Journal of Risk
ISSN:
1465-1211 (print)
1755-2842 (online)
Editor-in-chief: Farid AitSahlia
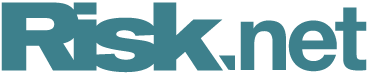
Recursive estimation of the exponentially weighted moving average model
Radek Hendrych and Tomáš Cipra
Need to know
- The (robust) recursive estimation algorithms suitable for the EWMA model are suggested.
- The prediction ability of the proposed (robust) recursive estimation schemes is investigated.
- The priorities of the introduced recursive estimation procedures are demonstrated.
Abstract
The exponentially weighted moving average (EWMA) model is a particular modeling scheme, supported by RiskMetrics, that is capable of forecasting the current level of volatility of financial time series. It is designed to track changes in the conditional variance of financial returns by assigning exponentially decreasing weights to observed past squared measurements. The aim of this paper is twofold. First, it introduces two recursive estimation algorithms that are appropriate for the EWMA model. Both are derived by employing the general recursive prediction error scheme. Moreover, they represent a computationally effective alternative to already established nonrecursive estimation strategies since they are effective in terms of memory storage, computational complexity and detecting structural changes. Second, this paper investigates the prediction ability of the proposed recursive estimation schemes when compared with other common (nonrecursive) estimation methods. The priorities of the suggested recursive estimators are demonstrated by means of a simulation study and an extensive empirical case study of eighteen key world stock indexes. Combinations of recursive predictions are also studied. Such a strategy can be recommended due to its advantageous properties when predicting volatility.
Copyright Infopro Digital Limited. All rights reserved.
You may share this content using our article tools. Printing this content is for the sole use of the Authorised User (named subscriber), as outlined in our terms and conditions - https://www.infopro-insight.com/terms-conditions/insight-subscriptions/
If you would like to purchase additional rights please email info@risk.net
Copyright Infopro Digital Limited. All rights reserved.
You may share this content using our article tools. Copying this content is for the sole use of the Authorised User (named subscriber), as outlined in our terms and conditions - https://www.infopro-insight.com/terms-conditions/insight-subscriptions/
If you would like to purchase additional rights please email info@risk.net