Journal of Financial Market Infrastructures
ISSN:
2049-5404 (print)
2049-5412 (online)
Editor-in-chief: Manmohan Singh
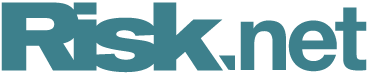
A balanced approach to central counterparty margining
Need to know
- This paper presents the potential benefits and risks of margin setting frameworks which incorporate expert judgment as a complement to model driven inputs relative to frameworks that rely primarily on automated models. We argue for a balance between these two approaches.
- Many global CCPs operate in multiple jurisdictions and, as a result, must adapt to multiple regulatory frameworks. It is important that CCPs be able to tailor their margin models to the unique risks associated with the products they clear.
- While the margin model framework is important, it is equally, if not more, important to examine margin collection practices and their impact on risk.
Abstract
This paper is meant to serve as a comparison of the approaches and margin models employed by central clearing counterparties (CCPs). We look at two different approaches: fully automated margin models and margin models that incorporate expert judgment. The latter type of model utilizes value-at-risk (VaR) methodologies, and the outputs of these VaR methodologies are subject to manual review before the final parameters are determined. The former type of model does not involve manual review or any alteration of the parameters. While these approaches may differ greatly, there are components of each that are critical to prudent margin setting. This paper concludes that a combination of data-driven models and applied expert judgment most appropriately captures the risks in centrally cleared markets, emphasizing the importance of expert judgment in margin setting. It is important to outline the goals of initial margin as a risk management tool before selecting any approach to margining. The foremost goal of initial margin is to cover potential future exposures, whether on a product- or portfolio-level basis. This is typically demonstrated through the coverage of losses achieving, at a minimum, the common 99% standard set out in the Principles for Financial Market Infrastructures (PFMIs) by the Committee on Payments and Market Infrastructures (CPMI) and International Organization of Securities Commissions (IOSCO) in April 2012. In addition to achieving coverage, the objective of a margining framework is predictability and stability.
Copyright Infopro Digital Limited. All rights reserved.
As outlined in our terms and conditions, https://www.infopro-digital.com/terms-and-conditions/subscriptions/ (point 2.4), printing is limited to a single copy.
If you would like to purchase additional rights please email info@risk.net
Copyright Infopro Digital Limited. All rights reserved.
You may share this content using our article tools. As outlined in our terms and conditions, https://www.infopro-digital.com/terms-and-conditions/subscriptions/ (clause 2.4), an Authorised User may only make one copy of the materials for their own personal use. You must also comply with the restrictions in clause 2.5.
If you would like to purchase additional rights please email info@risk.net