
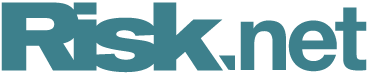
In fake data, quants see a fix for backtesting
Traditionally quants have learnt to pick data apart. Soon they might spend more time making it up
It wasn’t a surprise that videos of Tom Cruise playing golf and doing magic tricks should rack up millions of views on TikTok earlier this year. The real surprise was that the clips didn’t feature Tom Cruise at all. They were fakes.
The technology behind these ultra-realistic ‘deep fake’ videos, now common on social media platforms, has already found a home in finance where quants are using it to create parallel universes of data to test investment strategies.
Experts say this synthetic data
Only users who have a paid subscription or are part of a corporate subscription are able to print or copy content.
To access these options, along with all other subscription benefits, please contact info@risk.net or view our subscription options here: http://subscriptions.risk.net/subscribe
You are currently unable to print this content. Please contact info@risk.net to find out more.
You are currently unable to copy this content. Please contact info@risk.net to find out more.
Copyright Infopro Digital Limited. All rights reserved.
You may share this content using our article tools. Printing this content is for the sole use of the Authorised User (named subscriber), as outlined in our terms and conditions - https://www.infopro-insight.com/terms-conditions/insight-subscriptions/
If you would like to purchase additional rights please email info@risk.net
Copyright Infopro Digital Limited. All rights reserved.
You may share this content using our article tools. Copying this content is for the sole use of the Authorised User (named subscriber), as outlined in our terms and conditions - https://www.infopro-insight.com/terms-conditions/insight-subscriptions/
If you would like to purchase additional rights please email info@risk.net
More on Quant investing
The quants who kicked the hornets’ nest – to champion causality
A small but influential cadre says the multi-trillion-dollar factor investing industry is based on flawed science
‘Brace, brace’: quants say soft landing is unlikely
Investors should prepare for sticky inflation and volatile asset prices as central banks grapple with turning rates cycle
Trend following struggles to return to vogue
Macro outlook for trend appears to be favourable, but 2023’s performance flop gives would-be investors pause for thought
Can machine learning help predict recessions? Not really
Artificial intelligence models stumble on noisy data and lack of interpretability
How patchy liquidity is stymieing systematic credit
…and what investors like AllianceBernstein, Man Numeric and Acadian are doing about it
How Man Numeric found SVB red flags in credit data
Network analysis helps quant shop spot concentration and contagion risks
Is low vol crowded? That depends who you ask
Equity drawdowns have pushed more investors into low volatility strategies, raising fears of a build-up of risk
The quant investor harnessing the power of ants
Swarm Technology designs network of trading algorithms that mimics hive mind of insects
Most read
- Podcast: Olivier Daviaud on P&L attribution for options
- For a growing number of banks, synthetics are the real deal
- SG trader dismissals shine spotlight on intraday limit controls