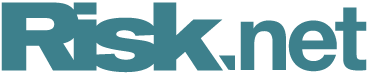
Cat risk: why forecasting climate change is a disaster
Forecasters are poles apart on climate-driven catastrophes; insurers fear there’s worse ahead

The year is 1987. The worst storm in centuries is about to sideswipe the UK with hurricane-strength winds. Notoriously – folklorically – BBC meteorologist Michael Fish addresses a viewer’s concerns: “Earlier on today, apparently, a woman rang the BBC and said she heard there was a hurricane on the way. Well, if you’re watching: don’t worry, there isn’t.”
The storm cost the insurance industry an estimated £2 billion ($2.5 billion). Although Fish claimed his comment was taken out of context
Only users who have a paid subscription or are part of a corporate subscription are able to print or copy content.
To access these options, along with all other subscription benefits, please contact info@risk.net or view our subscription options here: http://subscriptions.risk.net/subscribe
You are currently unable to print this content. Please contact info@risk.net to find out more.
You are currently unable to copy this content. Please contact info@risk.net to find out more.
Copyright Infopro Digital Limited. All rights reserved.
As outlined in our terms and conditions, https://www.infopro-digital.com/terms-and-conditions/subscriptions/ (point 2.4), printing is limited to a single copy.
If you would like to purchase additional rights please email info@risk.net
Copyright Infopro Digital Limited. All rights reserved.
You may share this content using our article tools. As outlined in our terms and conditions, https://www.infopro-digital.com/terms-and-conditions/subscriptions/ (clause 2.4), an Authorised User may only make one copy of the materials for their own personal use. You must also comply with the restrictions in clause 2.5.
If you would like to purchase additional rights please email info@risk.net
More on Risk management
Market knee-jerks keep VAR models on their toes
With a return to volatility, increased backtesting exceptions show banks’ algos are stretched
Why the survival of internal models is vital for financial stability
Risk quants say stampede to standardised approaches heightens herding and systemic risks
Clearing members welcome LME default fund cap
But 2022 nickel crisis still makes hedge funds doubt banks would foot the bill for default at all
Shaking things up: geopolitics and the euro credit risk measure
Gravitational model offers novel way of assessing national and regional risks in new world order
Crypto custody a bit(coin) closer after US accounting U-turn
Federal banking supervisors expected to eventually relax regimes for safeguarding digital assets
EU racing to comply with active account rules
Industry wants simpler route to exemptions ahead of ‘challenging’ deadline for new clearing regime
Banks urged to track vendor AI use, before it’s too late
Veteran third-party risk manager says contract terms and exit plans are crucial safeguards
JSCC plans to open JGB clearing to foreign investors
Clearing house aims to boost cleared market liquidity in Japanese government bonds