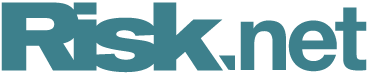
Theory vs Reality
Four of the industry's top op risk executives debated operational risk modelling and capital calculation at a recent half-day briefing discussion in London, moderated by Ellen Davis and sponsored by technology solutions firm Indra
Ellen Davis: What do you see to be the recent challenges and thoughts in the operational risk modelling and capital calculation arena?
Jordi Garcia (BBVA): When I started working on operational risk, everyone had a solution for capital calculation, but only in theory. A lot of mathematicians were preparing a lot of methodologies and formulas, and so on, to make these calculations available for the banks. The challenge comes when we have got real data in the databases, which is what we have in my group today, and especially for the banks who have decided not to apply thresholds, which are normally set at @10,000 - the standard one provided by Basel. We are collecting data from zero thresholds. There is a real challenge when you use real data and you try to model it to the different possibilities in the market. Another challenge is to identify the different behaviours of the different lines of business and the different typologies of risk in your own company. Diversification is also an issue that we are addressing. Another big challenge for us is how to mix the internal data with external data; this is something that is still a big problem because I don't think anybody has a solution for that at the moment. There are some possibilities that we may apply in the future. To me, those are the challenges.
Mercedes Marhuenda (Indra): Operational risk is a very young discipline in comparison to market and credit risk modelling. In fact, it shows that the op risk methods and tools to calculate operational risk capital are going to change in the coming years with the empirical evidence, the knowledge of operational risk teams and the feedback from researchers, regulators and academics. More exactly, under my point of view the main challenges in this area are in the input data field (the completeness and accuracy of the internal data and the process of mix them with external data) and in the modelling matter (the approach to use, the fat tails and the impact of high thresholds).
Jonathan Howitt (Man Group): We don't have a lot of internal data, so we consciously went for more of a scenario-driven approach. We said 'this is a qualitative exercise - it's not going to be exact from the outset - we're going to want to use this with management and drive out lessons for mitigation and so on'. So we never took an exact approach to this. We said we would pursue a qualitative lessons-learned approach.
What are our challenges? We have incorporated effects of insurance, which is quite telling because when you look at the amount it takes of your capital you say, 'well, are we getting a reasonable value for money out of this premium?' We do haggle out the business. There's a certain negotiation factor as to what the frequency and severity parameters should be when you take a purely qualitative, forward-looking approach. You say 'well, what's your 95% worst-case loss for this kind of problem, like a misselling, a misevaluation or a disaster recovery-type problem? But that's the fun of it. It's not just done in a vacuum; it's done with the management of the business and we will show them 'this is what insurance gets you; your investment in IT, your investment in disaster recovery or your compliance agenda to train sales people as to how they should sell - this is how we think it should reduce your risk'. We try and take a forward-looking view. We are consciously qualitative. I think there are challenges around that because, of course, it's imprecise, but we're honest about that. We don't try to allocate down to the enth level of business because it's actually not productive for a 'desk head' to worry about his op risk capital. We'd rather use Key Risk Indicators (KRIs), internal loss and triggers, if you like, at that level.
Santiago Carrillo (RiskLab): We are at the beginning of the process and I think we are starting to understand what operational risk really is. If I have learned something it is that we shouldn't be dogmatic. I will give some opinions - not the truth, not even my truth. I would like to talk about two ideas related to the challenges that Ellen has asked us to speak about. The first one has a lot to do with what Jordi has just spoken about. Some time ago, the idea was that in reality we only need to have high-threshold data and to apply Poisson and Pareto distributions for modelling the frequency and severity, and everything would be okay. From a mathematical point of view, there is a strong foundation for this. However, we have some proof in the data that, in many situations, it is not true: we are not in the asymptotical regime. The Pareto-Poisson solution does not seem to be the definitive solution to model and compute economic or regulatory capital. I think we need more data. We need a picture of the global severity distribution for risk management and event modelling.
The second one is about what can we do when we don't have enough data. From a regulatory point of view, you have a standard approach or the basic approach, but that's not the problem I'm interested in. The problem is, I can calculate my capital in the standard approach, but I need to understand my risk profile better and what are the real risks to my bank. But I have a small amount of data so how can I implement a consistent scenario analysis? How do I use a small amount of data when I can have external data with scenario generation? What is the logic of the scenario you must implement in your bank/in your entity in order to produce information and extract an intelligent understanding of your real situation? I think it is one of the big challenges for operational risk managers at the moment.
Ellen Davis: Correlations have become a very hot topic with the regulators and firms. Firms want to generally be able to correlate and regulators are a bit more cautious about correlation. Will correlations necessarily result in a capital reduction and how does the panel see the evolution of correlation modelling over the next 12 to 18 months?
Santiago Carrillo: Maybe the first thing we must say when talking about correlation is that in finance, we used to think in normal terms, in Gaussian terms, where correlations describe completely the dependence structure. In operational risk the distributions are not normal so we can have some surprises with the correlations. For example, it's very easy to produce theoretical situations in which the operational Value at Risk (VaR) of two cells under Basel II is bigger than the sum of the operational VaR of each cell. The VaR is not a coherent measure of risk. Paul Embrecht was the first, I think, to show this problem (the supra additivity of OpVaR) in a recent paper. I think we must be very careful in the study and use of correlations in operational risk in the future because there can be surprises with real data in practice.
Jordi Garcia: In my bank, BBVA, we are leaning to the same conclusions as Santiago because in some cases we have seen that the addition of one, two or more cells leads to less capital than the sum of each one, which is, in theory, the opposite of what one is expecting. However, in other cases, we have seen absolutely the opposite. In certain cases, the usage of diversification may lead to the capital being one half...if you consider the bank as a whole instead of being part of different lines of business. The situation is that there is nothing we can conclude at the moment. We agree absolutely with what Santiago is saying from an empirical point of view.
Ellen Davis: How does the panel think external data can best be used to help model operational risk and deliver the most value for firms in terms of modelling in a practical as well as theoretical sense?
Santiago Carrillo: I think that internal data will not, for many years, be enough to have a complete idea of what could happen in a bank. You need to complete the data with external data. It seems that the best way to use the data is for fitting the severity distribution. You must use both, internal and external data, for a good fitting of severity distribution.
Jordi Garcia: This issue with the external data, for me, is quality. I think one of the issues in operational risk is to have quality data, not only external, but also internal. Before entering into the calculation you should make sure that it is complete and that the classification of your data is also correct. When you decide to use an external database, you should also ask the same questions - is the database complete, has the data quality assurance been determined; is it performing okay or not? This is very important, otherwise, irrespective of the size of the event, if the data quality - let's say the classification of the event - is not good, you may get incorrect calculations when you apply the mathematical model.
Mercedes Marhuenda: The methods used to mix external and internal data should not overestimate the tail of the distribution of severity. For this reason, it is important to scale the external data to the profile of the bank and not to change the risk exposure of financial institutions.
Jonathan Howitt: I have looked at these databases where there is a mechanical way of including your external data in your model. Frankly, I'm not a believer. For the retail model it is perhaps better. We have very few peer-group companies where there is sufficient public data. We do subscribe to a database of 6000-odd media-style write-ups of losses. Sad to say, there is no shortcut but to read them. We filter them for, at least, what is industry relevant and whether we instinctively think it's going to be relevant, and then we come to a top 100 list for our hedge fund side and our brokerage side. We read them very carefully and pick maybe the top 20 that we think people really ought to read. We will then refer them across within our scenarios and if we observe a big external loss - I say this brutally, but economic capital is down to the divisions - we will change the severity, or we will recommend a change to the severity parameters of certain types of loss based on a new external data event. I guess the problem rests with the op risk department to read all this stuff, to be intelligent enough about the business, and throw the stuff out to the various areas within the business and say 'have you thought about this; could it happen here?' Often, and for their own purposes, they'll say 'it couldn't possibly happen here - not us, no never', but you persist. Basically, it's a trigger to review a severity parameter.
The obvious one for us was watching Refco go bust. It was the major competitor for our brokerage division and within a week it's gone. I'm glad to say we picked up its entrails. I think the fact that something as severe as that could happen so quickly in a business so similar to theirs was quite an eye opener for a lot of the senior executives in our brokerage division. A message was certainly learned.
Audience question: Firms have high-frequency low-impact events - settlement failures and that type of thing. At the other end of the scale, they have aircraft crashing into their buildings. This is a very difficult thing to model. However, Basel requires you to model high-frequency low-impact events into your capital calculation. In addition, there is a third category of potential operational risk, which is the risk that firms haven't even thought about; the event that's never happened before. None of us has a crystal ball. Technology, markets and products are evolving and there are risks that will impact your institutions in the future that have not impacted them in the past, or indeed any institution. How are you putting this into an economic capital calculation?
Santiago Carrillo: I think I must divide my answer into two parts because there are two questions in your question. The first one has to do with fat tails. I have an opinion but I am not sure if it is totally Basel II compliant. Effectively, Basel II says you must take fat tails into account; that is one of the reasons why Pareto distribution is successful. If you try to model through Pareto distribution, you will have at least two sources of uncertainty - the first one is that Pareto distributions are very sensitive to new data. For example, some months ago we used Pareto distributions to model the high-impact low-frequency events that we deduced from the last loss data collection from Basel II. We used this data to estimate the number of events in the business line. Then we used a shape parameter of 0.6 and 1.1. If you look at the literature you will see that few banks used these kinds of shape parameters. We have taken the Basel II data as representative of a major European bank and have produced Pareto distribution quarterly data. We try to estimate the shape parameter each quarter with that data. Even after five years, the fluctuation of economic capital was very big - even more than 100% - in many of the trajectories we tried to model. The modelling of fat tails through Pareto distributions often leads to very unstable estimations and tends to overestimate economic capital.
In some cases it is a really big overestimation - in the order of your country's GNP. It's something we need to think about more than we have done to date. In addition, if you use Pareto distributions for computation, the economic capital you will calculate is very sensitive to the proportion of data you have above or below the threshold. A small variation in this proportion produces a big change in the capital. The shape is not so important, but the proportion you have is really important. The problem has not yet been solved and the solution will probably demand you to model your severity distribution piece by piece; to have one kind of distribution for the central part - for the high-frequency low-impact data - and maybe a second distribution for the low-frequency high-impact data in order to be able to produce Monte Carlo simulations and so on. We are working in this direction and hope that it will be one of the solutions.
The other part of your question was how to take into account something that has never occurred before. It's much more complicated and has a lot to do with scenario simulation. With the software tool you can produce a stress analysis that takes into account internal and external data and something we call 'stress data'. You can produce data that corresponds to this new kind of situation, but you need expert opinion, scenario analysis and all the kinds of information Jordi just mentioned, in order to produce possible losses. You can produce possible losses and an estimation of how many times you can have these types of losses - once a year, once every five years or once every ten years. You then put it together in your Monte Carlo engine and produce the simulation. I'm not able to think of a better solution at the moment.
Only users who have a paid subscription or are part of a corporate subscription are able to print or copy content.
To access these options, along with all other subscription benefits, please contact info@risk.net or view our subscription options here: http://subscriptions.risk.net/subscribe
You are currently unable to print this content. Please contact info@risk.net to find out more.
You are currently unable to copy this content. Please contact info@risk.net to find out more.
Copyright Infopro Digital Limited. All rights reserved.
As outlined in our terms and conditions, https://www.infopro-digital.com/terms-and-conditions/subscriptions/ (point 2.4), printing is limited to a single copy.
If you would like to purchase additional rights please email info@risk.net
Copyright Infopro Digital Limited. All rights reserved.
You may share this content using our article tools. As outlined in our terms and conditions, https://www.infopro-digital.com/terms-and-conditions/subscriptions/ (clause 2.4), an Authorised User may only make one copy of the materials for their own personal use. You must also comply with the restrictions in clause 2.5.
If you would like to purchase additional rights please email info@risk.net
More on Operational risk
Integrated GRC solutions 2024: market update and vendor landscape
In the face of persistent digitisation challenges and the attendant transformation in business practices, many firms have been struggling to maintain governance and business continuity
Vendor spotlight: Dixtior AML transaction monitoring solutions
The Chartis Research report, AML transaction monitoring solutions, considers how, by working together, financial institutions, vendors and regulators can create more effective anti-money laundering (AML) systems.
Financial crime and compliance50 2024
The detailed analysis for the Financial crime and compliance50 considers firms’ technological advances and strategic direction to provide a complete view of how market leaders are driving transformation in this sector
Automating regulatory compliance and reporting
Flaws in the regulation of the banking sector have been addressed initially by Basel III, implemented last year. Financial institutions can comply with capital and liquidity requirements in a natively integrated yet modular environment by utilising…
Investment banks: the future of risk control
This Risk.net survey report explores the current state of risk controls in investment banks, the challenges of effective engagement across the three lines of defence, and the opportunity to develop a more dynamic approach to first-line risk control
Op risk outlook 2022: the legal perspective
Christoph Kurth, partner of the global financial institutions leadership team at Baker McKenzie, discusses the key themes emerging from Risk.net’s Top 10 op risks 2022 survey and how financial firms can better manage and mitigate the impact of…
Emerging trends in op risk
Karen Man, partner and member of the global financial institutions leadership team at Baker McKenzie, discusses emerging op risks in the wake of the Covid‑19 pandemic, a rise in cyber attacks, concerns around conduct and culture, and the complexities of…
Moving targets: the new rules of conduct risk
How are capital markets firms adapting their approaches to monitoring and managing conduct risk following the Covid‑19 pandemic? In a Risk.net webinar in association with NICE Actimize, the panel discusses changing regulatory requirements, the essentials…