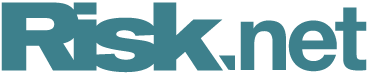
This article was paid for by a contributing third party.More Information.
The evolution of trading risk and the return to volatility
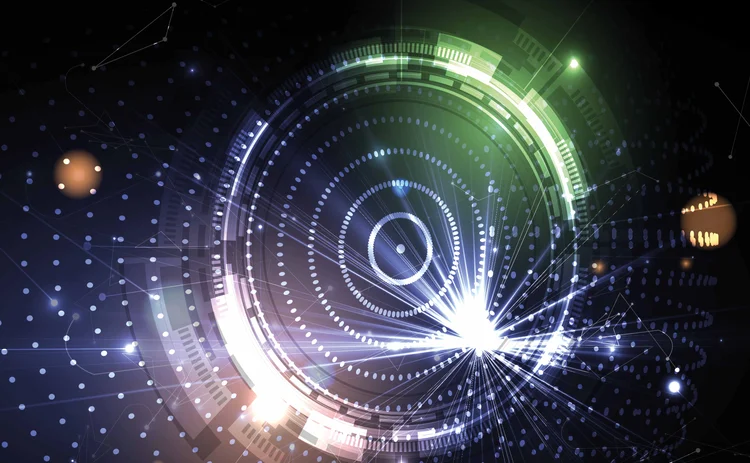
Risk managers are grappling with the complexities of today’s financial markets, tackling what seems to be a never-ending stream of crises, exacerbated by surging interest rates and widening credit spreads. In a recent Risk Live North America panel session sponsored by S&P Global Market Intelligence, panellists explored the impact of this return to volatility and the evolution of trading risk. This article examines the main themes arising from that discussion
The panel
- Mark Findlay, Managing director and global head of financial risk analytics, S&P Global Market Intelligence
- Joe Li, Managing director and global head of market risk, Citibank
- Ash Majid, Managing director and chief risk officer, SMBC Capital Markets and SMBC Nikko America
- Ji Qin, Head of market risk, HSBC
- Marc Vial, Head of global risk analytics, Mizuho Group
- Moderator: Sakshi Sharma, Commercial editor, Americas, Risk.net
Adapting to accelerated market change
Following a long period of stability, risk managers are rapidly and continually adapting to volatility as the new norm. In the wake of significant global upheavals driven by the Covid-19 pandemic and geopolitical tensions, there has been a marked shift in market and interest rate volatility. Recent crises – such as the liability-driven investment (LDI) crisis in the UK and the regional banking and yield shift crises in the US – are compounding challenges for trading risk.
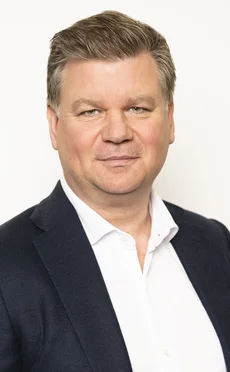
This volatility looks set to continue – and its scale and pace of change is proving particularly problematic. Mark Findlay, managing director and global head of financial risk analytics at S&P Global Market Intelligence, explained: “Everything is moving so much faster and in a shorter period of time. We have seen a massive volatility shift in the past 18 months to two years – that’s not going to go away.
“We’ve seen LDI squeezes in the UK, yield shifts in the US ... pandemics, global market shifts in commodities, and wars. It’s time to look at risk in a more prescient way to understand the stresses in the market.”
Looking back several years, risk managers had arguably become complacent. Market risk teams were more focused on the potential impact of negative rates on their models, with a general belief that interest rate shocks were not on the cards.
Marc Vial, head of global risk analytics at Mizuho Group, says perceptions have now shifted: “Risk managers have been reminded to look at both sides of risk; there is always an upside and a downside. They are now questioning what other risks they may be overlooking.”
Ji Qin, head of market risk at HSBC, agreed, adding that: “Now, more than ever, risk managers need to be aware of the potential for small ‘immaterial’ risks to blow up in a way they would not have done historically.” There was a strong sense among the panel that, given this return to volatility, the challenge lies in developing the right risk frameworks and tools to remain ahead of the game.
Data challenges
The return to volatility is highlighting data challenges within trading risk. From a process perspective, institutions need clear data controls and ownership. Having accurate, comprehensive and usable data is also vital. However, the quality and availability of data – particularly for newer and riskier asset classes – pose significant challenges.
“There’s been a push to generate alpha on the buy side, while the sell side is carrying [riskier assets] on its books,” said Findlay. “The data is often sketchy – there are a lot of gaps, so it has become a real challenge.”
Findlay also noted data concerns over the changing profile of trading books and the impact of private markets moving towards centre stage.
“If we look at the capital structure we’re all familiar with – in terms of debt-to-equity ratio – that spread has widened. Is that caused by private markets having more of a play?” he asked.
Another issue is the challenge of using historical data to make risk-based decisions. “Using data from the late 1980s for time-series analyses will result in different correlations than we see today,” said Ash Majid, managing director and chief risk officer at SMBC Capital Markets and SMBC Nikko America.
“Today’s scenario is the complete opposite, and we are seeing a lot of correlations breaking down from the norm.”
The panel stressed the importance of understanding how data impacts the quality of model outputs. “If you don’t understand the embedded assumptions or the margin of error, it may take you on the wrong path,” said Vial.
“Liquidity data is also an important – but difficult – challenge,” added Joe Li, managing director and global head of market risk at Citibank. “Institutions need to not just think about high-level or market liquidity metrics, they need to define position-level liquidity metrics.”
Technology and artificial intelligence (AI)
Significant advances in computational capabilities such as cloud computing, analytical tools and AI have shifted the bar since the financial crisis that began in 2007–08, playing a central role in assessing and quantifying trading risk.
Integration of these technologies drastically reduces the time it takes to run model calculations, automating data cleansing and enhancing data quality.
AI also offers significant opportunities for advanced risk modelling and scenario testing, which is key to navigating unchartered waters. It enables more refined scenario analyses, helping risk managers navigate an expanding range of variables and narrow a potentially infinite number of scenarios. It also frees time to focus on higher-value activities, such as analysing results and assessing the plausibility of scenarios.
The panel noted a range of AI use cases, such as scouring company reports for credit scoring, chatbots enabling the front office to check limits, and modelling new indexes. Findlay also highlighted AI’s potential to inform reverse stress-testing: “Being able to predict why a stress test is causing a particular impact on a portfolio, and being able to rerun that in an infinite loop of discovery is something AI can really help with.”
However, there was a note of caution around AI – particularly in terms of regulatory and governance challenges. There will be growing focus on oversight and understanding of AI models and backtest results.
The initial hype over developments such as ChatGPT is calming down as people realise that the quality of the data feeding AI is directly correlated to the quality of its outputs. “There are undoubtedly challenges with training AI models – particularly for riskier assets – based on the lack of stable data and the volatility seen in the past two years,” explained Findlay. “There is going to be more caution around it. The black-box approach regulators fear in understanding those models is something they’ll clamp down on even further.”
Majid also highlighted the discrepancy in AI adoption among financial institutions. “A lot of large banks are not comfortable with it. It will take a few more years, a few more use cases and for more governance to be built up around those tools before we see that full adoption,” he said.
Ultimately, AI is likely to lead to a fundamental change in the way analytics and technology are managed at large institutions, given its implications for data architecture and process.
Interconnected risks
The increasingly interconnected nature of risk – from market and liquidity to credit risk – means risk managers need a dynamic, integrated and holistic view, with consistency across different measures.
Findlay noted that the trend of risk moving out of silos will continue: “In the sell side, trading and sales are moving closer to risk in the middle office, and that same trend is being seen in the buy side. The more successful firms have risk processes that are much closer to front-office and investment processes, having the same datasets and tools they can leverage, with one informing the other to make smart choices.”
In summary
Against a backdrop of persistent volatility and ever-evolving challenges, being able to dynamically understand and quickly quantify current and future risk will be a key differentiator for risk managers. This new paradigm requires rapid, proactive approaches and the integration of advanced technologies and tools.
Risk managers can no longer afford to be complacent – instead, they require a holistic and comprehensive view of interconnected risks and a deep understanding of the data.
“On the buy side, yield changes on investment portfolios mean duration risk is top of mind. There are more callable features in the market now for the buy side. So having that analytics and modelling capability available is vital,” said Findlay. “You also have to balance those risks across different asset classes coming into play – areas such as private markets, structured products and off-balance sheet products where liquidity risk and cost will be a key factor. On the sell side, it’s about collateral, with more non-cash collateral being used in XVA agreements,” he added. “How do you manage those exposures over time, considering challenges such as geopolitical risk, foreign exchange risk and basis risk?”
Get instant XVA measures with zero implementation headaches
The XVA Pricer by S&P Global Market Intelligence provides deal-time XVA measures powered by a best-in-class analytics engine and market data. No data integration required.
Sponsored content
Copyright Infopro Digital Limited. All rights reserved.
You may share this content using our article tools. Printing this content is for the sole use of the Authorised User (named subscriber), as outlined in our terms and conditions - https://www.infopro-insight.com/terms-conditions/insight-subscriptions/
If you would like to purchase additional rights please email info@risk.net
Copyright Infopro Digital Limited. All rights reserved.
You may share this content using our article tools. Copying this content is for the sole use of the Authorised User (named subscriber), as outlined in our terms and conditions - https://www.infopro-insight.com/terms-conditions/insight-subscriptions/
If you would like to purchase additional rights please email info@risk.net