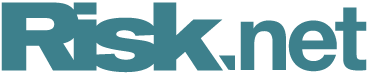
The case for reinforcement learning in quant finance
The technology behind Google’s AlphaGo has been strangely overlooked by quants
When Google’s Deepmind defeated the world’s top Go player in 2016, it was seen as a breakthrough for artificial intelligence. But the technique used to train AlphaGo, known as reinforcement learning (RL), has not gained much traction in finance, despite its ability to handle complex, multi-period decisions.
Igor Halperin, a senior quantitative researcher at Fidelity Investments, thinks it’s time for that to change: “RL is the best and most natural solution to most of the problems we have in quantitative finance,” he says.
He argues that nearly all problems in quantitative finance – including options pricing, dynamic portfolio optimisation and dynamic wealth management – can be solved with RL or inverse RL, or a combination of the two.
RL techniques work sequentially. At each stage, the algorithm observes the reward obtained in previous stages and proceeds accordingly, trying as many combinations of actions as possible to maximise a given reward function.
Halperin and Matthew Dixon, assistant professor at the Illinois Institute of Technology in Chicago, have published a research paper on the application of RL to dynamic wealth management.
They spotlight two techniques, which can be used either individually or in combination. The first is G-learning, a probabilistic extension of the Q-learning approach popularised by Deepmind. The advantage of G-learning – which is relatively new to finance, despite being well established in other fields – is that it can handle noisy environments and high dimensionality, which Q-learning struggles with.
For this reason, a previous effort by Gordon Ritter to apply Q-learning to dynamic portfolio optimisation was limited to a small number of assets.
“[Q-learning] couldn’t manage a portfolio of 500 stocks and it doesn’t cope well with noisy environments such as financial markets,” says Halperin.
RL is better than Black-Scholes and risk-neutral pricing in general, which makes more harm than good
Igor Halperin, Fidelity Investments
G-learning does not suffer from this problem. Given a reward function – in this case, the maximisation of wealth in a given time horizon – it can find the optimal combination of actions to reach a target outcome using the available historical data.
The second technique, which Halperin and Dixon introduce for the first time in their paper, is called generative inverse reinforcement learning, or GIRL. This works the opposite way to G-learning. GIRL takes the outcomes of strategies – the holdings and returns of a portfolio – and works backwards to infer what investment strategy the manager followed.
Halperin says the tools can be combined to create a robo-advisory solution. GIRL can be used to learn existing strategies, which G-learning can then optimally replicate for clients. The adviser can then potentially tailor solutions to clients’ objectives and level of risk aversion.
Other potential applications include minimising market impact in trade execution. The Royal Bank of Canada’s research centre, Borealis AI, has already used RL to develop a new trade execution system for the bank, called Aiden.
Halperin is also convinced RL can be successfully applied to price derivatives. “RL is better than Black-Scholes and risk-neutral pricing in general, which makes more harm than good,” he says. “Option pricing is all about managing risk, but the main assumption of risk-neutral formulation is that there is no risk, which is self-contradictory.”
Halperin and Dixon’s research is still in the experimental phase and has not been tested in practice, but the authors are confident about its effectiveness.
So why is RL missing from most quants’ existing toolkits? Matthew Taylor, associate professor of computer science at the University of Alberta, reckons it might be down to a scarcity of expertise. “In general, RL is not used much in finance, at least publicly,” he says. “There is a barrier to entry for financial institutions and there aren’t enough reinforcement learning professionals, or enough experts for all the potential.”
The work of Halperin, Dixon and others may fuel wider efforts to apply RL in finance.
Only users who have a paid subscription or are part of a corporate subscription are able to print or copy content.
To access these options, along with all other subscription benefits, please contact info@risk.net or view our subscription options here: http://subscriptions.risk.net/subscribe
You are currently unable to print this content. Please contact info@risk.net to find out more.
You are currently unable to copy this content. Please contact info@risk.net to find out more.
Copyright Infopro Digital Limited. All rights reserved.
You may share this content using our article tools. Printing this content is for the sole use of the Authorised User (named subscriber), as outlined in our terms and conditions - https://www.infopro-insight.com/terms-conditions/insight-subscriptions/
If you would like to purchase additional rights please email info@risk.net
Copyright Infopro Digital Limited. All rights reserved.
You may share this content using our article tools. Copying this content is for the sole use of the Authorised User (named subscriber), as outlined in our terms and conditions - https://www.infopro-insight.com/terms-conditions/insight-subscriptions/
If you would like to purchase additional rights please email info@risk.net
More on Our take
Hedge funds must race the clock to check their dealer-rule status
Working out whether a firm is caught by SEC registration requirement could take months
Filling gaps in market data with optimal transport
Julius Baer quant proposes novel way to generate accurate prices for illiquid maturities
Why Europe still awaits a private credit CLO
Tricky questions face managers that plan to launch the structure on the continent
The signs of tacit collusion in the dividend play trade
Game theory and real-world data point to a different understanding of how arbitrage in markets works
Decades of history says you can beat high inflation with quality
Factors such as momentum and value generally outperform the market irrespective of inflation, but new research suggests quality stocks are best when prices are rising rapidly
Esma faces tough task in implementing Emir 3.0
EU regulator must contend with tight timeframes and increasing workload without additional resources
Quants are using language models to map what causes what
GPT-4 does a surprisingly good job of separating causation from correlation
China stock sell-off will test securities firms’ risk managers
Regulatory measures to support stock market could add to risks facing securities sector