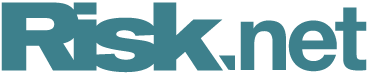
This article was paid for by a contributing third party.More Information.
Unlocking the power of model ops for risk management gains
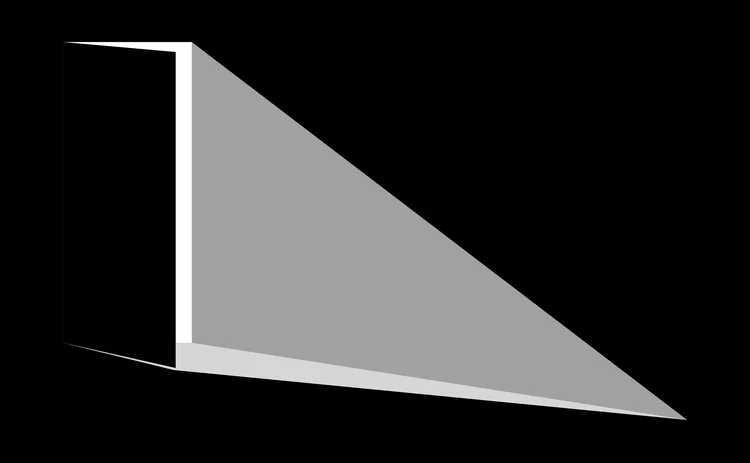
With banks coming under pressure to revisit risk models, many are now turning to model operations to bring much-needed improvements to every stage of the risk model lifecycle. A fundamental shift in the way risk models are developed, deployed, monitored and validated can yield various benefits, including higher transparency, lower capital requirements and the ability to govern thousands of models for business success
The panel
- Stephen Tonna, Senior banking solutions adviser, risk and quantitative solutions, Apac, SAS
- Terisa Roberts, Director, risk research and quantitative solutions, SAS
- Juyoung Lim, Apac model risk officer, Bank of America
- Anthony O’Connor, Senior lead, global model risk governance, HSBC
- Mohan Sharma, Head of operational and outsourcing risk, ICICI Bank
- Moderator: Catherine Law, Partner, Deloitte New Zealand
Many banks are now revisiting their model landscape in the face of growing regulatory requirements, the changing economic cycle and new technologies. However, the road from model development to deployment is often a long one beset with challenges and delays. As a result, financial institutions are turning to model operations to drive much-needed efficiencies. A departure from ‘business as usual’ at most banks, model ops provide a framework of best practice and model risk mitigation across the model lifecycle.
In a Risk.net webinar convened in May, panellists discussed the latest challenges for risk modelling at banks, looking at how risk model ops – a distinct branch of model ops – can increase the speed and efficiency of risk modelling, from development and validation through to deployment and ongoing monitoring.
Here we reveal five themes that emerged from the discussion.
Defining risk model ops
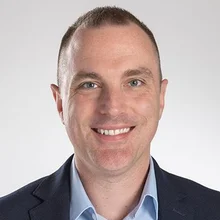
Stephen Tonna, senior banking solutions adviser, risk and quantitative solutions, Asia-Pacific (Apac) at SAS, described risk modelling as “a process of using quantitative methods that leverage, for example, analytics, algorithms and statistical machine learning techniques to identify and predict potential risks, while developing strategies to minimise their impact”. These methods enable firms to measure financial and non-financial risks, providing valuable insights and reducing uncertainties.
According to Tonna, the critical nature of risk models – many of which predict defaults or other potentially large financial risks, or ensure compliance with regulations such as Basel III/IV or Solvency II – means they need to be continuously monitored and updated to ensure ongoing performance, accuracy and reliability throughout their lifecycles.
Risk model ops therefore demand additional controls and considerations beyond those required for normal model ops. For example, documents must be stored and content must be clear and accurate to explain models to regulators and auditors. Traceability, visibility, auditability and segregation of duty are essential for meeting regulatory requirements and ensuring models remain effective in complying with regulations, while also understanding the potential loss of a portfolio.
Why banks need a model rethink
The panel identified several reasons banks should revisit their models:
- Most models were developed in a low interest rate environment and will therefore need an overhaul
- Regulatory expectations are tightening
- Emerging risks, such as climate change, require new modelling approaches
- Modellers need to explore whether machine learning and artificial intelligence (AI) would improve their models
- Following the Covid-19 pandemic, banks need to compete in a more agile way, with customers demanding faster services – especially around credit decisions.
With so much change required, banks also need to overhaul the operations and governance framework surrounding their models. “A fundamental shift is needed in the way models are developed, deployed, monitored and validated,” said Tonna.
Key model ops challenges and solutions
The panellists identified a host of challenges besetting model ops about which webinar delegates were also asked their opinions. Through an online poll, delegates were asked to pinpoint the biggest bottlenecks facing their organisations today in the risk model lifecycle.
The top response, with 52% of the vote, was “incomplete and incorrect data”. A further 22% of respondents said that “deploying risk models to production systems takes too long”, while 14% said there were “too many manual steps in model validation”. Some 12% voted for “lack of a centralised model repository”.
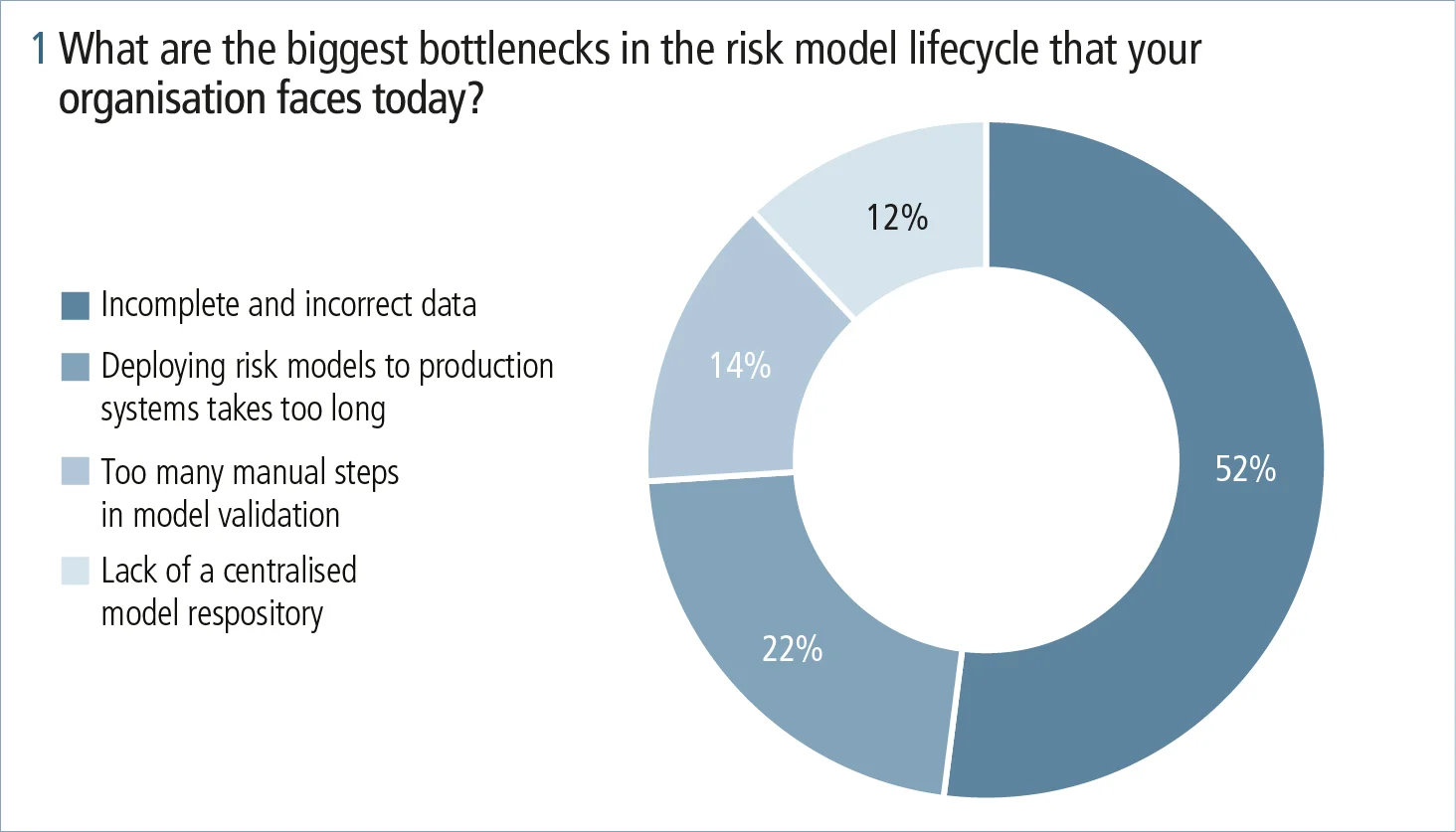
Other challenges identified by the panel included the increased complexity of models, third-party model risk and key person risk. Mitigation strategies were discussed for each of these risks.
For example, model complexity – while not necessarily a bad thing – requires some safeguarding. “We need to put in additional controls for more sophisticated models,” said Terisa Roberts, director, risk research and quantitative solutions at SAS.
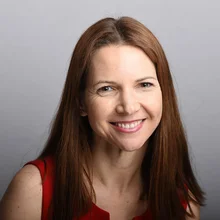
Similarly, third-party models were identified as worthy of additional attention, requiring the same scrutiny as a bank’s own models so that staff are not encouraged to buy third-party models instead of building their own. Mohan Sharma, head of operational and outsourcing risk at ICICI Bank, noted that, if documentation for third-party models falls below the bank’s standards – as is often the case – banks will need to look at whether the model can be used in certain cases and not others.
Key person risk – the problem of firms having only a few individuals with in-depth model knowledge – is ameliorated by the current scarcity of analytics talent, noted Roberts. She recommended lowering the barrier of entry for model development by leveraging low-code and no-code interfaces, for example. That way a wider range of personnel can be involved and models can be built quickly. “It may not apply to regulatory approved models, but may be an effective way to develop more models for auxiliary functions,” she stressed. Another option is to automate parts of the model lifecycle, such as feature selection, backtesting and performance monitoring, to help alleviate this issue, Roberts added.
Best practices in model ops
Panellists identified a range of best practices firms should engage in across model development and management. They recommended identifying and defining models so they are differentiated from, for example, a standard calculator or statistical averaging tool. They also advocated creating a central model inventory, where all models are recorded and stored alongside their documentation.
Documentation must be clear and accurate to explain the models to regulators and auditors, and should include performance guidelines. “Would you know if the model has gone wrong?” asked Sharma.
There must be full traceability of data to ensure every input is accurate and meets data governance standards. Data privacy is a key consideration and needs written policies, especially if third-party models are used.
Good communication links need to be set up across departments. “That means collaboration between risk, modellers, data scientists, practitioners and business stakeholders,” said Juyoung Lim, Apac model risk officer at Bank of America.
Panellists also recommended channelling the greatest time and effort into the most critical models.
Future innovation
As the discussion turned to expectations for future developments in model ops, there was wide agreement that automation is set to increase throughout the model lifecycle. Areas ripe for automation were seen as model validation – especially around the reporting process – and periodic model monitoring.
Tonna said there should be a reduction in manual coding going forward. Manually recoding a model into a particular production system is time-consuming and error-prone, he noted.
AI is set to be used more widely. “AI methods are developing at ground-breaking speed and there are significant operational efficiencies to gain,” said Roberts. Sharma suggested that AI can be especially useful for wading through reams of proxy data, in order to come up with more accurate probabilities around customer credit and behaviour, and balance sheet impact. However, extra consideration needs to be given to AI operations, especially around security, but also to guard against bias and to ensure the model’s fairness, he noted.
In summary, the panel cautioned that there is a lot more to learn around ethical principles and best practices in data collection, analysis and modelling as the industry moves towards a more standardised approach.
Learn more
View the webinar, Unlock the power of model ops: orchestrating your success in risk management
Discover more about SAS model ops best practice
Sponsored content
Copyright Infopro Digital Limited. All rights reserved.
You may share this content using our article tools. Printing this content is for the sole use of the Authorised User (named subscriber), as outlined in our terms and conditions - https://www.infopro-insight.com/terms-conditions/insight-subscriptions/
If you would like to purchase additional rights please email info@risk.net
Copyright Infopro Digital Limited. All rights reserved.
You may share this content using our article tools. Copying this content is for the sole use of the Authorised User (named subscriber), as outlined in our terms and conditions - https://www.infopro-insight.com/terms-conditions/insight-subscriptions/
If you would like to purchase additional rights please email info@risk.net