Journal of Financial Market Infrastructures
ISSN:
2049-5404 (print)
2049-5412 (online)
Editor-in-chief: Manmohan Singh
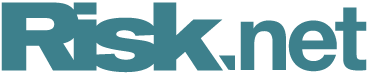
Initial margin model sensitivity analysis and volatility estimation
Need to know
- A variety of volatility estimation techniques used in initial margin models are applied to a long history of the S&P 500 index.
- A range of acceptable parameters are identified based on statistical properties of the worst loss over a margin period of risk.
- The proposed method provides a discriminating approach to margin model sensitivity analysis.
Abstract
The advent of mandatory central clearing for certain types of over-the-counter derivatives, and margin requirements for others, means that margin is now the most important mitigation mechanism for many counterparty credit risks. Initial margin requirements are typically calculated using risk-based margin models, and these models must be tested to ensure they are prudent. However, two different margin models can calculate substantially different levels of margin and still pass the usual tests. This paper presents a new approach to parameter selection based on the statistical properties of the worst loss over a margin period of risk estimated by the margin model under scrutiny. This measure is related to risk estimated at a fixed confidence interval, but it leads to a more powerful test that is better able to justify the choice of parameters used in margin models. The test proposed is used on a variety of volatility estimation techniques applied to a long history of returns of the Standard & Poor’s 500 index. Some well-known techniques, including exponentially weighted moving average volatility estimation and generalized autoregressive conditional heteroscedasticity, are considered, and novel approaches derived from signal processing are also analyzed. In each case, a range of model parameters that gives rise to acceptable risk estimates is identified.
Copyright Infopro Digital Limited. All rights reserved.
You may share this content using our article tools. Printing this content is for the sole use of the Authorised User (named subscriber), as outlined in our terms and conditions - https://www.infopro-insight.com/terms-conditions/insight-subscriptions/
If you would like to purchase additional rights please email info@risk.net
Copyright Infopro Digital Limited. All rights reserved.
You may share this content using our article tools. Copying this content is for the sole use of the Authorised User (named subscriber), as outlined in our terms and conditions - https://www.infopro-insight.com/terms-conditions/insight-subscriptions/
If you would like to purchase additional rights please email info@risk.net