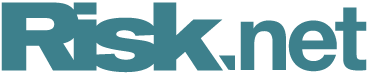
This article was paid for by a contributing third party.More Information.
Machine learning in fraud analytics to combat financial crime – Getting it right
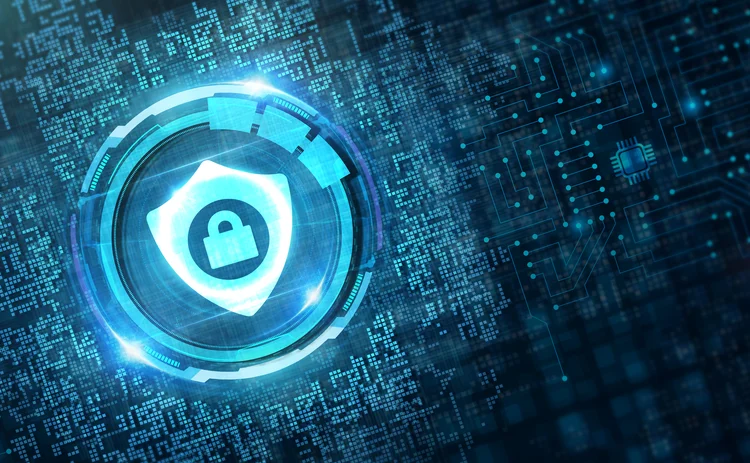
Risk and compliance professionals gathered for a Risk.net webinar in association with NICE Actimize to consider the challenges and opportunities of successfully harnessing artificial intelligence (AI) in the fight against financial criminals
For every dollar lost through fraud, financial services companies lose a few more dollars on the associated expenses, from time, effort and investigations, to fines and legal fees.
Technology offers the possibility of enormous efficiency savings in banks’ efforts to fight fraudsters, but investing in machine learning technology can seem a dizzying prospect for risk and compliance professionals balancing limited budgets and competing pressures.
For firms that have already implemented machine learning models to spot fraudulent transactions among payments traffic, 2020’s coronavirus (Covid‑19) pandemic has provided criminals with a fresh avenue of attack, preying on public fear with a new wave of scams.
“The speed at which criminals move to exploit a situation is astonishing,” said Damian Matich, global head of fraud analytics at NICE Actimize. “There’s any number of scams already working in the market for fraudsters looking to exploit the coronavirus situation.”
Financial institutions are on the front lines of detecting and preventing such fraudulent transactions. Fortunately, they have a variety of machine learning-based techniques at their disposal for analysing fraud patterns and combating organised fraud rings.
“These are unchartered waters, but it’s good that most of the models will be tested, ensuring they are working properly and identifying potential misconduct and fraud,” said a senior operational risk manager at a large international bank.
Lessons learned
Companies face challenges in building anti-fraud models as machine learning analytics becomes more sophisticated. The panellists on the NICE Actimize webinar hosted by Risk.net in March shared the lessons of their own experience of the challenges associated with building machine learning-based models and putting them into action.
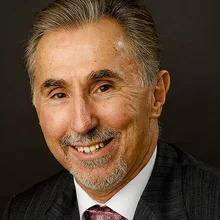
Speed is a leading concern. “You need a rapid time to deployment, because fraud changes so rapidly,” said Matich. “Building what we call a supervised machine learning model requires lots of data – clean data, validated data – together with the fraud tags associated with that particular data tranche.
“The key issue is to build and aggregate the data and correlate it with the fraud tags, and to build and deploy an effective model in a short space of time that is relevant to the initial fraud typology.”
The need to ensure new models do not have AI data bias built into them was highlighted as a cause for concern by Andrew Fleming, global compliance MI, senior risk reporting manager, at HSBC.
“When you’re building your model, you must develop a fair model – one that is actually using data in the fairest way towards your customers,” Fleming said.
“It’s not just about being mindful of the changing threat landscape, but also the impact of the changes you make, which impact individual customers. Should those changes be challenged, we need to be able to explain those changes to the regulator or customer. If we can’t do that, we’re going to be fined for any unintended data bias that unfairly impacts our customers,” he warned.
The technology is maturing fast, with plenty of options for tools on offer to develop machine learning models. However, while accuracy is critical, states Fleming, the personalisation of customer data is the first step to success.
“Customer data models or the dataset that you’re looking to utilise are only as good as the data you’re feeding into them,” Fleming said. “So, the first key step in all this is to think about personalisation of your customers’ data and understanding who your customers actually are. This helps us clarify their risk to the business, their value to us and helps us understand their needs better. Accuracy plays a critical role in this, but accuracy based on an incomplete understanding of the customer will provide limited value.”
Taking an inventory of legacy systems with access points into the new model is another vital early task, according to Fleming, which should include screening out the old tech that won’t work and would hamstring the new system by its obsolescence. “If you bring in a wonderful system and try to overlay it into legacy systems that, frankly, aren’t up to task anymore, it’s not going to succeed,” Fleming added.
In many cases, the tech is newer than the data being fed into it. The data may not have kept pace with the technology over time, instead showing its age and lacking the right level of quality, granularity or standards.
“If you don’t have validation protocols in place and normalised data all the way from inception, then it becomes difficult to subsequently ingest that data and to rely on it to the level of certainty that you want to today,” said Stan Yakoff, adjunct professor teaching market structure regulation, trading and risk management at Fordham University School of Law.
“It’s like raising kids – you want to start instilling good behaviour early on because, by the time they’re 18, it becomes much harder to change their habits. The same is true of data quality – the earlier you’re able to build this governance and oversight in and focus on the data, the better your output will be,” said Yakoff.
For fraud risk professionals, a familiar problem was cited by the senior op risk manager: false positives. These false alarms represent a significant drain on investigators’ time and resources.
“Sometimes you introduce AI and it creates false positives,” he said. “Within those false positives, you can potentially miss something. It’s important to know what you’re putting into a model and review the output before you put it into production.”
Striking a balance
The validation of any new model entails striking a balance between thoroughness and timely deployment. Different teams are involved, many of which have operational jobs on the frontline as well as tinkering with any new roll-out. Mechanisms must be created whereby risk modelling teams can quickly validate models without comprising their independence.
“It’s a fine line,” the senior op risk manager admitted, “you have to work closely with the risk management teams and keep testing different scenarios before it goes into production, ensuring you have the right individuals involved to participate in the creation of these models and ensure proper testing and sign-off.
A balance must be struck between the need for independence for developing new machine learning models, and managing tensions between the model risk management teams and those on the front line of the business that will be using models day to day.
“There should always be tension, and that’s a good thing,” Fleming said, “because, if you get to the situation in which we’re too comfortable with each other, things get missed. We want to have that challenge in the process. We want to have people checking whether what’s been produced is correct and providing value.”
A risk with previous models, he observed, is that without being checked they can run until a regulator spots something amiss, with potentially greater consequences – from fines and censure to losses of reputation or business.
A somewhat dissenting view was offered by Matich, working on the model production side. He suggested that, in some companies, the balance has shifted towards overzealous controls rather than the frontline aim of stopping crime. This has created some avoidable delays, he noted.
“I’ve seen too many examples in which the validation process has swung towards becoming overly bureaucratic, and it has lost some of its relevance to the goal we’re trying to achieve, which is to stop criminal behaviour,” he warned.
“In my view, we need to have dialogue at an early stage between the model developers, the business and the validation teams, get around the table and work out the requirements of each party involved and the mechanisms for putting processes in place. This would ensure the validation teams are more aware of what’s going on throughout the build, rather than only when models are ready for production,” he explained.
Validation is not just a pre-deployment exercise – particularly for advanced machine learning. Revalidation of those models is required on a more frequent basis, stressed a compliance director at a large international bank.
“Machine learning is not as much rules-based as it is based on how data anomalies and patterns are being detected,” the compliance director said. “Those data anomalies and patterns could change as your client base and your counterparties change, or as your own employee turnover brings nuances into the dataset. I know having a rigorous revalidation schedule is going to be time- and resource-consuming, but it’s definitely something that needs to be accommodated.”
Research continues on using AI to assist in validating machine learning-based models. Fleming said it is possible, but remains subject to difficulties, not least the challenges of explaining to regulators’ satisfaction how the AI validated the model.
“The better the machine you make, involving machine learning or the more advanced deep-learning processes, the more likely you will end up with a black-box scenario,” he said. “It’s running through the systems and providing a ‘yes or no’ outcome, but the problem is you’re unable to explain why it’s coming to that yes or no decision, and that is a critical area of concern.”
Explainability
There is an odd paradox that the more advanced the machine learning model, the more opaque it becomes. A risk, for a validation process or if a regulator poses questions, is that as with a student using a calculator to do their mathematics homework – it is no longer possible to ‘show your working out’ to explain why the answers given by the model are the right ones.
Fleming argued that regulators in the US and Europe, acting on new laws – such as the General Data Protection Regulation – and concerned with fairness issues such as gender bias, will demand detail from AI models that banks may struggle to provide.
“The ‘get out of jail free’ card for explainability used to be to say: ‘Here’s my algorithm and here are the databases we utilised to extract the data on which we made the relevant decision’. But now we’re getting to the point where explainable is no longer enough – it has to be understandable. For example, can you explain to the regulator a decision on a customer impacted by that decision, and why it was made, in very clear and simple terms? And why it was a fair decision,” he said.
“We’re getting machines that are more complex that are able to do far more than the average human can do in a much shorter space of time. We can’t allow those decision processes to fall into a black box. When the machine makes a decision, it will have to explain why, and do so in simple terms,” Fleming said.
The specific variables used should have been carefully selected, Yakoff underlined: “Data-dependent systems and processes should be tested by asking questions such as: What variables and configurations are you inputting or relying on; which data types are being brought in; what is the likelihood that data can change and who is authorised to change it; what do you expect the output to look like; and what operations or protocols do you have in place when the output is not matching your original expectations?”
Historical data, models and ways of doing things also provide useful contextual analysis. “The more traceability and transparency you have for this information – in essence, the data provenance – the easier the conversation will be with the regulator, senior management, your colleagues, and even with your kids when you explain what it is you do for a living,” Yakoff added.
Fleming disagreed, citing examples of unintended algorithm bias – for example, assigning a higher credit rating based on gender and biometrics resulting in unfair bias, failing facial recognition for some ethnicities, or speech recognition software that works better for men than women. Even the biggest tech giants in the world have got it badly wrong and have been penalised accordingly by regulators.
“These are all current examples of unintended bias in algorithms designed by clever people such as data scientists and analysts. Unfortunately, data can be viewed through a lens that has a particular viewpoint, which – while not intended or wanted – sometimes has an unconscious bias that becomes built into it,” said Fleming.
“This is one of the reasons we have to be careful with our algorithms, and how often we check the data and validate the models – to ensure they are accurate and that no sections of the customer base are being discriminated against,” he said.
The compliance director provided the legal risk perspective to the challenge, beginning by classifying the purpose and types of models being used. “We need to start with the classification of what sort of machine learning models we are using to be able to avoid getting into legal problems, and to be better able to explain what we’re doing and why we’re doing it,” he said.
Checking the checker applies to validation and revalidation, he argued, emphasising scrutiny, strong governance and including audit trails to verify it. “Whoever is validating the answers within the teams should be an independent party from those conducting the user acceptance testing,” he added.
Intelligence sharing
Financial criminals have grown more sophisticated in their own use of technology, and are known to go from bank to bank to find and exploit a weak link. Data and technology are also affording new opportunities to share intelligence among the banking community, providing more information and a greater line of sight on suspected fraudsters and suspicious transactions.
In the UK, Matich pointed to VocaLink Mastercard as an example of collaboration for intelligence sharing that can inform a decision about a fraudulent transaction in real time. Banks are supplying account data on money mules – people moving stolen money – to the Mastercard service, which can see the financial transactions that flow through the UK financial network. Keeping data protection regulations in mind, such information can be sent encrypted to the investigators that need it.
“They’re able to successfully run a network analysis and determine the flow of funds from that mule, effectively into the criminal repository. That’s a great example of how feeding key pieces of data into the central network allows us to better trace outcomes,” Matich said.
Banks in the US could do more to pool information held by individual institutions about fraud, the senior op risk manager admitted, to more quickly pick up on criminal trends, and learn from each other’s successes or failures.
“It would be helpful to hear what’s going on in a more open forum, to prevent fraud from occurring as opposed to learning about it after the fact,” Matich said. “I think it would be helpful to contribute ideas and recommendations that firms can take away and perform their own analysis, then come back and compare notes.”
Different countries have different approaches. The US Department of Justice, Fleming noted, publishes online updates on the various types of fraud being practised on the public, from fake Covid‑19 cures to phishing for account details by pretending to offer government financial support.
In the UK, police units such as the UK’s Dedicated Card and Payment Crime Unit, funded by industry, represent positive steps towards pooling information, noted Fleming, who used to work in law enforcement. However, he thinks authorities could be doing much more to share crucial information in combating the fraudsters.
“There are lots of different ways that law enforcement is actively engaging with the business community, but isn’t nearly enough – particularly in the use of technology,” Fleming said. “Law enforcement needs to be more proactive in sharing data – not just with banks or insurance companies – but with all businesses to tell them when there’s a crime commonly occurring,” he added.
Watch the full webinar, Machine learning in fraud analytics – How to get it right
The panellists were speaking in a personal capacity. The views expressed by the panel do not necessarily reflect or represent the views of their respective institutions.
Sponsored content
Copyright Infopro Digital Limited. All rights reserved.
You may share this content using our article tools. Printing this content is for the sole use of the Authorised User (named subscriber), as outlined in our terms and conditions - https://www.infopro-insight.com/terms-conditions/insight-subscriptions/
If you would like to purchase additional rights please email info@risk.net
Copyright Infopro Digital Limited. All rights reserved.
You may share this content using our article tools. Copying this content is for the sole use of the Authorised User (named subscriber), as outlined in our terms and conditions - https://www.infopro-insight.com/terms-conditions/insight-subscriptions/
If you would like to purchase additional rights please email info@risk.net