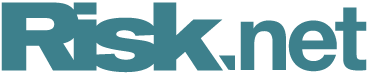
Quant Guide 2017: Rutgers University
Piscataway, USA

Financial Statistics and Risk Management Master
MSc in Mathematical Finance | metrics table at end of article
Rutgers offers two quantitative finance courses, each run by a different department: the statistics and mathematics departments. The financial statistics and risk management programme, as its name implies, is more focused on statistics, and the methods taught are more geared towards those used by the buy side. The mathematical finance programme is organised by the mathematics department. It aims to provide a balanced focus between investment and investment banking. A separate quant finance-focused course, not featured in Risk.net’s guide, is offered by Rutgers Business School.
Financial statistics and risk management
The financial statistics and risk management programme offers courses on machine learning, risk management and advanced statistical methods. “The emphasis is on how to backtest models, prevent data snooping, and data problems. It is a much deeper level of data analysis and data validation than in most other courses,” says Neville O’Reilly, associate programme director.
He says the department is looking to add further data management courses to the programme in the form of certificates, likely to happen in autumn 2018.
The master’s – a 15-month programme founded in 2011 – was sculpted in response to the financial crisis, says O’Reilly; the faculty sought to create a programme that reflected the changing demands of the industry.
“There was a need after the financial crisis for less top-down modelling of derivatives pricing and complicated derivatives like collateralised debt obligations and tranches, and for more bottom-up data analysis,” he says.
Of 350 applicants, around 35 have typically been admitted annually. This is set to decrease in future to 30.
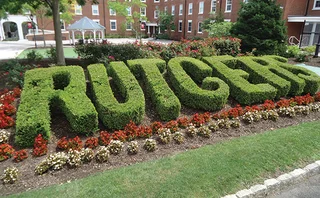
Students are kept up to date with current trends by visiting practitioners. “I think there are quite a few changes in both investment and risk management that we try to keep track of and expose the students to,” says O’Reilly. “And part of that effort is in having practitioners come and talk to the students or give workshops.”
Every two weeks a practitioner will lead a workshop or seminar to explain current research or trends in the industry. Gary Chropuvka, for example, head of customised beta strategies, alternative investment strategies and tax-advantaged core strategies businesses at Goldman Sachs, has previously given a seminar on smart beta.
Some lecture courses are also taught by practitioners. The advanced statistics for finance and algorithmic trading courses are taught by Gordon Ritter, for example, a senior portfolio manager at GSA Capital Partners. Another opportunity to meet industry practitioners is through the quant finance club. It invites speakers to the university, hosts career fairs and members can also participate in domestic competitions.
There is also the opportunity to undertake an internship and around 60% of the students typically do. The department has partnered with Imagine Software, a risk analytics consultancy, which employs six or eight interns every semester. Students not doing an internship will instead work on a project supervised by either a practitioner or a member of the faculty.
Patrick Ewane graduated from Rutgers in 2013 and is now working in real estate investment strategy at BlackRock. He was attracted to the course’s statistical nature.
“They are not your traditional financial engineering programme. In the past, quantitative finance programmes focused on heavy mathematics and stochastic analysis for derivative products and structuring. That made sense prior to the crisis but not now.” The demand now is in statistical analysis, high-frequency trading and statistical arbitrage, says Ewane.
After graduating, Ewane got his first job at Cantor Fitzgerald in equity research. For Ewane the internship was a crucial element of the master’s and having contact with practitioners helped with this. “I was able to use the connections of the professors and in-residence practitioners we had to secure an internship,” says Ewane.
Mathematical finance
Numerical analysis is a particularly strong element of Rutgers’ mathematical finance master’s, says Paul Feehan, director of the programme. “Numerical analysis is taught over two semesters here, while most other programmes set only one semester. We can therefore cover a much broader array of topics in a much greater depth than would typically be done in other courses.”
These numerical courses are linked with the computational courses, and as a result the students can be given projects with greater scope than might otherwise be possible.
Students are prepared for the computational courses with a C++ primer designed by the engineering department. Overall the course is a more classical quant programme, and more mathematically inclined than Rutgers’ statistical programme. The faculty targets 50 students for admission.
The programme was created by Feehan in 2006. Having worked for a few years at Bloomberg and JP Morgan, and seen first-hand how the industry picks its quants, Feehan designed the programme to produce ready-made quants with both rigorous mathematical training and the required specialised knowledge.
“We want to try to encourage firms – big and small – to consider master’s students whenever possible, rather than hire a PhD student with a more general background,” says Feehan.
Recognising the increasing demand for more statistical skills among buy-side firms and the decreasing demand for complex pricing models, the course has adapted to reflect the change. “We’ve increased offerings for students in statistics, data science, machine learning and data analysis,” says Feehan.
Beginning later this year, students will also have the opportunity to take extra courses with a particular speciality. “Our target is to have certificates in data science and risk management, and we’re adding courses to support both of those.” Certificates are in addition to a degree.
There are plenty of opportunities for students to meet industry practitioners. “Every week we have a representative from the financial industry come in to give a presentation to our students. They tell them what they do, what their firms do and how the industry is changing,” Feehan says. In addition, the industry-oriented elective courses are taught by practitioners and there is an industry advisory board offering advice on industry trends and how the programme should adapt. There is also the opportunity to intern. Internships are not mandatory but they are encouraged and support is given to students seeking one.
Alvin Huang works in compliance surveillance at JP Morgan, looking at issues such as spoofing, front-running or benchmark manipulation. He graduated from the course in 2009 but already had industry experience as a trader, having graduated with a bachelor’s in biochemistry in 2000. “I wanted to be able to quantify, automate, and ultimately scale certain equity trading strategies,” says Huang. “I also wanted to expand my knowledge in financial derivatives and modelling.” Huang was attracted by the mathematical orientation of the course.
Particularly impressive for Huang was the breadth of the training in programming. While there was a course specific to C++, other courses encouraged the adoption of other languages such as R and MatLab. “Whether it is in trading, operations or compliance surveillance, without the rigorous programming background, I definitely would not be where I am today.”
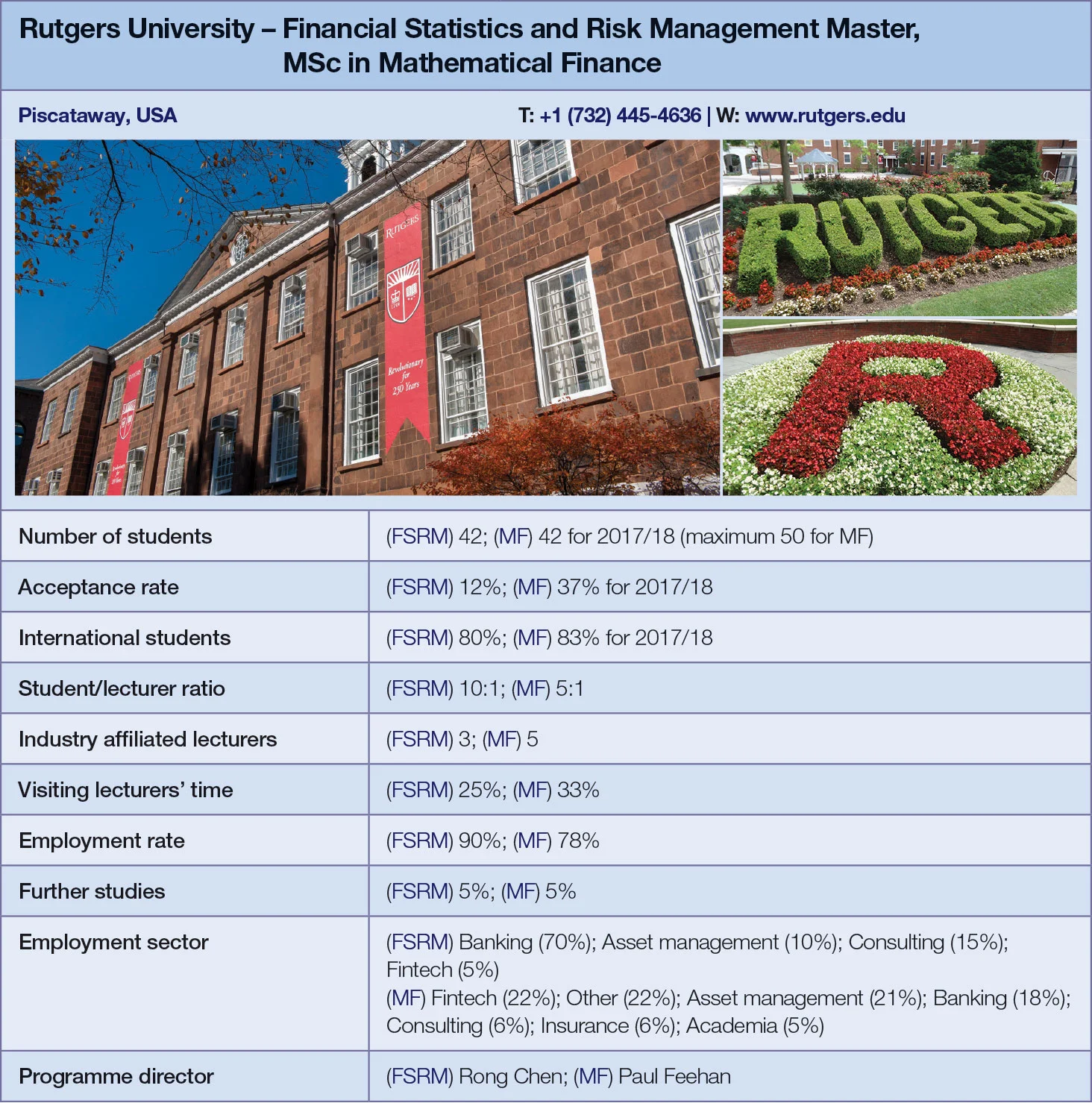
Click here for links to the other universities and an explanation of how to read the metrics tables
Only users who have a paid subscription or are part of a corporate subscription are able to print or copy content.
To access these options, along with all other subscription benefits, please contact info@risk.net or view our subscription options here: http://subscriptions.risk.net/subscribe
You are currently unable to print this content. Please contact info@risk.net to find out more.
You are currently unable to copy this content. Please contact info@risk.net to find out more.
Copyright Infopro Digital Limited. All rights reserved.
As outlined in our terms and conditions, https://www.infopro-digital.com/terms-and-conditions/subscriptions/ (point 2.4), printing is limited to a single copy.
If you would like to purchase additional rights please email info@risk.net
Copyright Infopro Digital Limited. All rights reserved.
You may share this content using our article tools. As outlined in our terms and conditions, https://www.infopro-digital.com/terms-and-conditions/subscriptions/ (clause 2.4), an Authorised User may only make one copy of the materials for their own personal use. You must also comply with the restrictions in clause 2.5.
If you would like to purchase additional rights please email info@risk.net
More on Quantitative finance
Quant Finance Master’s Guide 2023
Risk.net’s guide to the world’s leading quant master’s programmes, with the top 25 schools ranked
Baruch topples Princeton in Risk.net’s quant master’s rankings
US schools cement top five dominance as graduate salaries soar
Is it worth doing a quant master’s degree?
UBS’s Gordon Lee – veteran quant and grad student supervisor – asks the hard question
Starting salaries jump for top quant grads
Quant Guide 2022: Goldman’s move to pay postgrads more is pushing up incomes, says programme director
Quant Finance Master’s Guide 2022
Risk.net’s guide to the world’s leading quant master’s programmes, with the top 25 schools ranked
Princeton, Baruch and Berkeley top for quant master’s degrees
Eight of 10 leading schools for quantitative finance programmes are based in US, latest rankings show
Quant grad conveyor belt stalls as banks retrench
Jobs market is long quant graduates, short vacancies – but hiring freeze shows signs of thawing
Quant Finance Master’s Guide 2021
Risk.net’s guide to the world’s leading quant master’s programmes, with the top 25 schools ranked