Quantopian that crowdsources strategies, including from amateur quants.
Quant funds see the area as ripe for the application of new technology because the linkages in question often are complex and non-linear.
Firms have built machine learning allocation models that learn from historical data how assets and strategies might behave in different markets and how relationships between them could change. These models start, though, with no base understanding of how markets work and can latch onto spurious patterns in the data, ascribing them unfounded meaning.
Models that use probabilistic programming, by contrast, start out with rules about how markets function but include an element of uncertainty about how far those rules will hold. The models simulate millions of future paths for a given portfolio within those boundaries.
“Compared to machine learning, probabilistic programming allows you to build a model that is far more targeted and incorporates much more information about the domain of the problem,” says Wiecki. “We might know that the correlations often have a certain structure or that volatility tends to change over time,” he says. “If we can prime the model to say ‘this is the direction to look in’, that is really helpful.”
Probabilistic programming essentially automates Bayesian inference, the statistical technique whereby the probability of a given event is updated step-by-step based on fresh evidence. Only recently have these tools become powerful enough to use in models at the scale needed for quant finance.
Quantopian’s models generate a probability distribution of future daily returns for the dozens of different strategies the hedge fund runs, based on past performance data. “Sometimes, due to luck, even a bad strategy will have a good run and a good strategy will have a bad run. Those would be scenarios we consider in the millions of forecasts that we simulate,” Wiecki says.
The approach allows the firm to find the portfolio balance that works best across all possible future scenarios, rather than just the most likely scenario and lets the firm be more “principled” about the uncertainty in its forecasts.
“A strategy that has a good track record could be good, or it could have got lucky, or it could be overfitted. We can never truly know which,” Wiecki says.
Only users who have a paid subscription or are part of a corporate subscription are able to print or copy content.
To access these options, along with all other subscription benefits, please contact info@risk.net or view our subscription options here: http://subscriptions.risk.net/subscribe
You are currently unable to print this content. Please contact info@risk.net to find out more.
You are currently unable to copy this content. Please contact info@risk.net to find out more.
Copyright Infopro Digital Limited. All rights reserved.
You may share this content using our article tools. Printing this content is for the sole use of the Authorised User (named subscriber), as outlined in our terms and conditions - https://www.infopro-insight.com/terms-conditions/insight-subscriptions/
If you would like to purchase additional rights please email info@risk.net
Copyright Infopro Digital Limited. All rights reserved.
You may share this content using our article tools. Copying this content is for the sole use of the Authorised User (named subscriber), as outlined in our terms and conditions - https://www.infopro-insight.com/terms-conditions/insight-subscriptions/
If you would like to purchase additional rights please email info@risk.net
More on Our take
Are market-makers better at dealing with central bank intervention?
Lack of pain following BoJ intervention suggests dealers are better at handling event risk
Hedge funds must race the clock to check their dealer-rule status
Working out whether a firm is caught by SEC registration requirement could take months
Filling gaps in market data with optimal transport
Julius Baer quant proposes novel way to generate accurate prices for illiquid maturities
Why Europe still awaits a private credit CLO
Tricky questions face managers that plan to launch the structure on the continent
The signs of tacit collusion in the dividend play trade
Game theory and real-world data point to a different understanding of how arbitrage in markets works
Decades of history says you can beat high inflation with quality
Factors such as momentum and value generally outperform the market irrespective of inflation, but new research suggests quality stocks are best when prices are rising rapidly
Esma faces tough task in implementing Emir 3.0
EU regulator must contend with tight timeframes and increasing workload without additional resources
Quants are using language models to map what causes what
GPT-4 does a surprisingly good job of separating causation from correlation
Most read
- Breaking out of the cells: banks’ long goodbye to spreadsheets
- Too soon to say good riddance to banks’ public enemy number one
- Industry calls for major rethink of Basel III rules