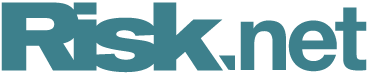
Some quant shops doomed to ‘struggle’ – López de Prado
Theory-first firms must modernise their methods or wither, says machine learning expert

Quant shops that stick too stubbornly to theory when devising strategies will trail behind maths-driven “empiricists” who analyse data with no preconceptions. That’s according to Marcos López de Prado, the former head of machine learning at AQR and founder of a new venture that aims to disrupt the traditional quant asset management business.
“Testing only flawed theories leads to confirmation bias. What I call the maths-quant firms will continue to thrive because of the growing number of alternative datasets, greater computing power, and the development of new analytical methods,” he says.
The “econ-quants”, by contrast – firms that ground their strategies in theories from economists such as factor investing pioneers Eugene Fama and Kenneth French – tend to use research techniques that make them prone to formulating strategies that look good on paper, but are a figment of excessive testing. “Unless there is some sort of revolution in the econ-quant space, those firms will struggle.”
López de Prado left AQR in September, after just a year with the firm, to launch an enterprise that will use machine learning to scope for trading strategies in data. The project’s aims are not unlike what some rivals are attempting. Where López de Prado hopes to gain an edge is by using methods borrowed from outside finance to zero in on statistically significant data and weed out false positives. The firm will then provide trading instructions direct to investors, chopping out the need to outsource trade execution to asset managers.
To aid this data-crunching effort, López de Prado is looking ahead to the arrival of super-fast quantum computers. Whoever harnesses this next-gen technology first will have an advantage over peers, he says.
Leaden factors
But computational prowess is worthless if the underlying strategies are flawed. López de Prado is dismissive about the rationale behind strategies centred on risk factors. Such investment techniques have become big business: BlackRock estimates the factor investing sector will be worth over $3 trillion by 2022. The Cornell University professor believes some factors are built on shaky scientific foundations, though.
“The firms that are founded by economists typically use a quantitative toolkit that mostly comprises econometric analysis or classical statistical methods,” he says. “They form a theory, they select the data that helps them prove that theory, they run some thousands of linear regressions, they report only the result with the lowest p-value.”
P-values measure the statistical significance of an observation. The lower the score, the less likely the findings are simply down to chance.
Frequently such tests, which firms apply to sift meaningful signals from statistical noise, ignore the number of strategies tried and discarded before finding those that work. A quant that backtests a thousand ideas will find bogus strategies with Sharpe ratios as high as three, López de Prado says.
Canadian economist Campbell Harvey has identified that most factor investing strategies fail tests of statistical significance when multiple testing is accounted for. But even Harvey was “generous” in some of his assumptions, López de Prado says. “If you acknowledge that each study is the result of thousands of unreported backtests, rather than one backtest, it is quite unlikely any of these factors is real.”
Crucially, because the strategies in question target low Sharpe ratios, it will take decades or more to prove they are false, he says.
Firms also take as “axioms” ideas that often turn out to be wrong or overly simplistic, López de Prado argues, such as the notion that markets pay a linear premium on risk, or that correlations are effective measures of co-dependence.
Several of the main factors proffered as persistent return drivers – such as buying value stocks – have lagged the market for years (see figure 1). Meanwhile, firms that use mathematics to detect patterns in data without preconceptions, such as Renaissance Technologies, have enjoyed eye-catching returns.
True positives
The name of López de Prado’s new firm – True Positive Technologies – refers to tools he developed to detect false investment strategies, so preventing unnecessary losses for investors, he explains. He sold patent applications for several such algorithms to AQR before leaving the firm.
In the new venture, machine learning will help detect patterns in data and filter out bogus discoveries – in other words, it will be used as a research tool. This is different from how sceptics in the industry talk of the technology as a black box model to run portfolios autonomously. “We can use machine learning algorithms to identify which are the important features involved in a market phenomenon, and how the features interrelate,” López de Prado says.
Such an approach is common for researchers in disciplines like materials science and biomedical research but is less prevalent in finance.
More than 700 papers in the field of chemometrics – the discipline at the intersection of chemistry and statistics – include machine learning terms, for example, compared with fewer than 100 papers on statistics and economics, López de Prado found.
Big asset managers assert that size gives them a competitive edge, enabling them to cross trades and lessen transaction fees for clients in a sector locked in a margin-shredding price war.
But López de Prado aims to undercut the cost-cutters by not taking custody of assets. Instead, the firm will develop strategies through its “strategy factory” that investors can implement themselves, and will advise institutions on building simpler, do-it-yourself strategies in-house.
“Institutional investors don’t need to rely on asset managers any more,” López de Prado says. Instead of paying for managers to maintain operational infrastructure that institutions already have, such as trading desks, “you insert within the institution the component they are missing – the advanced research”, he explains.
For the simplest strategies the fees could be as low as 10 basis points of managed assets with no performance element. For more complex strategies targeting higher Sharpe ratios, the performance fees would be the main source of income.
But what if López de Prado’s machines find markets to be largely efficient? Might the maths-quants find that new data yields little new alpha? López de Prado insists not.

The view of markets as efficient price-discovery machines – an idea that underpins the notion of simple, persistent and unchanging factors – is wrong and getting more so, he argues. “Markets are adaptive. They are not stale. They do not conform to our ideals.”
Regulation has created inefficiencies by stopping players such as investment banks’ proprietary trading desks from arbitraging skewed prices. Passive investing has put trillions of dollars in the hands of vehicles that will buy “anything in an index regardless of valuation, pricing, fundamentals, expectations”.
Information, meanwhile, is becoming less evenly spread among market participants, with the maths-quants able to detect trading opportunities in new data that others cannot see, so he claims. “We have more data and computing power, and better mathematical techniques to find and exploit imperfections that before we were not even aware of,” López de Prado says.
He draws a comparison with mining for precious metals, where chemical extraction means more gold is extracted today than when miners were digging through visible seams.
“There was a period in history when people thought that gold had been essentially depleted. But today, through industrial processes, more can be extracted in a year than in a couple of centuries in the past. In quant investing we are talking about more than just the opportunities that were available yesterday. Markets are more inefficient than ever.”
Quantum dawn
Tech advances such as the deployment of quantum computing will also favour a minority of market players across different fields of investment.
Firms ignoring advances on computing’s front line will find quantum computing is “irrelevant until it becomes the only thing that matters”, López de Prado predicts. At that point “there will be no time to adjust or adapt. There will not be a year. The profits will be obtained by the first with the technology”.
Quantum computers use the ability of sub-atomic particles to exist in multiple states at once to churn through data exponentially faster than a conventional machine with its binary units of memory.
Right now, quantum computers face practical impediments to their day-to-day use. They must be cooled to well below freezing to work, for example. But quants have shown already how the machines can solve complex finance problems such as optimising large portfolios.
A patent is pending on a technology developed by López de Prado and researchers at 1QB Information Technologies that uses quantum computing to optimise portfolios.
“We don’t know when these new machines will be deployed, but when they are, swathes of investing behaviour will be immediately transformed,” López de Prado says.
Liquidity arbitrage traders that triangulate between markets would find there was “all of a sudden” no money to be made, he says. “That’s the kind of opportunity that would collapse at the flip of a switch.”
Asset managers that rebalance portfolios frequently would be able to optimise their trading execution using much faster simulations from machine learning algorithms running on quantum computers.
Buyers and sellers in complex asset classes with abundant data, such as real estate, would benefit from the combination of machine learning techniques and greater computing power.
“People need to prepare,” López de Prado says. “We don’t know how long it could take for these machines to become commercially available, but one thing is certain: once they do, it will take no time for someone to extract most of the profit.”
Editing by Alex Krohn
Only users who have a paid subscription or are part of a corporate subscription are able to print or copy content.
To access these options, along with all other subscription benefits, please contact info@risk.net or view our subscription options here: http://subscriptions.risk.net/subscribe
You are currently unable to print this content. Please contact info@risk.net to find out more.
You are currently unable to copy this content. Please contact info@risk.net to find out more.
Copyright Infopro Digital Limited. All rights reserved.
As outlined in our terms and conditions, https://www.infopro-digital.com/terms-and-conditions/subscriptions/ (point 2.4), printing is limited to a single copy.
If you would like to purchase additional rights please email info@risk.net
Copyright Infopro Digital Limited. All rights reserved.
You may share this content using our article tools. As outlined in our terms and conditions, https://www.infopro-digital.com/terms-and-conditions/subscriptions/ (clause 2.4), an Authorised User may only make one copy of the materials for their own personal use. You must also comply with the restrictions in clause 2.5.
If you would like to purchase additional rights please email info@risk.net
More on Quant investing
Execs can game sentiment engines, but can they fool LLMs?
Quants are firing up large language models to cut through corporate blather
The quants who kicked the hornets’ nest – to champion causality
A small but influential cadre says the multi-trillion-dollar factor investing industry is based on flawed science
‘Brace, brace’: quants say soft landing is unlikely
Investors should prepare for sticky inflation and volatile asset prices as central banks grapple with turning rates cycle
Trend following struggles to return to vogue
Macro outlook for trend appears to be favourable, but 2023’s performance flop gives would-be investors pause for thought
Can machine learning help predict recessions? Not really
Artificial intelligence models stumble on noisy data and lack of interpretability
How patchy liquidity is stymieing systematic credit
…and what investors like AllianceBernstein, Man Numeric and Acadian are doing about it
How Man Numeric found SVB red flags in credit data
Network analysis helps quant shop spot concentration and contagion risks
Is low vol crowded? That depends who you ask
Equity drawdowns have pushed more investors into low volatility strategies, raising fears of a build-up of risk