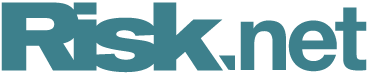
This article was paid for by a contributing third party.More Information.
Moonshots and machines: can AI solve the problems of fincrime?
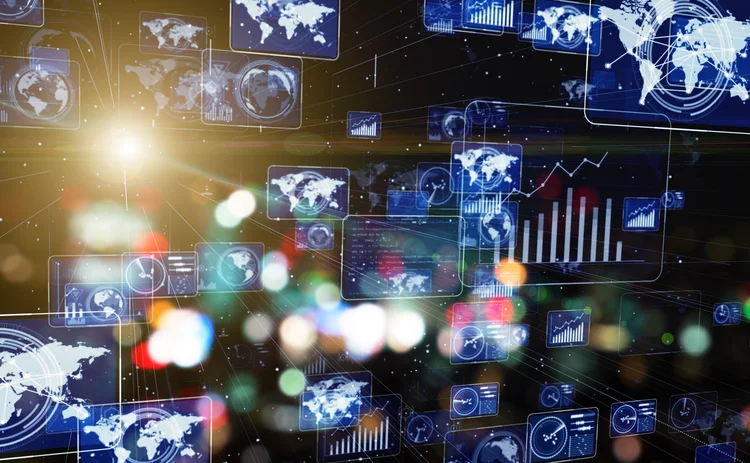
New technologies such as artificial intelligence (AI) and machine learning promise much in the battle against financial crime, but where are these solutions best deployed? A panel of anti-money laundering and analytics professionals convened for a Risk.net webinar in association with NICE Actimize to discuss the targeted use of AI in fighting financial crime
The Panel
- Achi Hackmon, Head of Artificial Intelligence and Analytics, NICE Actimize
- Jayati Chaudhury, Global Investment Banking Lead for Anti-Money Laundering Transaction Monitoring, Barclays
- Patrick Dutton, Regional Head, Intelligence and Analytics, HSBC
- Moderator: Steve Marlin, Staff writer, Risk Management, Risk.net
The fight against financial crime brings many challenges for banking and financial institutions, as well as the businesses they serve. But it also affords the financial sector a chance to deploy artificial intelligence (AI) and machine learning solutions to assist with compliance. As financial criminals grow more sophisticated, the entities they seek to victimise must keep up with ever-changing modes of crime to protect themselves. AI and machine learning may offer a solution, but only one-third of webinar attendees polled said they were using AI for anti-money laundering (AML), Bank Secrecy Act and sanctions compilance.
The learning curve is steep as people learn how to use AI and machine learning, said Jayati Chaudhury, global investment banking lead for AML transaction monitoring at Barclays. “It looks good on the résumé, but that is not the reason firms will invest in it,” she said.
Her point is pertinent in that there must be a clear benefit to the use of such systems to justify the capital expenditures needed to acquire them. The session discussed how and where AI and machine learning solutions are best directed.
AI for surveillance and mapping relational networks
Client surveillance is arguably an obvious starting point when it comes to deploying AI in the financial world, not least because of the volume of data and the difficulties of defining standards of normal behaviour. For example, said Chaudhury, in investment banking the anticipation of how customers will behave will not necessarily match how they actually behave. “Detecting patterns of behaviour over time and defining ‘normal’, keeping your current business lines and your risk in mind, is a challenge,” she said.
At such times, there is only so much firms can do through process-oriented controls such as risk assessment, which most reputable institutions perform at least annually to gauge their readiness for market risks and emerging threats.
“A lot of the time, customers intending to misuse financial systems are flying under the radar,” Chaudhury said.
A single transaction may not look suspicious, the panel concurred. In and of itself, a trade may look perfectly legitimate. Yet, when one takes a holistic view of a customer’s behaviour – placing them at a centre of an investigational nexus – and examines all their connections and the payments they have made through an institution, interesting circumstantial evidence of malfeasance may arise.
“Normal databases are not enough,” Chaudhury said. “Customer behaviour may [appear] normal if you look at it in singularity but, if you put it together and try to detect networks [and] … multiple activities over a period of time, that may change the story, and that is what is important to present to regulators.”
“It does not have to be all through the brokerage account. The customer might have been onboarded as a corporate client [and] … they may hold a corporate and brokerage account with your firm, and may also be engaging your firm in correspondent banking activities. At the end of the day, the firm is still liable because it has not been able to detect that kind of suspicious behaviour,” she said.
Such a holistic view, keeping clientele as the focus, is key to ferreting out network linkages, Chaudhury said. “It is becoming much more pertinent in investment banking and in other areas where defining normal is very difficult.”
False alerts
False alerts have long been the bane of AML professionals. The panel recommended that, in conjunction with rules-based processes, it would be a boon if institutions could apply machine learning to learn from patterns of behaviour and detect what is not normal, but “not just because of value and volume, because that tends to create more false positives and is not necessarily the best use of [internal] investigators’ time”, Chaudhury said.
Similarly, Patrick Dutton, HSBC’s regional head of intelligence and analytics in the US, observed that AI can solve all or no problems “if you have not figured out that purpose at the outset”.
“Are we trying to solve a problem of efficiency? Our current model has a lot of [false] alerts. We can use investigator output and machine learning efficiently and effectively to reduce false positives, so humans do not have to look at those as much. That’s today’s problem,” he said.
Dutton stresses that the real potential of such technologies, yet to be meaningfully tapped, lies in information of what may yet occur. “Tomorrow’s problem might be how do I use AI to find things I was not finding before – for more effective financial crime risk management.”
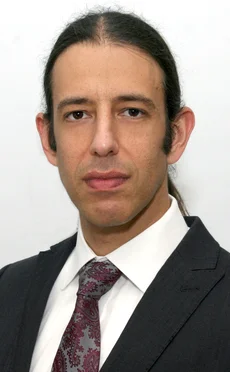
Another way to lower false positives could be to use new solutions to analyse old data, said Achi Hackmon, NICE Actimize’s head of AI and analytics.
“If you had a problem you solved previously with rules or even with the ‘scorecard model’, and you use the same data … and you apply a machine learning algorithm on top, typically we are able to cut the number of alerts by at least half, while maintaining or exceeding the same detection levels. So you can find all the same cases, but be more accurate about it by leveraging machine learning algorithms, even with existing data,” he said.
The use of AI and machine learning is key to handling the vast troves of data that are part of contemporary compliance. A key conclusion of the panel was that, if insights could be gleaned from data and, therefore, behavioural patterns and relationship networks could be detected, that would greatly aid the fight against financial crime.
“For financial crime – in particular, in areas of surveillance that are very tech-enabled – … [AI and machine learning solutions] are the right fit. The size and scale of the problems we are trying to solve need to be taken into account. Surveillance is a very pertinent topic where you can try to use these types of solutions for pattern recognition,” Chaudhury said.
Yet, she adds, even with the best systems, there is no way to successfully detect crime 100% of the time – nor is that what regulators expect of institutions’ transaction monitoring platforms.
The regulatory requirement, she said, is whether an organisation has a “reasonably robust system that can pick up deviations in patterns” from what is normal. It is up to institutions to define what normal is, given their client base.
“Regulators have given us quite a bit of leeway in defining what normal is [based on] our own risk appetite and our own risk assessment. So, if you have done that, these types of solutions can be leveraged to bring out those insights in the data – especially [for] banks of our size where the volume of data is quite huge,” Chaudhury said.
She acknowledges, however, there are always “inherent data problems” that can complicate matters but, overall, AI has been quite helpful.
AI can therefore offer insight into data by teasing out trends and patterns of interaction that might offer tell-tale signs of the typologies and methods of crime occurring at an institution. Notwithstanding technological advances, modern AML, know-your-customer, counterterrorist financing and sanctions compliance is still a risk-based proposition for the financial world: different individuals, families, nationalities and professions get a different risk weighting and score based on an institution’s overall risk matrix.
To that end, clustering and segmentation is a very powerful enabler. Good segmentation can be more accurately achieved using machine learning technology for financial crime detection, according to Hackmon. The process looks at a population of, for example, people, accounts and transactions, and letting the system discern what all the clusters and segments within the data might suggest.
“You do not need to tell [the algorithm] anything, except maybe the interesting features within the data by which to cluster. It does its work in an unsupervised fashion,” he said.
And with a nod towards machine learning, Hackmon adds: “The distinction is whether you tell the system what the solution is or whether you let it find it by itself. It really depends on the type of problem you are trying to solve.”
One of the poll questions posed pertained to unstructured data, which HSBC’s Dutton pointed out was a common complaint in compliance circles – notwithstanding the need to bring more data into the system even, at times, from the public domain.
While unstructured data is a challenge, it may be a place where machine learning and AI techniques “such as natural language processing [NLP] can come into play by taking unstructured data and ‘pulling the structure’ out of it using [tech] and then leveraging other models to create an actionable result or decision,” Hackmon adds.
But, he stressed it was important to decide upfront whether a problem is fit for machine learning. “It needs to be important. Is it a meaningful problem? You need good data and domain expertise to start you off in the right direction.”
One of the best uses of AI is against data-rich and weak signal problems and, according to Dutton, financial crime certainly qualifies. While noting that many different applications exist, his bank has found uses for NLP.
“[NLP] can be used sometimes to identify some interesting things within your regulatory suspicious activity reports filings here in the US and suspicious transaction reports in other places. There can be some hidden things in there that NLP can come out with,” he said.
Similarly, with concerns about trade-based money laundering in the trade finance space and its text-heavy and excessive documentation requirements: “NLP can be very useful in helping to ascertain irregularities or trends that you are looking for,” said Dutton.
He adds that HSBC also used robotic process automation to help with the explainability of its AI models: “The machine is going to write its reasoning and how it came to a decision, in a way that an investigator, auditor, regulator, whoever, can see what the model is doing and how it came to the conclusion it came to.”
Another innovation in which NICE Actimize is investing is a concept called “drift detect” – the detection of the smallest behavioural changes in real time. Hackmon indicated that, as compliance teams build their models around “particular features and aspects of data, determining how behaviour has changed early in the game is super important … Sometimes reality changes in small ways and you need to pick up on that early on.”
He added that machine learning was an ongoing and evolving process, and that firms should not adopt a ‘one-and-done’, silver bullet approach to seek out an ideal algorithm to last them for all time because regulatory requirements keep changing. Accordingly, algorithms and models need to be diligently and regularly managed over time to keep abreast of changing typologies and methods of financial crime.
“Machine learning is not a moonshot,” Hackmon said. “It is not something you do once. You have to keep thinking of how to streamline your processes, how you monitor behaviours through time, and how your system continues improving.”
Watch the full webinar, Solving the right problems with AI
The panellists were speaking in a personal capacity. The views expressed by the panel do not necessarily reflect or represent the views of their respective institutions.
Sponsored content
Copyright Infopro Digital Limited. All rights reserved.
You may share this content using our article tools. Printing this content is for the sole use of the Authorised User (named subscriber), as outlined in our terms and conditions - https://www.infopro-insight.com/terms-conditions/insight-subscriptions/
If you would like to purchase additional rights please email info@risk.net
Copyright Infopro Digital Limited. All rights reserved.
You may share this content using our article tools. Copying this content is for the sole use of the Authorised User (named subscriber), as outlined in our terms and conditions - https://www.infopro-insight.com/terms-conditions/insight-subscriptions/
If you would like to purchase additional rights please email info@risk.net