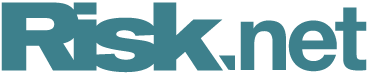
This article was paid for by a contributing third party.More Information.
Model risk management is evolving: regulation, volatility, machine learning and AI
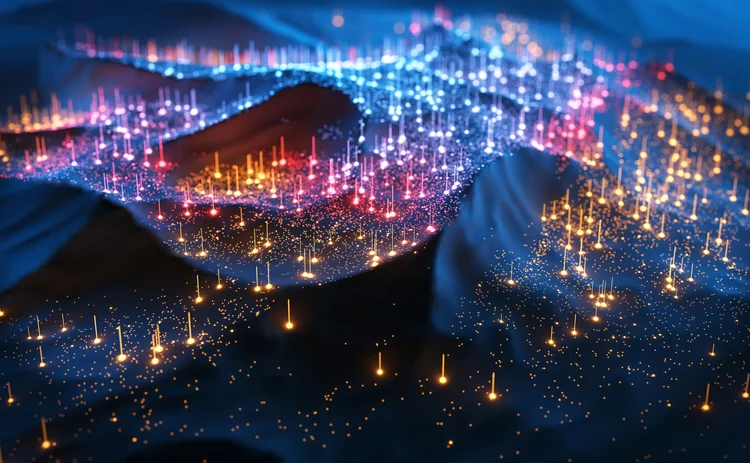
Emerging from the impact of the Covid-19 pandemic, the world is now dealing with geopolitical uncertainty, increased concerns over counterparty risk and rising interest rates, all of which present fresh challenges for model risk managers. Thomas Oliver, head of model validation at Quantifi, explores how the model risk management (MRM) landscape is changing in response to these challenges
Which model risks are regulators most concerned about in 2023?
With the high-profile failures of entities such as Archegos, FTX, Silicon Valley Bank and Credit Suisse, many regulators such as the UK’s Prudential Regulatory Authority (PRA) are pressing banks to ensure they have completed adequate assessments of their liquidity, credit and counterparty risks. This includes due diligence against fraud, and satisfactory credit risk methodologies for evaluation of entities with concentrated operating exposures, such as crypto assets, sector-specific concentrations or asset-liability mismatches. Longer‑term concerns raised by the US Federal Reserve and PRA have related to model estimation of climate change risks in long-maturity commitments, such as infrastructural or mortgage lending.
As the broad principles of MRM have been established for several years through the Fed’s SR11-07 guidance and the European Union’s Targeted Review of Internal Models, regulators now expect high visibility on a bank’s internal model risk – model inventory, review lifecycle, usage governance – alongside evidence of established control processes. However, regulators have been sympathetic to the additional risk management complexities faced by firms during the pandemic, and in the aftermath of the invasion of Ukraine, with many regulators extending timescales for full Basel III compliance – for example, to January 2025 for the UK and EU.
Lessons learned from the pandemic
The sudden and severe economic shocks from the pandemic created model risk challenges, because of the magnitude of behavioural and market changes, and the pace at which these changes occurred. Declines in oil consumption during lockdowns caused such logistical storage stresses that West Texan Intermediate (WTI) futures traded below zero.

Credit-scoring models also struggled to assess individuals and small businesses that had suffered a collapse in incomes but also were receiving unprecedented government support. In the UK, for example, despite a -9.7% contraction in GDP in 2020, fewer businesses went bankrupt in 2020 and 2021 than in the years preceding the pandemic.
With historical dynamics seldom reflecting recent conditions, it has been increasingly important to ensure that models have timely adjustments to calibration procedures and usage scopes. Institutions need to be nimble in monitoring rapid performance changes, and able to adjust model processes and usage patterns quickly, requiring good communication between model developers, validators and other stakeholders, and backup business logic established for handling periods of model degradation.
How is machine learning influencing MRM?
Machine learning has scope to transform multiple areas of MRM. As machine learning models enter operational usage, there are additional requirements to evidence model robustness and explain model decision-making. Complex machine learning models may need use of decomposition analysis with techniques such as local interpretable model-agnostic explanations, Shapley additive explanations or implementation of dedicated interpretability models. There may also need to be additional evidence of sufficient operational and implementation steps – firms’ machine learning operations – to ensure complicated models have reproduceable outcomes, timely recalibration and accurate alignment between methodology and production deployments. More sophisticated models, in areas such as natural language processing, may also have reliance on external models (transfer learning), such as GPT-4, with adaptions for specific tasks rather than training from in-house inception. This means that model management needs to understand the risk of biases or deficiencies even when, in some cases, there may not be visibility on the original training data or calibration code.
On the validation side, machine learning promises increasing support for validators to systematically benchmark models and performance to minimum expected thresholds, such as using multi-model automated machine learning checks, to ensure proposed models are making use of all potentially relevant data and identifying any possible enhancements.
If US regulators limit the role of internal models for credit risk measurement, what does this mean for MRM?
Regulators have multiple concerns around reliance on internal models; the risks from a complex model may be much harder to understand and mitigate than the known limitations of cruder, but more transparent, methodologies. For internal models to be adequately conservative, there needs to exist internal capacity within the bank to assess the model, and external regulatory capacity to ensure internal control processes are robust. The desire of revenue functions in banks to increase lending or take larger market positions creates the risk of incentives to hide favourable bias in a model’s complexity.
From an MRM perspective, a move towards simpler standardised models would simplify regulatory compliance. Regulators are, however, aware that forcing all banks to use common modelling frameworks would disincentivise innovation and reduce the diversity of opinions on valuation or risk management. This could lead to distortions of markets where pricing is driven by now-identical regulatory capital considerations. Prescription enforcement of common, simpler methodology could worsen the impact of systematic model errors if all participants, rather than a single bank, harness a common flawed model. US regulators are therefore weighing up the transparency benefits against the risk of introducing market distortions; if they conclude that the difficulty of assessing the conservatism of firm-specific internal credit models is intrinsically too high, then standardisation may be the best approach to ensuring adequate conservatism across all regulated entities.
How does adoption of FRTB impact MRM for market risk?
The Fundamental Review of the Trading Book (FRTB) is the part of Basel III that specifically focuses on estimations of market risk for banks’ trading portfolios. Basel lll already led to a material increase in banks’ buffers between 2011 and 2021.

FRTB permits internal model treatment of positions, only where there are liquid markets, to obtain realistic estimates of the historical volatility and correlations of such positions. The risk representations of positions need to capture a sufficient component of their actual market profit and loss, and show accurate prediction of historical losses through backtesting. In addition, the testing horizon now extends to all available historical data, and less liquid positions need to use larger change horizons. This significantly increases the amount of data that will need to be retained and the comprehensiveness of product treatment within market risk methodologies.
With these more standardised criteria, FRTB shifts discussion about sufficiency of representations that, historically, would be determined by each bank and each regulator to a more standardised format. Model risks still arise where there is market behavioural change, such that different risk factors become dominant or where position hedging means that a bank builds material exposure to risk factors that are less well modelled or omitted. Model validation can therefore be more focused on models’ robustness to market regime change, the quality of the underlying valuation models and the hedging behaviour for intended model usage.
Technology remains a key enabler for agile methodologies
Technology has always been at the core of trading and risk measurement: it is operationally critical to have computational-efficient approaches to price, obtain sensitivities and evaluate risks for all assets in portfolios. Implementation and regular upgrading of reliable analytics can create significant challenges for quantitative and technology departments. Institutions unable to produce and maintain such analytics economically themselves are looking to fintech providers such as Quantifi that specialise in delivering optimised model libraries, data science platforms and analytic frameworks through application programming interfaces and on-premise solutions. As well as direct usage for pricing and risk management, Quantifi can also support model validation activities. Download the related white paper from Quantifi, Model risk management: strengthening model governance, or learn how Quantifi can help your business.
Sponsored content
Copyright Infopro Digital Limited. All rights reserved.
You may share this content using our article tools. Printing this content is for the sole use of the Authorised User (named subscriber), as outlined in our terms and conditions - https://www.infopro-insight.com/terms-conditions/insight-subscriptions/
If you would like to purchase additional rights please email info@risk.net
Copyright Infopro Digital Limited. All rights reserved.
You may share this content using our article tools. Copying this content is for the sole use of the Authorised User (named subscriber), as outlined in our terms and conditions - https://www.infopro-insight.com/terms-conditions/insight-subscriptions/
If you would like to purchase additional rights please email info@risk.net