Journal of Credit Risk
ISSN:
1744-6619 (print)
1755-9723 (online)
Editor-in-chief: Linda Allen and Jens Hilscher
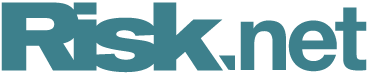
Incorporating small-sample defaults history in loss given default models
Need to know
- Constructing two regressions instead of one is the correct way to estimate LGD.
- For a low-default portfolio, applying two regressions can give results better than a single model.
- The inclusion of small samples in the LGD estimation requires ensemble modeling techniques.
Abstract
This paper proposes a methodology for estimating loss given default (LGD) that accounts for small default sample sizes. Regulatory guidelines for estimating LGD state that it is essential to take into account all observed defaults from the selected time period. In many portfolios, such as those for many emerging markets, the number of observed defaults is limited. Further, methodologies that exclude information from unresolved defaults produce biased estimates. We show that the distribution of LGD is bimodal, and we develop an estimation approach that uses a combination of logistic and linear regression to produce more reliable estimates. We implement our methodology for a sample of loans of a Polish bank, and demonstrate refinements to our modeling that produce the most precise final estimates of LGD.
Copyright Infopro Digital Limited. All rights reserved.
You may share this content using our article tools. Printing this content is for the sole use of the Authorised User (named subscriber), as outlined in our terms and conditions - https://www.infopro-insight.com/terms-conditions/insight-subscriptions/
If you would like to purchase additional rights please email info@risk.net
Copyright Infopro Digital Limited. All rights reserved.
You may share this content using our article tools. Copying this content is for the sole use of the Authorised User (named subscriber), as outlined in our terms and conditions - https://www.infopro-insight.com/terms-conditions/insight-subscriptions/
If you would like to purchase additional rights please email info@risk.net