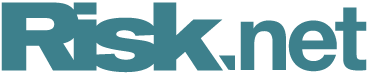
This article was paid for by a contributing third party.More Information.
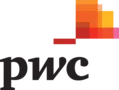
Model risk management transformation
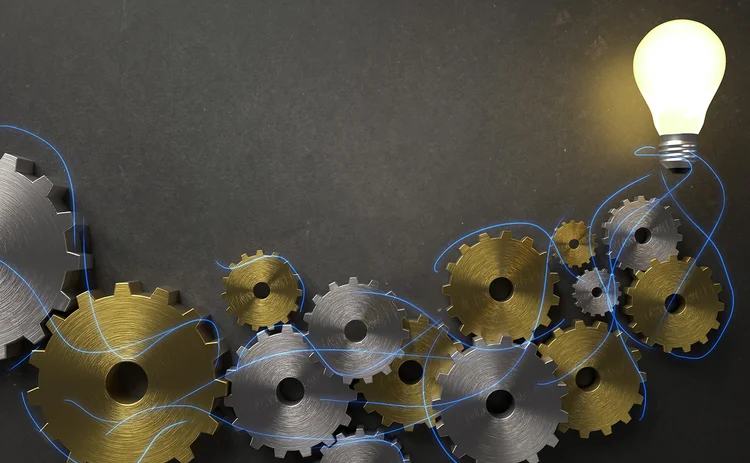
Financial institutions have been maturing their approaches to model risk management (MRM) and – as models become more complex and pervasive, and regulatory expectations continue to increase – leading financial institutions seek faster and further movement. Ashutosh Nawani, head of financial risk management, and George Stylianides, global risk lead, financial services, at PwC Risk Consulting, explore why, by revising their approaches to MRM, institutions are targeting flexible, adaptable and efficient MRM functions that are fit for the future and will deliver real business value
Future-proofing MRM
Within the next three years, leading MRM functions will comprise three pillars as high-performing, enterprise-wide business enablers:
- Holistic mandate-encompassing diverse skills, and embracing technology, regulation and analytics
- A seamless venturing between modelling, reporting and monitoring
- An agile, integrated and responsive framework to organisational and regulatory change.
To achieve such mature framework, transformation enablers are required across a number of areas. Firstly, processes and policies need to change to reflect models’ complexity, materiality and breadth, which will determine the degree of oversight and governance required. Secondly, more automation will be introduced to standardise and industrialise model validation procedures and libraries to drive rapid assessment and deployment. Finally, approaches such as robotic process automation (RPA) can execute routine and repetitive tasks far more efficiently.
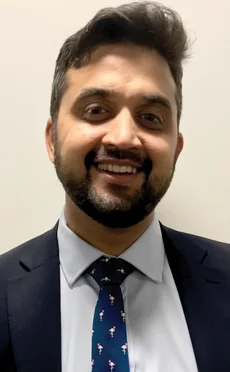
It is imperative for institutions adopting new technology approaches to support an enterprise-level centralised data platform. This will act as a single source of truth for model risk activities such as validation, monitoring and reporting. Furthermore, integrated solution tools can link MRM components to enhance the automation, effectiveness and transparency of governance and oversight. And improvements to MRM operating models should secure cost effectiveness and efficiency by moving from a solely in-house function to a hybrid model that leverages strategic outsourcing partnerships.
Financial institutions expect to gain significant value from accelerating their journeys to a next-generation, more simplified, automated MRM function. There are a number of key opportunities to take action and start making progress, embracing policies, processes, technology and people. Clarity of roles and responsibilities and the alignment of key model management activities is as important as the appropriate use of digital technologies such as RPA, machine learning and advanced analytics. Cultivation of the right talent mix and culture is vital, as is the development of a common data platform.
Model risk for a new wave of models
Following the recent Prudential Regulation Authority supervisory statement on algorithmic trading models, banks are going to face challenges related to the MRM of this new wave of models.
Algorithmic trading, machine learning and artificial intelligence (AI) models allow financial institutions to process a broad variety of data types – both structured and unstructured – and it can be hard to test the integrity and appropriateness of the data used by these algorithms. Consequently, the first challenge is that identification of risks arising from errors in data, its use and its quantification is still in an early stage, but rising very quickly to the top of MRM agendas.
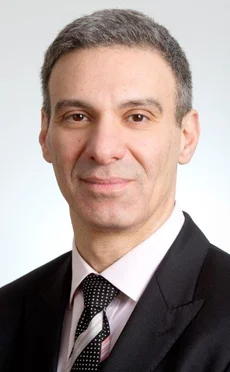
Another challenge MRM managers face is driven by the assessment of the conceptual soundness of these models. This is more complicated than that of standard models, since algorithmic trading/AI/machine learning models are not yet thoroughly understood by practitioners. Therefore, it is hard to evaluate how fit for purpose a given model is for a particular task.
If a model has had a strong performance in predicting future outcomes of a certain quantity up until today, going forward one can never be sure that the model will continue to be fit for purpose when the market enters a new phase.
Consolidation of MRM framework is therefore key for algorithmic trading/AI/machine learning models. The first step is to empower governance framework. The next step is to reassess the definitions of models within the bank to ensure all new model types are covered. These new categories of models should be listed in a dedicated model inventory, including associated controls and other mitigants – kill-switch procedures, for example.
Furthermore, new and existing machine learning and AI models – or other new types in general – should be forced to follow the same steps of testing as other more established model types. Subsequently, controls should be implemented to cap exposure to a counterparty, order attribution, message rate, frequency of order, stale data, and order and position size. Lastly, specific quantitative tests to these models should be implemented. For instance, additional stress-testing of IT systems in dynamic testing environments may be required to understand the impact of extreme events and avoid future disaster in the event of IT system errors.
In conclusion, the ultimate goal is an MRM framework fully embedded in business decision-making that enables proactive risk management for any type of model. While every financial institution will embark on different journeys based on different strategic visions, in the context of current capabilities and regulations the desired final destination remains common to them all.
Model risk management – Special report 2019
Read more
Sponsored content
Copyright Infopro Digital Limited. All rights reserved.
You may share this content using our article tools. Printing this content is for the sole use of the Authorised User (named subscriber), as outlined in our terms and conditions - https://www.infopro-insight.com/terms-conditions/insight-subscriptions/
If you would like to purchase additional rights please email info@risk.net
Copyright Infopro Digital Limited. All rights reserved.
You may share this content using our article tools. Copying this content is for the sole use of the Authorised User (named subscriber), as outlined in our terms and conditions - https://www.infopro-insight.com/terms-conditions/insight-subscriptions/
If you would like to purchase additional rights please email info@risk.net