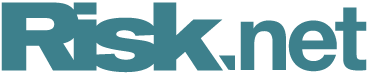
Solving the enigma of the volatility smiles
Has the problem of jointly calibrating the volatility smiles of the Vix and S&P 500 been solved?
For years, art historians and scientists have tried to unlock the mysteries of the Mona Lisa’s smile, which flickers and fades when viewed from different angles. Quants are similarly beguiled by the so-called volatility smile, the characteristic shape obtained by plotting implied volatilities against the strikes of an option.
Since the introduction of derivatives on the Vix index in 2006, many have sought to jointly calibrate the smiles of S&P and Vix options. One may expect them to match, since the Vix is a measure of the 30-day implied volatility of an option on the S&P 500. But their smiles are different: the S&P’s resembles a smirk to the left, while the Vix smirks to the right.
This reflects the different skews, or slopes, of the Vix and S&P. The implied volatility of an S&P option is a decreasing function of the strike, while the implied volatility of a Vix option is an increasing function of the strike.
Conventional volatility models cannot calibrate both.
This matters, because Vix options are commonly used to hedge volatility exposure on the S&P 500. If the two options are modelled separately, they might not fit market data consistently, and in theory an arbitrage may arise.
Julien Guyon, a senior quantitative analyst at Bloomberg, published his solution to the problem in Risk.net in early April.
Rather than modelling continuous time dynamics, Guyon’s discrete-time model tries to fit just three data points: the S&P and Vix smiles at a given Vix future expiry date, and the S&P smile 30 days after. “I realised that in order to easily decouple the at-the-money skew of the S&P and at-the-money implied volatility of the Vix, it was much more convenient to work in discrete time”, says Guyon.
The key was designing a joint distribution for the three variables consistent with each marginal distribution, subject to a set of constraints. This is an optimal transport problem, with known solutions. The calibration is then performed with a non-parametric approach, which, according to Guyon, allows for a perfect calibration to market data.
One of the main findings of this research is the absence – at least in the sample – of joint arbitrage opportunities between Vix and S&P options. In fact, Guyon proves this is a consequence of the existence of a model that calibrates both smiles.
Guyon’s work, which was circulating in the quant community for some months, inspired Mathieu Rosenbaum, professor at École Polytechnique in Paris, to devise a completely different solution to the same problem.
If you want to fit the S&P smile, the rough vol is going to be better than any other classical stochastic volatility models
Mathieu Rosenbaum, École Polytechnique
Together with Jim Gatheral, a professor of mathematics at Baruch College in New York, and Paul Jusselin, a PhD student in financial mathematics at École Polytechnique, Rosenbaum developed a diffusion model based on rough volatility – a concept pioneered by Gatheral, Thibault Jaisson and Rosenbaum in 2014 and widely explored by other quants in recent years.
“If you want to fit the S&P smile, the rough vol is going to be better than any other classical stochastic volatility models,” says Rosenbaum.
The second ingredient in the model is the so-called Zumbach effect, which accounts for the way past volatility and price dynamics influence future volatility.
In total, the model has only five parameters, “with easy interpretation for all of them”, Rosenbaum says. He is very satisfied with the results: “I call it perfect fit with five parameters.”
Guyon begs to differ. “I respectfully disagree, because when you look closely at their numerical results, you can recognise the kind of issues I had to deal with: not enough at-the-money S&P skew,” he says.
“But I must say their model is very interesting,” Guyon adds. “They get a very decent joint fit, especially considering the very small number of parameters.”
The fit could be improved. A paper published last year by Blanka Horvath of King’s College, Aitor Muguruza of Natixis and Mehdi Tomas at École Polytechnique, showed how neural networks can be used to better calibrate rough volatility models. Rosenbaum is working on implementing this.
Others argue the problem has already been solved.
If you have a model in which the index can jump down and Vix can simultaneously jump up, you can reproduce both the SPX and the Vix skew with just one parameter
Rama Cont, Oxford University
In 2013, Rama Cont, professor of mathematical finance at Oxford University, and Thomas Kokholm of Aarhus University, proposed a jump-diffusion model based on a simple principle. “If you have a model in which the index can jump down and Vix can simultaneously jump up, you can reproduce both the SPX and the Vix skew with just one parameter,” says Cont.
Such co-moves in the index and its volatility link the two smiles and explain the opposing skews.
Cont first developed his jump-diffusion model to address a practical problem in the industry. Initial margins for options and futures on Vix and S&P were computed separately, using two different models. “That led to an incorrect representation of volatility risk,” he says. “With a consistent joint model, when you hedge one instrument with the other, the model shows that the risk is reduced and avoids overestimating risk,” he explains.
This approach has not gained traction with banks, however. A head quant at a global investment bank says he is sceptical of volatility models with jumps because of their limited tractability and the difficulties with risk managing and calibrating them. “Jumps lead to incomplete markets, so it is rare that they are adopted to price options in production,” he says.
Instead, banks model the two option smiles independently: the Black-Scholes implied volatility of the Vix and its corresponding futures are marked using observed options prices, while the S&P volatility surface is marked separately to reconcile with the theoretical prices of the Vix and Vix futures, with certain convexity adjustments. This approach is theoretically inconsistent, and care must be taken to ensure the S&P implied volatility surface is reflected properly in the Vix and Vix futures prices when calculating risk.
“It’s a very approximate solution,” admits the head quant, “but that is what most banks have to work with.”
Still, he acknowledges that the two new models from Guyon and Rosenbaum et al are an important step towards reconciling the two smiles.
“These models ultimately should be useful for live risk management, and include risk sensitivities, but they still have to provide a solution that is tractable and reasonably fit to reality in continuous time,” he says. “Quants have real stress scenarios from 2018 and from the current Covid-19 crisis. If their models fail to provide good risk management, they might turn out to be more dangerous than useful, and their mispricing could lead to major losses.”
The joint calibration of the Vix and S&P smiles has not yet been satisfactorily achieved and there is no consensus on the way forward. Rosenbaum thinks the rough volatility model will be up to the job once the neural network calibration is implemented. Cont still reckons his 2013 jump model already solves the problem. Guyon is working on a continuous-time extension of his discrete time approach, to allow for risk management in real time.
When the problem is solved, there will be fewer smiles to explain.
Only users who have a paid subscription or are part of a corporate subscription are able to print or copy content.
To access these options, along with all other subscription benefits, please contact info@risk.net or view our subscription options here: http://subscriptions.risk.net/subscribe
You are currently unable to print this content. Please contact info@risk.net to find out more.
You are currently unable to copy this content. Please contact info@risk.net to find out more.
Copyright Infopro Digital Limited. All rights reserved.
As outlined in our terms and conditions, https://www.infopro-digital.com/terms-and-conditions/subscriptions/ (point 2.4), printing is limited to a single copy.
If you would like to purchase additional rights please email info@risk.net
Copyright Infopro Digital Limited. All rights reserved.
You may share this content using our article tools. As outlined in our terms and conditions, https://www.infopro-digital.com/terms-and-conditions/subscriptions/ (clause 2.4), an Authorised User may only make one copy of the materials for their own personal use. You must also comply with the restrictions in clause 2.5.
If you would like to purchase additional rights please email info@risk.net
More on Our take
Podcast: Lorenzo Ravagli on why the skew is for the many
JP Morgan quant proposes a unified framework for trading the volatility skew premium
Quants see promise in DeBerta’s untangled reading
Improved language models are able to grasp context better
Counterparty risk model links defaults to portfolio values
Fed’s Michael Pykhtin proposes using copula models to capture effects of margin calls on default risk
Does Basel’s internal loss multiplier add up?
As US agencies mull capital reforms, one regulator questions past losses as an indicator of future op risk
Is JSCC-CFTC stalemate about to be broken?
Japan CCP gains allies in battle to clear yen swaps for US clients, but CFTC shakeup could dash hopes
What T+1 risk? Dealers shake off FX concerns
Predictions of increased settlement risk and later-in-the-day trading have yet to materialise
Go your own way: departures pose new challenges for CFTC
Loss of Democratic majority would impede chairman’s ambitions for regulatory agenda
Altice’s dropdown is a warning for European creditors
Carve-out used to shield assets from lenders may occur in a fifth of European deals