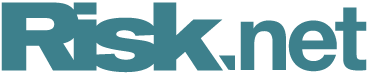
Reducing noise is as important as radical change
Quants study ways to reduce noise in XVA Greeks calculations
Price sensitivities to risk factors, otherwise known as Greeks, have recently been just as challenging to calculate as they are essential in efficient risk management and regulatory reporting.
This is especially true in the case of derivatives with early exercise features, such as Bermudan options, where exercise is allowed at certain specified dates before expiry.
It becomes even more complicated and time-consuming when one has to price valuation adjustments (XVAs) for these instruments, as this involves computation of the future values of the derivative at each exercise point.
Distributions of future values are computed using Monte Carlo simulations and regression. Finding Greeks, on the other hand, requires differentiation of these regressions at each future value, which can be computationally very intensive. It can also produce a lot of noise in the estimates, as the regressions themselves are occasionally noisy.
“To calculate the Greeks of prices or XVAs, we should go through these regressions and differentiate at each future value,” says Alexandre Antonov, a director at Standard Chartered in London. “Regression techniques can be very complicated and painful. Sometimes you would like to avoid the differentiation of regressions.”
In this month’s technical, Pathwise XVA Greeks for early-exercise products, Antonov and his co-authors Serguei Mechkov, a senior vice-president at Numerix in Paris, Michael Konikov, an executive director, Andy McClelland, a director and Serguei Issakov, a senior vice-president within the same firm, show differentiation of regressions can in fact be avoided for some derivatives instruments.
Future values of products with early exercise are typically computed using a so-called backward Monte Carlo, a combination of Monte Carlo simulation and regression, carefully calculating the exercise conditions, and then running a forward Monte Carlo aggregating the final result.
Typically, the Greeks of prices are calculated by differentiating the regression function. Since prices are based on optimal exercise values in the future, differentiation at future exercise dates is not required for derivatives that are typically priced using backward Monte Carlo, because the differentials at these points are zero.
The quants show this conclusion can also be extended for XVAs to a wider class of products. For the calculation of credit valuation adjustment sensitivities of products with single exercise and simple collateral arrangements, such as one-sided credit support annexes, previous works have showed differentiation of regression can be avoided altogether by differentiating cashflows of the derivative instead and applying extra regressions.
If we manage to apply it to only cashflows, it is easy, because quite often we know all the cashflows analytically, so it’s not a big deal. However, the derivative of the regression is complicated
Alexandre Antonov, Standard Chartered
The cashflows could then be combined in such a way that one can arrive at the Greeks.
The authors extend this to any instrument that can be priced using backward Monte Carlo, such as those with callable, multi-callable or combinations of callable and barrier options.
“If we manage to apply it to only cashflows, it is easy, because quite often we know all the cashflows analytically, so it’s not a big deal. However, the derivative of the regression is complicated,” says Antonov.
Numerix has been using the technique as part of its XVA pricing package for almost a year.
In recent years, techniques such as adjoint algorithmic differentiation (AAD) helped substantially speed up sensitivities calculation both for XVAs and regulatory reporting by anywhere between 10–500 times. AAD is already being used for a number of applications, such as risk management of complex derivatives, calculation of market risk sensitivities for regulatory requirements and computation of CVA Greeks.
Antonov says that for Greeks for certain instruments and under specific conditions, the results in their paper also extend to algorithmic techniques such as AAD, making it possible to avoid the inefficient step of differentiating regressions that is otherwise part of the algorithm.
Avoiding differentiation has two advantages – speed and accuracy. Using this new technique, Greeks can be computed almost as quickly as the time it takes to price the derivatives. Cutting the step of differentiating regressions also helps reduce noise in the estimation.
With growing regulatory requirements and the industry’s rapid move towards clearing and electronic trading, optimisation is clearly the key to running a successful business, pushing quants to focus more on issues such as margin compression and price impact optimisation.
It is no surprise, therefore, that some would want to improve efficiency and reduce complexity wherever possible, even for techniques that already work well, such as AAD, for instance.
They may not seem like radical changes, but small improvements over a period of time could save someone a lot of pain in the long run.
Only users who have a paid subscription or are part of a corporate subscription are able to print or copy content.
To access these options, along with all other subscription benefits, please contact info@risk.net or view our subscription options here: http://subscriptions.risk.net/subscribe
You are currently unable to print this content. Please contact info@risk.net to find out more.
You are currently unable to copy this content. Please contact info@risk.net to find out more.
Copyright Infopro Digital Limited. All rights reserved.
You may share this content using our article tools. Printing this content is for the sole use of the Authorised User (named subscriber), as outlined in our terms and conditions - https://www.infopro-insight.com/terms-conditions/insight-subscriptions/
If you would like to purchase additional rights please email info@risk.net
Copyright Infopro Digital Limited. All rights reserved.
You may share this content using our article tools. Copying this content is for the sole use of the Authorised User (named subscriber), as outlined in our terms and conditions - https://www.infopro-insight.com/terms-conditions/insight-subscriptions/
If you would like to purchase additional rights please email info@risk.net
More on Our take
Hedge funds must race the clock to check their dealer-rule status
Working out whether a firm is caught by SEC registration requirement could take months
Filling gaps in market data with optimal transport
Julius Baer quant proposes novel way to generate accurate prices for illiquid maturities
Why Europe still awaits a private credit CLO
Tricky questions face managers that plan to launch the structure on the continent
The signs of tacit collusion in the dividend play trade
Game theory and real-world data point to a different understanding of how arbitrage in markets works
Decades of history says you can beat high inflation with quality
Factors such as momentum and value generally outperform the market irrespective of inflation, but new research suggests quality stocks are best when prices are rising rapidly
Esma faces tough task in implementing Emir 3.0
EU regulator must contend with tight timeframes and increasing workload without additional resources
Quants are using language models to map what causes what
GPT-4 does a surprisingly good job of separating causation from correlation
China stock sell-off will test securities firms’ risk managers
Regulatory measures to support stock market could add to risks facing securities sector