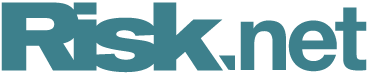
Model misfires raise questions over training data
Quants wrestle with how far into the past their machine learning models should peer
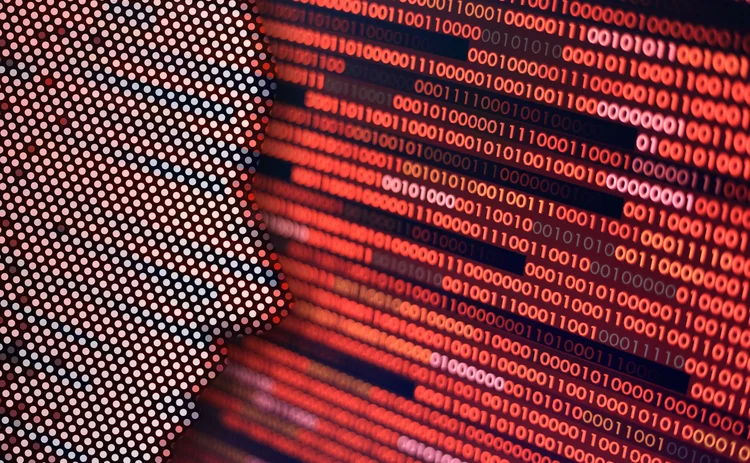
Wisdom accumulated over many decades is highly prized in most cultures. Less so for machine learning in investing, it would seem.
Algorithms that use long histories of data to build their understanding of markets flopped during the Covid-19 pandemic. Such models failed “pretty spectacularly” in the extreme events of 2020, says Michael Heldmann, head of multi-factor equity investing for North America at Allianz Global Investors, citing research conducted by the firm.
“They have been hammered
Only users who have a paid subscription or are part of a corporate subscription are able to print or copy content.
To access these options, along with all other subscription benefits, please contact info@risk.net or view our subscription options here: http://subscriptions.risk.net/subscribe
You are currently unable to print this content. Please contact info@risk.net to find out more.
You are currently unable to copy this content. Please contact info@risk.net to find out more.
Copyright Infopro Digital Limited. All rights reserved.
You may share this content using our article tools. Printing this content is for the sole use of the Authorised User (named subscriber), as outlined in our terms and conditions - https://www.infopro-insight.com/terms-conditions/insight-subscriptions/
If you would like to purchase additional rights please email info@risk.net
Copyright Infopro Digital Limited. All rights reserved.
You may share this content using our article tools. Copying this content is for the sole use of the Authorised User (named subscriber), as outlined in our terms and conditions - https://www.infopro-insight.com/terms-conditions/insight-subscriptions/
If you would like to purchase additional rights please email info@risk.net
More on Quant investing
Execs can game sentiment engines, but can they fool LLMs?
Quants are firing up large language models to cut through corporate blather
The quants who kicked the hornets’ nest – to champion causality
A small but influential cadre says the multi-trillion-dollar factor investing industry is based on flawed science
‘Brace, brace’: quants say soft landing is unlikely
Investors should prepare for sticky inflation and volatile asset prices as central banks grapple with turning rates cycle
Trend following struggles to return to vogue
Macro outlook for trend appears to be favourable, but 2023’s performance flop gives would-be investors pause for thought
Can machine learning help predict recessions? Not really
Artificial intelligence models stumble on noisy data and lack of interpretability
How patchy liquidity is stymieing systematic credit
…and what investors like AllianceBernstein, Man Numeric and Acadian are doing about it
How Man Numeric found SVB red flags in credit data
Network analysis helps quant shop spot concentration and contagion risks
Is low vol crowded? That depends who you ask
Equity drawdowns have pushed more investors into low volatility strategies, raising fears of a build-up of risk
Most read
- Breaking out of the cells: banks’ long goodbye to spreadsheets
- Too soon to say good riddance to banks’ public enemy number one
- Industry calls for major rethink of Basel III rules