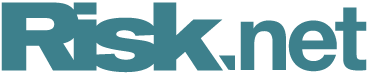
This article was paid for by a contributing third party.More Information.
Rethinking the model lifecycle: from quick fixes to long-term gain
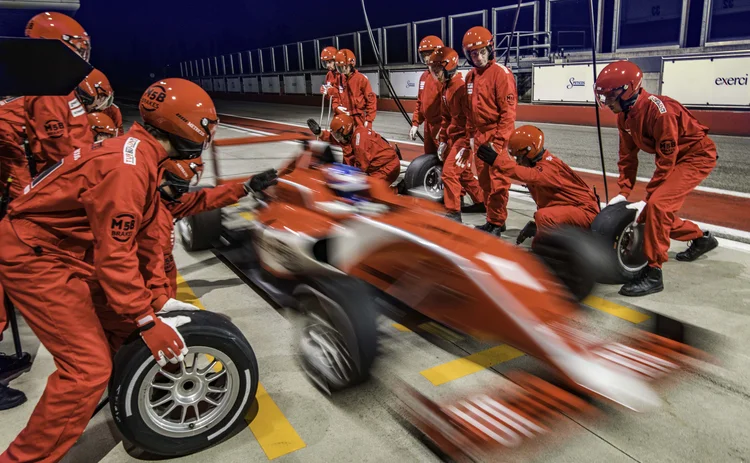
The Covid-19 pandemic has caused widespread disruption to banks’ risk models. Some failed in the crisis while others have required significant overlays or frequent recalibration as extreme volatility has given way to ongoing uncertainty. As banks seek more agile solutions, Risk.net and SAS convened leading model risk practitioners from the US and Canada to discuss the challenges in the current environment and the opportunity to drive longer-term transformation in the model lifecycle
Any crisis exposes weaknesses in the system, but what is crucial is how these are mended and what tools are put in place for safeguarding the future. In the wake of the Covid-19 pandemic and the widespread implementation of governmental and economic stimuli, banks find themselves in limbo. Some risk models are in disrepair, while regulatory measures may also be restricting firms’ agility to bring new models to market.
Credit models have been particularly hard hit. In May, Risk.net reported on the difficulty firms face in managing widely divergent economic data across markets, to the point where many banks are adding “uncertainty buffers” to the provisions calculated by their expected credit loss models. These sentiments echoed by participants at two recent roundtables hosted by Risk.net and SAS in the US and Canada.
According to a McKinsey report published in January 2021, model failures have focused attention on the wider limitations of the model lifecycle. “The causes of the failure include not only Covid-19 effects but also regulatory requirements and models’ increasing time to market,” the report says. “Institutions are realising that even models that have not been significantly affected by these stresses are wanting in other ways.”
The report emphasises that the present crisis is creating a moment in which banks can rethink the entire model landscape and model lifecycle. “The next S-curve for model risk management (MRM) includes new model strategies to address new regulation and changing business needs. Models must become more accurate, so banks need to recalibrate them more frequently and develop new models more rapidly.”
A sustainable operating model is needed, since monitoring, validation and maintenance activities must support the redevelopment and adjustment of models. Quick fixes are unsustainable in the long run, meaning a more permanent overhaul is required.
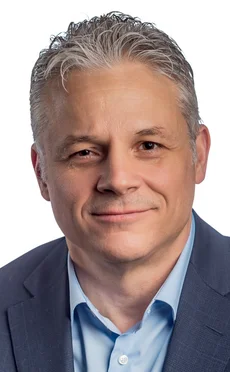
Speaking at the US roundtable, Darryl Ivan, head of the North American risk business practice at SAS, stated that – while every organisation is unique and has its own sets of challenges – there is a broad set of activities and improvements organisations recognise they have to undertake to address the Covid-19 pandemic’s impact on risk models.
“Automating and accelerating model production is one of the key activities. Accelerating and improving your data preparation, use of artificial intelligence (AI)/machine learning and potentially leveraging alternative forms of data is something that often comes up,” Ivan said.
Doing that in a cost-effective way while remaining agile, demonstrating incremental improvements and showing quick time-to-value is always a challenge, he added.
Speaking at the same event, Adam Levy, principal, modelling and valuation at KPMG, said one evident phenomenon is that models do not perform well in unstable times. “We’re in a rapidly changing environment. Things can quickly deteriorate, and it has put immense pressure on a lot of folks from the first line and the second line to try to address that.”
“From a modelling perspective, model risk is more of an ongoing monitoring approach. Staying on top of your models and leveraging technology for performance monitoring is key,” he added.
Prepare to be shocked
Although this crisis may be different in nature to those of the recent past, there is some amount of preparedness thanks to previous shocks to the system, such as those seen in the global financial crisis of 2007–08, model risk experts said.
“When we compare this to the last crisis back in 2007–08, the difference is that the industry is a lot more prepared today. We have more disciplined MRM now so we know what to do; we prepare well for the situation and have clear playbooks,” said Agus Sudjianto, head of model risk at Wells Fargo.
“The frontline knows what to do and what to expect, and I would credit this to the SR 11-7 [supervisory guidance on model risk management from the US Federal Reserve]. The fact we have had SR 11-7 for 10 years ensures the industry is well prepared for the situation that we have.”
Sudjianto added that Wells Fargo is employing validation on demand whereby models can be tested at any given time through a universal protocol.
Model risk leaders at the Canada roundtable sounded a more cautious note. They said that, post-pandemic, no-one can really say they are fully prepared and efficient when it comes to model development, model validation and then monitoring and deployment.
“The question is how can we react faster? And how do we get from a static modelling framework to a more dynamic one?” asked Mario Schlener, partner and financial services risk management practice lead for Canada, and global financial services risk technology alliance and innovation lead at EY.
A more robust monitoring framework is required that can adjust faster to changing market situations. “This is true across risk domains. It is not just credit in the banking book. It’s also in the market and in the trading books, which we are obviously much faster in adopting because it’s a very different risk domain than the credit risk side,” he added.
The crisis has led to models needing to come to market much faster than before. Some institutions have been successful in reducing the time-to-market from an average of 18 months to between three and six months, according to Schlener.
But, overall, a standardisation agreement between different stakeholders needs to take place. The model development team should agree with the model validation team in advance what tools can be used.
“We currently see a lot of work being done and a lot of efficiency conversations taking place. Specifically, institutions that have been successful in reducing the time have been working in that area a lot and trying to solve these problems along the line,” Schlener said.
Data points and technology
Romana Mizdrak, managing director, model risk division at the Office of the Superintendent of Financial Institutions said the Canadian prudential regulator is encouraging organisations to identify opportunities to leverage data points from the pandemic and update models as appropriate.
“Institutions should also bear in mind that the new data is artificially benign. One certainly cannot expect government support will always be there to the same extent,” said Mizdrak.
In terms of silver linings that may have emerged from the crisis, model risk leaders believe the relationship with technology has been enhanced, pointing to evidence of the number of models being developed with open-source code and other technologies.
One US bank participant said he had observed a substantial increase in sample sizes and feature exploration and, with current expected credit loss risk assessment calculations, an explosion in the amount of computer power required.
So, as firms run what-if scenarios around models and adapt the model lifecycle, they will need to ensure the governance controls are sufficiently robust, which was not the case in the past. “The model may be broken, and we know models will be, but the governance cannot be broken, and that’s the key,” Sudjianto said.
A Global Association of Risk Professional (Garp) report published in April warns that, while the reliability of automated analytics is likely to improve over time and get even more user-friendly, the underlying modelling will only become more complicated.
“Model risks will therefore be harder to manage with currently available tools,” according to the report.
“Predicting a reasonable spectrum of possible outcomes is challenging – and the more complex the analytical model, the less transparent the model and its results. While explainability tools can help in understanding the workings and the outcomes of models, less powerful models are often preferred for their simplicity,” the report states.
It is increasingly likely, Garp says, that traditional model validation will change in the wake of digitisation. Consequently, the role of model risk managers is likely to change, partly to account for more unconventional risks.
The move to dynamic modelling
Markets and events will continue to change, so how can firms keep up? “It is how dynamic and how fluid your technology is,” said Ivan. “The challenge lies in how you’re able to adapt, challenging models along the way and adapting analytics,” he added.
Sudjianto noted the explosion of models in the market, many of which are adapted to suit particular customers. This adds to the challenge and requires an agile approach to ensure the firm can react quickly to current events.
The important thing is that firms have a framework for managing model risk, he added. “Frontline and second line know what to do when the model performance degrades, which is a big difference in today’s practice.”
Levy emphasises the role of technology from an MRM perspective. “We’ve come a very long way from having 80 or 100 models in an inventory and doing a validation on every single one of those models.” Technology will continue to be leveraged, he added, to be able to meet the scale of model development, and to increase the numbers of AI-driven models.
The McKinsey report also states that a redefined MRM framework will include all stakeholders and cover the entire model lifecycle. “The model inventory will be reshaped to better support the needs of the business. Standardised processes will provide the foundation for the use of advanced analytical and digital tools and progressive automation.”
Building more sustainable models requires consistently going back to having the right operating model to facilitate the quicker and faster production of those models, right through to retraining.
It is clear that, while the pandemic is a landmark event, it is not the only driver for accelerating the model lifecycle. Pandemics and similar events provide new information to industry professionals to better adapt to an agile approach to MRM in the future.
Sponsored content
Copyright Infopro Digital Limited. All rights reserved.
You may share this content using our article tools. Printing this content is for the sole use of the Authorised User (named subscriber), as outlined in our terms and conditions - https://www.infopro-insight.com/terms-conditions/insight-subscriptions/
If you would like to purchase additional rights please email info@risk.net
Copyright Infopro Digital Limited. All rights reserved.
You may share this content using our article tools. Copying this content is for the sole use of the Authorised User (named subscriber), as outlined in our terms and conditions - https://www.infopro-insight.com/terms-conditions/insight-subscriptions/
If you would like to purchase additional rights please email info@risk.net