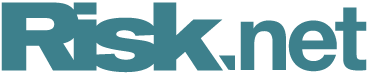
This article was paid for by a contributing third party.More Information.

Fast and accurate KVA using AAD

Traders do not need to resort to crude and unreliable approximations in their capital valuation adjustment (KVA) calculations. Adjoint algorithmic differentiation (AAD) for KVA is rapid, accurate and requires no extra computational power, writes Alexander Sokol, head of quant research at CompatibL.
Calculating the valuation adjustment reflecting the cost of future capital (KVA) is now a key part of evaluating a prospective trade. Recently, several competing views of defining and computing KVA have emerged, some based on the hurdle rate or target return on capital, and others on assessing the effect of the trade on the entire balance sheet of the firm.
Irrespective of which KVA framework is used, calculating future capital for each path and time step of the Monte Carlo simulation is always required. For 5,000 paths and 400 time steps, this means performing capital calculation two million times.
Calculating capital under FRTB is perceived to be difficult and slow, and practitioners are often quick to conclude that performing the same calculation two million times is impossible. Instead, they resort to crude approximations that ignore all but a few leading contributions to capital, and capture even those in a highly stylised and imprecise way. These crude approximations are then superficially ‘improved’ by rescaling the capital requirement by a constant multiplier to match today’s capital.
For realistic portfolios, a comparison with the exact first-principles calculation of capital shows such approximations are so unreliable as to make them practically useless. In fact, the sensitivities to key risk drivers these approximations most often use as proxies for the market risk capital are the very same sensitivities that are well hedged in a realistic portfolio. As a result, even the approximations that seem to work reasonably well for a few swaps in a research paper fail completely for a diversified and well-hedged portfolio encountered in a practical setting. The extent of their failure would be plain were it not concealed by the rescaling to match today’s capital requirement.

There is a much better way of calculating the future capital requirement for KVA. Instead of trying to crudely approximate capital as a function of market risk factors, capital can be calculated from first principles for each path and time step using its complete set of inputs, with fast approximations only employed for simple, atomic inputs to the capital calculation, such as individual trade sensitivities.
A key computational technique that makes this work in practice is adjoint algorithmic differentiation (AAD). With AAD, the calculation of an unlimited number of sensitivities of trade price is around five times slower than the calculation of the trade price itself. As we will see, this makes it possible to compute KVA for sensitivity-based contributions to capital at a computational effort only five times greater than the effort of computing CVA.
Here is a rough outline of the method. We first consider the standardised approach to market risk capital for the trading book (SA-TB). The SA-TB capital requirement consists of components based on delta, vega and curvature, plus the default risk charge and the residual risk add-on (RRAO). We will tackle them in order of increasing complexity. The RRAO is based only on trade notional and is easy to compute. The default risk charge is based on both notional and mark-to-market, and also references the credit rating that may change along the path; its contribution to KVA can also be computed at a fraction of the effort of computing CVA. Thanks to AAD, both delta and vega contributions to KVA can be computed together at a fixed multiple of around five compared with the effort to compute CVA. Finally, the curvature-based charge can be computed by interpolation with computational effort comparable with that of CVA.
Even the internal models approach (IMA) in FRTB is not too complex for first-principles simulation. While IMA requires considerably more complex calculations than SA-TB, a bank able to compute today’s IMA capital has all the computational tools in its possession to implement the same logic for first-principles calculation of IMA KVA. Because of the need to compute expected shortfall (ES) for each path and time step, this calculation would be too slow for practical use without further optimisations. Once again, AAD comes to the rescue. If portfolio P&L is calculated using sensitivities on those dates of the historical stress period when the change in market risk factors is small, and full revaluation is performed only where significant market shocks have occurred, the resulting estimate of ES will be relatively fast to compute and still be far more accurate than the type of crude approximations to the capital requirement that are part of today’s market practice.
A complete set of examples for this calculation can be downloaded from www.modval.org
Read more articles from the FRTB special report
Sponsored content
Copyright Infopro Digital Limited. All rights reserved.
As outlined in our terms and conditions, https://www.infopro-digital.com/terms-and-conditions/subscriptions/ (point 2.4), printing is limited to a single copy.
If you would like to purchase additional rights please email info@risk.net
Copyright Infopro Digital Limited. All rights reserved.
You may share this content using our article tools. As outlined in our terms and conditions, https://www.infopro-digital.com/terms-and-conditions/subscriptions/ (clause 2.4), an Authorised User may only make one copy of the materials for their own personal use. You must also comply with the restrictions in clause 2.5.
If you would like to purchase additional rights please email info@risk.net