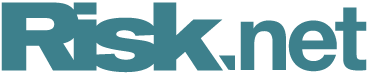
This article was paid for by a contributing third party.More Information.

Trading costs versus arrival price – An intuitive and comprehensive methodology
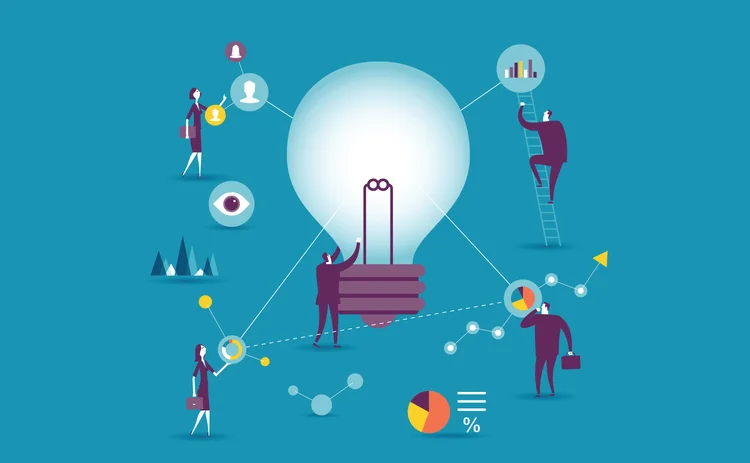
Craig Niven, managing director, cash equity execution at Societe Generale Prime Services explores how a five‑month study allowed the organisation to develop a market impact model using historical data, and why it is key for clients in the long term to build a model that is suitable for them rather than relying on a generic model

Ten months into the revised Markets in Financial Instruments Directive (Mifid II) and buy-side firms are still coming to terms with the greater accountability assigned to proving best execution. The new rules have wrought a new world order, including large, conditional and periodic auctions, and the systematic internaliser regime. As a result, transaction cost analysis has become an even more important tool to navigate this increasingly complex landscape.
The traditional role of pre-trade, on the other hand, was to provide portfolio managers with estimates of trade costs and market impact based on extensive historical trade information for a particular security. It enabled traders to assess the core attributes of orders – such as spread, volatility and volume consumption – and forecast the market impact of using any broker and algo combination to determine the optimal place to send orders. While that remains the case, pre-trade analysis has come into much sharper focus against the current backdrop of regulation, dynamic algorithms, fragmentation and smart-order routing. Traders want a more granular view – down to an individual order – and to match against different venues to see where it is most effective.
This involves looking at trending information in real time to adjust orders accordingly throughout the transaction lifecycle. Advances in artificial intelligence (AI) and machine learning are making these tasks easier as firms can now scrutinise copious amounts of data faster and more efficiently.
According to research by Societe Generale, the veracity of any good model depends on several variables, especially as trading costs can vary across trade type and size, stock characteristics, and global markets and exchanges. However, as with many things, it is not the quantity but the quality and relevance of the data being plugged into the model that is the most important ingredient: big data does not always equate to smart data.
While there are many well-established datasets from exchanges, venues and transaction reports, Mifid II has created alternative trading venues such as systematic internalisers where it has been difficult to discern what proportion of activity is addressable and capable of being interacted against. This is because many brokers have used the systematic internaliser regime to report technical or over-the-counter trades.
To address some of these issues, Societe Generale embarked on a five-month study to develop a market impact model using its own historical data. The objective was to offer an intuitive and comprehensive methodology to measure trading costs versus arrival price. The latter is designed to achieve or outperform the bid/ask midpoint price at the time the order is submitted. It considers the user-assigned level of market risk that identifies the pace of execution as well as the user-defined target percentage of volume.
Methodology
The study defined market impact as shortfall minus alpha period loss. Market impact related to the liquidity displayed in the market since the beginning of the order, while alpha period loss was tied to the volatility of the instrument and fundamental events impacting the instrument pricing and the execution duration. The initial sample was 190,000 client orders, but after filters were applied it was whittled down to around half – 93,700 – of the most relevant orders according to the size, strategy, limit/price and volume cap. Implementation shortfall was chosen as a benchmark because it is one of the most challenging.
Post-trade
For the post-trade section, the model market impact employed two standard factors: participation rate – which measures the market share of the participant during their trading period – and trading duration. Where duration was expressed roughly in days, it was normalised by the instrument’s specific 60-day volatility in per cent. This allowed sample orders on instruments with different volatility profiles but trading on similar periods to be considered.
The simple measure of spread that is the main determinant of liquidity was used and divided into three bands – a small, very liquid spread below 6 basis points, a medium, moderately liquid spread between 6bp and 11bp, and a large, illiquid spread greater than 11bp. The study found the cost could be fairly accurately predicted with a bid offer of less than 11–12bp, but anything with around a 25bp spread would require a different model.
Pre-trade
After validating the post-trade explanatory factors of participation rate and normalised duration, the goal was to develop pre-trade estimators of these factors that minimised the standard deviation. Achieving this allowed post-trade factors to simply be replaced by their respective pre-trade estimates. The list of strategies used in the modelling was limited to benchmarks such as volume-weighted average price, relative value and volume. To achieve this, it had to be ensured that participation rate and end time (duration) could be accurately estimated. These estimators were based on stock-specific volume curves, the specific market configuration (trading hours) and order parameters (open/close included, volume cap and start/end time).
As with the post-trade model, the predictive model produced good results, although it is more difficult to achieve very high values of adjusted r2 because of the noisy nature of market impact. R2 is not a gauge of the performance of a portfolio but a measure of the correlation of the portfolio’s returns to those of the benchmark. It can be thought of as a percentage, with 70–100% constituting the high end and 1–40% the low end of the spectrum.
The calculations showed that the market impact sensitivity to the duration was higher than the one to the participation rate that mirrors the findings on the post-trade study. Overall, the results revealed that the model was promising to predict the market impact on average for 50% of the r2 for the most liquid stocks, with a good confidence interval for each of the parameters. However, it was unable to make the same assumptions for the illiquid stocks in the sample as a result of poor outcomes.
Putting the model to the test
To validate its model, Societe Generale selected a set of 5,000 client orders. It looked at the distribution of the estimation error, defined as the difference between the realised value and the estimated value the model predicted. Despite the din of market impact, the pre-trade model could be applied to a large proportion of its instruments universe, and it was easy to implement as an estimator into its strategies that targeted the arrival price. However, the study noted that creating a single model that catered to all liquidity groups of instruments was too ambitious. Most illiquid instruments with adjusted factors and functions required a dedicated market impact model.
Moreover, buy-side firms should not only rely on generic models from their brokers, but start to develop their own proprietary solutions over the longer term. This is particularly true in the wake of Mifid II as different execution venues such as block trading platforms and systematic internalisers have gained traction, while AI and machine learning are becoming embedded in the trading process. For example, algo wheels are increasingly being used by traders to assess, monitor and justify algo and broker choices. They help remove subjectivity from the selection process and guide buy-side firms to the counterparty or strategy that best suits their pre-defined execution criteria. They not only standardise the process but allow traders to retrieve unbiased data on the performance of the different algo. The main benefits are performance gains from improved execution quality and workflow efficiency from automating small order flow.
Building their own pre-trade estimate models will help buy-side traders navigate this changing post-Mifid II execution landscape. While a broker’s model has its purposes, it may prove too generic and not completely fit with some traders’ requirements. Plugging their own historical trade information into a pre‑trade cost estimate model will provide traders with a much stronger starting point to the trade execution process. It will not only enable them to analyse their own flow to better forecast costs, but ensure they select the most suitable algo wheel or other execution tool.
Equally as important, having an internal model can help serve as a powerful marketing tool. Regulation may be pushing buy-side firms to take greater responsibility to prove best execution, but shifting market dynamics are also driving them to improve their offering. A prolonged low interest rate period in Europe combined with increased scrutiny on fees and a move to passive investing has put intense pressure on fund managers to generate returns and differentiate. Tools such as pre-trade cost analysis models that can leverage data to offer greater insights into the transaction lifecycle and enhance performance can be one way to sharpen the competitive edge.
The importance of building an accurate pre‑trade cost estimate model
- Pre-trade is a core component of the best execution process. The increasing focus on best execution from a regulatory perspective has propelled pre-trade into mandatory status.
- Research by Societe Generale has shown that the accuracy of any pre‑trade cost estimate model depends on many variables, but the main one is to have accurate and relevant data.
- A Societe Generale study found that anything with a bid offer of less than 11–12 basis points can predict the cost fairly accurately, while a roughly 25bp spread would require a different model.
- Trading costs vary across trade type, stock characteristics, trade size, and international markets and exchanges.
- Building an accurate model requires the use of big data.
- Clients should think about building the right model in the longer term and not relying on a generic broker model. They should analyse their own flow to better forecast the costs to pick the most appropriate execution tool. This has become increasingly relevant with the proliferation of algo wheel usage. It can also serve as a powerful marketing tool because firms are under pressure to generate returns.
Sponsored content
Copyright Infopro Digital Limited. All rights reserved.
As outlined in our terms and conditions, https://www.infopro-digital.com/terms-and-conditions/subscriptions/ (point 2.4), printing is limited to a single copy.
If you would like to purchase additional rights please email info@risk.net
Copyright Infopro Digital Limited. All rights reserved.
You may share this content using our article tools. As outlined in our terms and conditions, https://www.infopro-digital.com/terms-and-conditions/subscriptions/ (clause 2.4), an Authorised User may only make one copy of the materials for their own personal use. You must also comply with the restrictions in clause 2.5.
If you would like to purchase additional rights please email info@risk.net