Journal of Operational Risk
ISSN:
1744-6740 (print)
1755-2710 (online)
Editor-in-chief: Marcelo Cruz
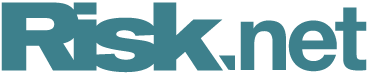
A nonlinear analysis of operational risk events in Australian banks
Yifei Li, Neil Allan and John Evans
Need to know
- Cladistics analysis provides new insights into operational risk events in banks by identifying the combinations of underlying characteristics that were involved in each risk event.
- The analysis for Australian banks indicates that poor control has been by far the main characteristic of operational risk event losses.
- The analysis has identified the main characteristics involved with operational risk losses and these are shown to be consistent over time, thus enabling management to concentrate on these characteristics to reduce future losses.
Abstract
We propose a methodology applied to complex systems to analyze operational risk events in banks, with the objective of determining an understanding of the key characteristics and their relationships in initiating operational risk losses. We applied our methodology to operational risk losses in Australian banks over the period 2010-14. The analysis identified that there are a small number of characteristics that are common to many operational risk events, and these "level 1" characteristics are stable across time, which implies operational risk losses could be controlled by managing these characteristics. The methodology adds value to the existing analysis by identifying the main characteristics of operational risk events in a rigorous manner.
Copyright Infopro Digital Limited. All rights reserved.
As outlined in our terms and conditions, https://www.infopro-digital.com/terms-and-conditions/subscriptions/ (point 2.4), printing is limited to a single copy.
If you would like to purchase additional rights please email info@risk.net
Copyright Infopro Digital Limited. All rights reserved.
You may share this content using our article tools. As outlined in our terms and conditions, https://www.infopro-digital.com/terms-and-conditions/subscriptions/ (clause 2.4), an Authorised User may only make one copy of the materials for their own personal use. You must also comply with the restrictions in clause 2.5.
If you would like to purchase additional rights please email info@risk.net