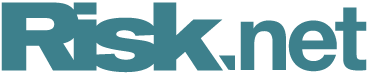
AllianceBernstein digs into its own data, looking for alpha
Firm combs through information about its portfolio managers for signs of bias and bad habits
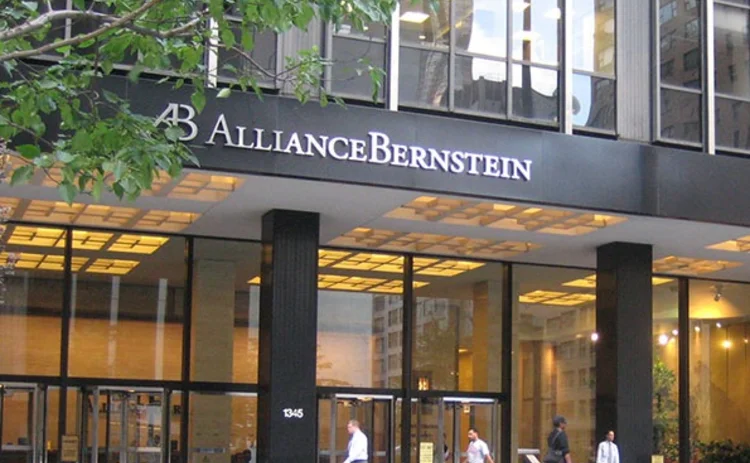
Andrew Chin, head of quantitative research at AllianceBernstein, views its in-house data as “uncharted territory”. Chin, who is also Alliance’s chief risk officer, is looking to scry through so-called alternative data sets beyond the typical market, company and economic fundamentals. And he has a ready hoard of numbers – the firm’s internal and proprietary data – to distil for alpha.
Over the years, AllianceBernstein has gathered data on its portfolio managers’ decisions, from how they trade –
Only users who have a paid subscription or are part of a corporate subscription are able to print or copy content.
To access these options, along with all other subscription benefits, please contact info@risk.net or view our subscription options here: http://subscriptions.risk.net/subscribe
You are currently unable to print this content. Please contact info@risk.net to find out more.
You are currently unable to copy this content. Please contact info@risk.net to find out more.
Copyright Infopro Digital Limited. All rights reserved.
You may share this content using our article tools. Printing this content is for the sole use of the Authorised User (named subscriber), as outlined in our terms and conditions - https://www.infopro-insight.com/terms-conditions/insight-subscriptions/
If you would like to purchase additional rights please email info@risk.net
Copyright Infopro Digital Limited. All rights reserved.
You may share this content using our article tools. Copying this content is for the sole use of the Authorised User (named subscriber), as outlined in our terms and conditions - https://www.infopro-insight.com/terms-conditions/insight-subscriptions/
If you would like to purchase additional rights please email info@risk.net
More on Asset management
Execs can game sentiment engines, but can they fool LLMs?
Quants are firing up large language models to cut through corporate blather
Pension schemes prep facilities to ‘repo’ fund units
Schroders, State Street and Cardano plan new way to shore up pension portfolios against repeat of 2022 gilt crisis
Fears of runaway risk on offshore reinsurance
Life insurers catch the eye of UK regulator for pension buyout financing trick
Hot topic: SEC climate disclosure rule divides industry
Proposal likely to flounder on First Amendment concerns, lawyers believe
‘Brace, brace’: quants say soft landing is unlikely
Investors should prepare for sticky inflation and volatile asset prices as central banks grapple with turning rates cycle
Trend following struggles to return to vogue
Macro outlook for trend appears to be favourable, but 2023’s performance flop gives would-be investors pause for thought
Start-up bond platform OpenYield prepares to launch
Start-up aims to give retail brokers the same electronic liquidity used by the professionals
Can machine learning help predict recessions? Not really
Artificial intelligence models stumble on noisy data and lack of interpretability
Most read
- Breaking out of the cells: banks’ long goodbye to spreadsheets
- Too soon to say good riddance to banks’ public enemy number one
- Industry calls for major rethink of Basel III rules