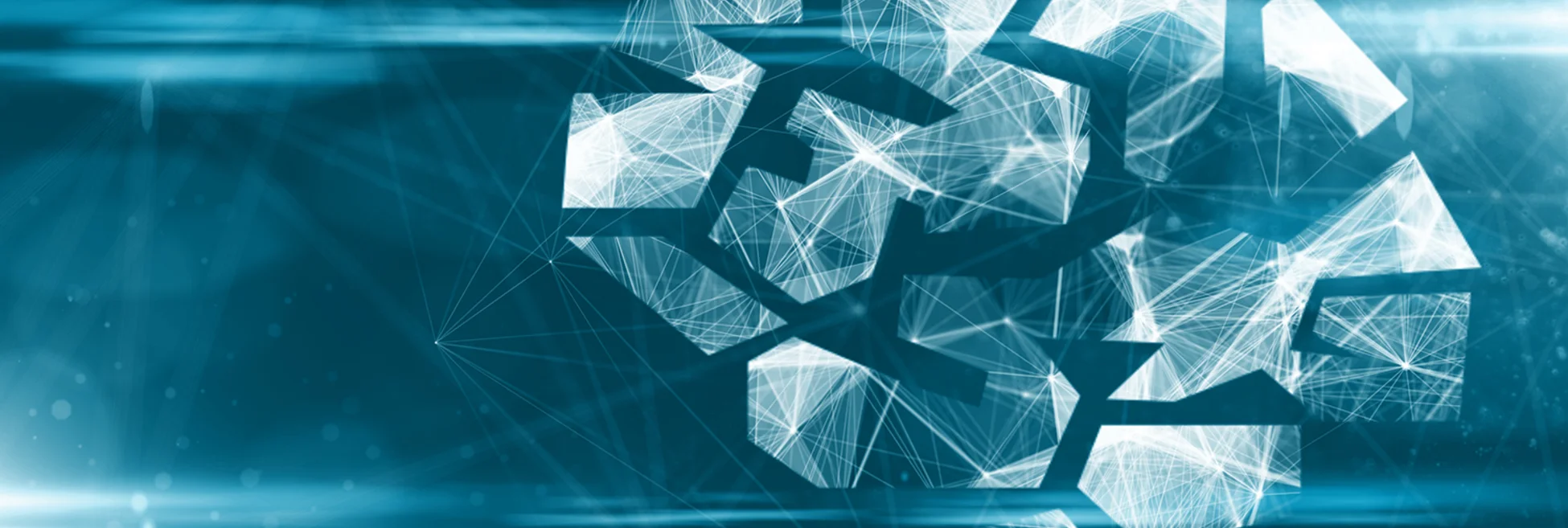
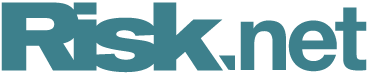
Differential machine learning: the shape of things to come
A derivative pricing approximation method using neural networks and AAD speeds up calculations by orders of magnitude
CLICK HERE TO DOWNLOAD THE PDF
Brian Huge and Antoine Savine combine automatic adjoint differentiation with modern machine learning. In addition, they introduce general machinery for training fast, accurate pricing and risk approximations, applicable to arbitrary transactions or trading books, and arbitrary stochastic models, effectively resolving the computational bottlenecks of derivatives risk reports and regulations
Pricing approximation has proved tremendously useful with advanced stochastic models. Many models do not allow fast, closed-form pricing and compute prices with slower numerical methods. Approximate analytics, such as the SABR formula (Hagan et al 2002), provide fast pricing in return for some degree of approximation, making it possible to practically apply sophisticated models on trading desks. Although researchers traditionally derived pricing approximations by hand, automated approximations inspired by machine learning (ML) have emerged in recent years (Horvath et al 2019; McGhee 2018; Ferguson & Green 2018); here, machines learn to encode fast pricing approximations from examples produced by numerical methods.
This article creates a general pricing and risk approximation factory, applicable to arbitrary derivatives instruments and stochastic models, where the simulation of the training set and the learning process are fast and reliable enough for online implementation, in real time, as part of a risk computation. Applications include custom risk reports, backtesting and regulations such as XVA, CCR, FRTB and Simm-MVA.11 1 XVA: counterparty value adjustment (CVA) and friends. CCR: counterparty credit risk. FRTB: Fundamental Review of the Trading Book. Simm-MVA: standardised initial margin model-margin valuation adjustment.
We follow the classic ideas of Longstaff & Schwartz (2001) and Carriere (1996) and train machines with datasets of simulated cashflows. We apply modern ML in place of classic regression, along the lines of Lapeyre & Lelong (2019), for example.
The main breakthrough here is that we are training ML models using not only simulated cashflows but also the differentials of cashflows with respect to initial state variables, also known as pathwise differentials. Automatic adjoint differentiation (AAD) has provided the derivatives industry with differentials that are computed behind the scenes, automatically, accurately to machine precision, and very efficiently, for a computation cost not much higher than that of cashflow simulation (see Giles & Glasserman 2006). The present article discusses how to train ML models with pathwise differentials as well as why this makes a dramatic difference in pricing and risk approximation quality. Our numerical examples illustrate the capability of differential ML to reliably train ML models on small datasets simulated in real time.
A more complete write-up is available as a preprint on arXiv (Huge & Savine 2020). A TensorFlow implementation is available on GitHub,22 2 See https://github.com/differential-machine-learning/notebooks. along with the reproduction of some numerical examples from this article as well as practical implementation details not covered in the text. We have also posted appendixes with some important additions, including mathematical justifications and proofs, and extensions to other ML frameworks, such as regression (where differentials provide effective regularisation) and principal component analysis (where differentials allow the extraction of the main risk factors of a transaction or a trading book). The questions of asymptotic control and convergence guarantee, critical for reliable deployment in production, are also addressed in these appendixes.
Training pricing approximations on simulated datasets
Machines learn pricing approximations from training sets of examples , . The training inputs are the initial state vectors in dimension , and they are often in the hundreds or thousands. The training labels are final payouts, defined as the discounted sum of all the cashflows in the instrument or trading book, simulated with an implementation of the stochastic model, with initial condition . Hence, , where is a Monte Carlo (MC) implementation under the pricing measure, and are the random numbers for path number . Therefore, are independent, unbiased (but noisy) estimates of the correct pricing function evaluated in , which justifies the practice of training pricing approximations on simulated cashflows.
The computation of each training example takes one MC path; hence, the computational cost to simulate the entire training set is similar to one pricing by MC. By contrast, training sets of ground-truth price labels require numerical methods for each training example, so the production of the dataset is too slow for online application.
The purpose of the training set is to learn an approximation as close as possible to the correct pricing function . One definition of the conditional expectation is the function of closest to in , the distance between and being estimated by the mean squared error (MSE) between the predictions and labels . It follows that approximations trained by minimisation of the MSE converge near the correct pricing functions in the limit of an infinite training set.33 3 How ‘near’ depends on two things. First is the capacity of the approximation, that is, the size of the subspace of functions it is capable of representing. Neural networks are universal approximators (Hornik et al 1989), and deep neural networks, in particular, are notoriously high capacity approximators (Dixon 2019); hence, they are well suited to this context. Second is the ability of the training algorithm to find the global minimum of the MSE. This is critical for unsupervised online operation and is addressed in appendix 4 online.
Overcoming limitations with differentials
Longstaff & Schwartz (2001) and Carriere (1996) recommend linear approximators. However, recent literature has largely illustrated the superiority of modern ML models such as deep neural networks, which are high capacity models, resilient in high dimension. Nevertheless, we found that the performance of modern ML remains insufficient in the context of complex transactions and trading books, where a vast number of training examples (often in the hundreds of thousands or millions) is necessary to learn accurate approximations, so the dataset cannot be simulated in real time. Our conclusion is consistent with the findings of Horvath et al (2019), Ferguson & Green (2018) and McGhee (2018), whose training consumes vast datasets and happens offline. High capacity ML models are prone to overfitting and require large training sets, even with regularisation.
We also observed that risk sensitivities converge considerably more slowly than values and often remain blatantly wrong, even with hundreds of thousands of training examples. Risk sensitivities are defined as the gradients of the (true) pricing function , and they are estimated as the gradients of the approximation . Unfortunately, as is well known in numerical analysis, the derivatives of a good approximation are not always a good approximation of the derivatives.
The challenge addressed here is therefore to learn accurate approximations of pricing and risk functions, and to overcome overfitting, with training sets of limited size simulated in realistic time. Our main proposition is to augment training sets with pathwise differentials. Recall that training labels are payouts simulated with training inputs as initial conditions: . Pathwise differentials are defined as the gradients of payouts with respect to initial state:
Those differentials, denoted , are defined path by path, hence the name pathwise differentials. They are also the differentials of training labels with respect to training inputs. We sometimes call them differential labels.
The most effective way to compute pathwise differentials is AAD. We do not cover AAD in this article, and refer instead to the founding article Giles & Glasserman (2006) and the vast amount of literature that has followed. Pathwise differentials carry more information than is typically used, eg, by averaging for risk reports. They tell us how the initial state affects cashflows in many different scenarios. We can leverage this information in many ways. The algorithms in what follows learn high-quality pricing functions precisely from differential labels. Differentials make a spectacular difference in approximation performance, particularly with smaller datasets. Differentiation by AAD or bumping requires cashflow smoothing to obtain well-defined pathwise derivatives.
Differential machine learning
Notation
To avoid unnecessarily heavy notation, we work in the context of feedforward neural networks, but the findings carry over to other ML models in a straightforward fashion. The mathematical structure of feedforward networks greatly simplifies the exposition. Besides, neural networks are well suited to pricing approximation, due to their high capacity and resilience in high dimension, as previously noted. Finally, trained neural networks are capable of very efficient evaluation and differentiation. Neural nets predict values and derivatives with almost analytic speed, making them suitable for use on complex risk reports, backtesting and regulatory capital.
Let us briefly recall the evaluation and differentiation of feedforward networks to establish the necessary notation for what follows.
Feedforward equations
Define the input (row) vector and the predicted value . For every layer in the network, define a scalar ‘activation’ function , applied element-wise over vectors or matrices. We denote by , the weights and biases of layer .
The network is defined by its feedforward equations:
(1) |
where is the row vector containing the pre-activation values, also called units or neurons, in layer .
Backpropagation
Feedforward networks are efficiently differentiated by backpropagation, which is generally applied to compute the derivatives of some cost function with respect to the weights and biases of the network. For now, we are not interested in those differentials but in the differentials of the predicted value with respect to the inputs . Recall that inputs are initial states and predictions are approximate prices; hence, these differentials predict risk sensitivities (Greeks). Backpropagation of (1) is of the form:
(2) |
with the adjoint notation , and is the element-wise (Hadamard) product.
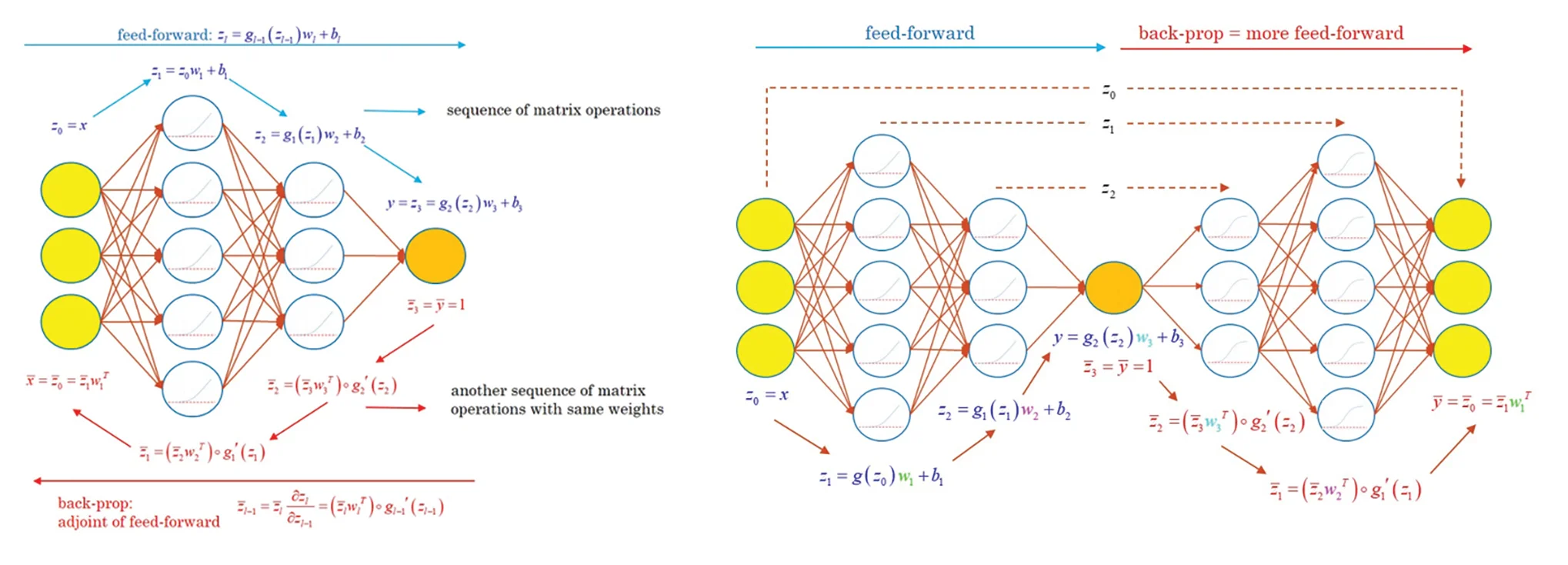
Click here to enlarge the image
Notice the similarity between (1) and (2). Backpropagation defines a second feedforward network with inputs , , and output that share weights with the first network, where the units in the second network are the adjoints of the corresponding units in the original network. This realisation leads to a combined twin network for the prediction of values and risks.
Figure 1 illustrates a feedforward network with and , , , , together with backpropagation (left). It also shows how the forward and reverse passes are unrolled in a twin network (right).
Twin networks
We can combine feedforward (1) and backpropagation (2) equations into a single network representation, or twin network, corresponding to the computation of a prediction (approximate price) together with its differentials with respect to inputs (approximate risk sensitivities).
The first half of the twin network (see figure 1, right) is the original network, traversed with feedforward induction to predict a value. The second half is computed with the backpropagation equations to predict risk sensitivities. It is the mirror image of the first half, with shared connection weights.
A mathematical description of the twin network is simply obtained by concatenation of (1) and (2). Evaluating the twin network returns a predicted value and its differentials with respect to the inputs . The combined computation evaluates a feedforward network of twice the initial depth. Like feedforward induction, backpropagation computes a sequence of matrices by vector products. The twin network, therefore, predicts prices and risk sensitivities with twice the computational complexity of value prediction alone, irrespective of the number of Greeks. Hence, a trained twin net approximates prices and risk sensitivities with respect to potentially many states in a particularly efficient manner.
Note from (2) that the units of the second half are activated with the differentials of the original activations . To backpropagate through the twin network, we need continuous activation throughout. Hence, the initial activation must be , ruling out, for example, ReLU.
A TensorFlow implementation of the twin network is available on GitHub44 4 See https://github.com/differential-machine-learning/notebooks/blob/master/DifferentialML.ipynb. along with a detailed discussion of the practical implementation details, which we skip here. That notebook also implements the training of the twin network with a dataset augmented with differential labels, which we will discuss next.
Training with differential labels
The purpose of the twin network is to estimate the correct pricing function by an approximate function . It learns optimal weights and biases from an augmented training set , where are the differential labels.
Here, we describe the mechanics of differential training and discuss its effectiveness. As is customary with ML, we stack training data in matrices, with examples in rows and units in columns:
Note that (1) and (2) apply to matrices or row vectors identically. Hence, the evaluation of the twin network computes the matrices:
respectively in the first and second half of its structure. Training consists in finding weights and biases minimising some cost function :
Classic training with payouts alone
Recall classic deep learning. We have seen that the approximation obtained by global minimisation of the MSE converges to the correct pricing function (modulo finite capacity bias), hence:
The second half of the twin network does not affect cost; hence, training is performed by backpropagation through the standard feedforward network. A conventional deep learning algorithm, the practical details of which are covered in the demonstration notebook, trains the network by minimising the MSE.
Differential training with differentials alone
Let us change gears and train with pathwise differentials instead of payouts , by minimisation of the MSE (denoted ) between the differential labels (pathwise differentials) and the predicted differentials (estimated risk sensitivities):
Here, we must evaluate the twin network in full to compute , effectively doubling the cost of training. Gradient-based methods minimise by backpropagation through the twin network, effectively accumulating second-order differentials in its second half. A deep learning framework such as TensorFlow performs this computation seamlessly. As we have seen, the second half of the twin network may represent backpropagation; in the end, this is just another sequence of matrix operations, easily differentiated by another round of backpropagation, carried out silently and behind the scenes. The implementation in the demonstration notebook is identical to training with payouts, save for the definition of the cost function. TensorFlow automatically invokes the necessary operations, evaluating the feedforward network when minimising and the twin network when minimising .
In practice, we must also assign appropriate weights to the costs of wrong differentials in the definition of the , as covered in the implementation notebook and discussed on Github.
Let us now discuss what it means to train approximations by minimising the between pathwise differentials and predicted risks . Given appropriate smoothing, expectation and differentiation commute so the (true) risk sensitivities are expectations of pathwise differentials:
It follows that pathwise differentials are unbiased estimates of risk sensitivities, and approximations trained by minimising the converge (modulo finite capacity bias) to a function with correct differentials; hence, the right pricing function, modulo an additive constant.
Therefore, we can choose to train by minimisation of value or derivative errors and converge near the correct pricing function all the same. This consideration is, however, an asymptotic one. Training with differentials converges near the same approximation, but it converges much faster, allowing us to train accurate approximations with much smaller datasets, as we will see in the numerical examples. This is because of the following.
- •
The effective size of the dataset is much larger: with training examples, we have differentials ( being the dimension of the inputs ). With AAD, we effectively simulate a much larger dataset for a minimal additional cost, especially in high dimension (where classical training struggles most).
- •
The neural nets pick up the shape of the pricing function, learning from slopes rather than points, which results in a much more stable and potent education, even with few examples.
- •
The neural approximation learns to produce correct Greeks by construction, not only correct values. By learning the correct shape, the ML approximation also correctly orders values in different scenarios, which is critical in applications such as value-at-risk and expected loss, including for FRTB.
- •
Differentials act as an effective, bias-free regularisation, as we will see next.
Differential training with everything
The best numerical results are obtained by combining values and derivatives errors in the cost function:
which is implemented in the demonstration notebook with the two previous strategies as particular cases. Note the similarity with classic regularisation of the form penalty. Ridge (Tikhonov) and Lasso regularisations impose a penalty for large weights (in and metrics, respectively), effectively preventing overfitting in small datasets by stopping attempts to fit noisy labels. In return, classic regularisation reduces the effective capacity of the model and introduces a bias, along with a strong dependency on the hyperparameter . This hyperparameter controls regularisation strength and tunes the vastly documented bias-variance trade-off. If one sets too high, their trained approximation ends up as a horizontal line.
Differential training also stops attempts to fit noisy labels, with a penalty for wrong differentials. It is, therefore, a form of regularisation, but a very different kind. It does not introduce bias, since we have seen that training on differentials alone converges to the correct approximation too. This breed of regularisation comes without bias-variance trade-off. It reduces variance for free. Increasing hardly affects the results in practice.
Differential regularisation is more similar to data augmentation in computer vision, which is, in turn, a more powerful form of regularisation. Differentials are additional training data. Like data augmentation, differential regularisation reduces variance by increasing the size of the dataset for little cost. Differentials are new data of a different type, computed on the same sample paths as existing data, but it reduces variance all the same, and without the introduction of a bias.
Numerical results
Let us now review some numerical results and compare the performance of differential and conventional ML. We picked three examples from relevant textbooks and real-world situations in which neural networks learned pricing and risk approximations from small datasets.
We kept the neural architecture constant in all the examples, with four hidden layers of 20 softplus-activated units. We trained neural networks on mini-batches of normalised data using the Adam optimiser and a one-cycle learning rate schedule. The demonstration notebook and appendixes discuss all the details. A differential training set takes two to five times longer to simulate with AAD, and it takes twice as long to train twin nets than to train standard ones. In return, we are going to see that differential ML performs up to a thousandfold better on small datasets.
Basket options
The first (textbook) example is a basket option in a correlated Bachelier model for seven assets:
where and . The task is to learn the pricing function of a one-year call option on a basket, with strike 110 (we normalised asset prices at 100, without loss of generality, and basket weights sum to 1). The basket price is also Gaussian in this model; hence, Bachelier’s formula gives the correct price. This example is also of particular interest because, although the input space is seven dimensional, we know from maths that actual pricing is one dimensional. Can the network learn this property from data?
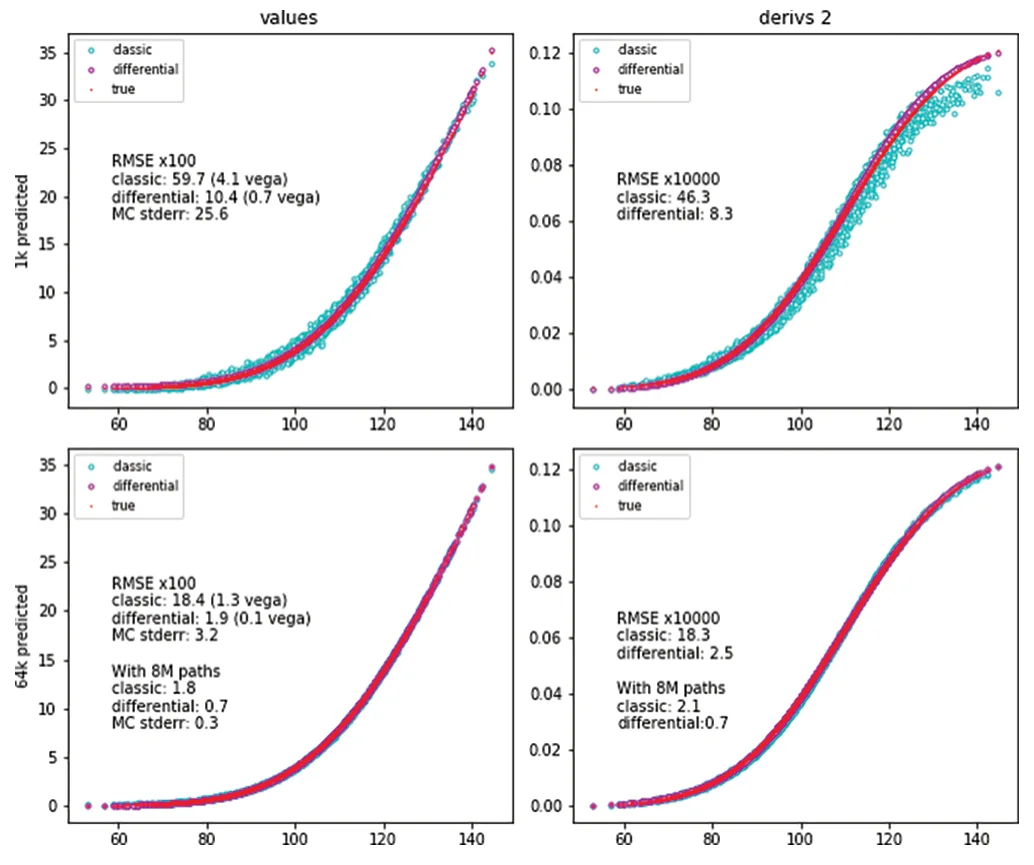
We trained neural networks and predicted values and derivatives in 1,024 independent test scenarios (see figure 2), with initial basket values on the horizontal axis and option prices/deltas on the vertical axis (we show one of the seven derivatives). These are compared with the correct results computed with Bachelier’s formula. We also trained networks on 1,024 (1k) and 65,536 (64k) paths, with cross-validation and early stopping. The twin network with 1k examples performed better for values than the classical net with 64k examples, and a lot better for derivatives. In particular, it learned that the option price and deltas are a fixed function of the basket, as evidenced by the thinness of the approximation curve. The classical network did not learn this property well, even with 64k examples. It overfitted the training data and predicted different values or deltas for various scenarios on the seven assets with virtually identical baskets.
We also compared test errors with standard MC errors (also with 1k and 64k paths). The main point of pricing approximation is to avoid nested simulations with similar accuracy. We see that the error of the twin network is, indeed, close to MC; classical deep learning error is an order of magnitude larger. Finally, we trained with eight million samples and verified that both networks converge to similarly low errors (not zero, due to finite capacity), while the MC error does converge to zero. The twin network gets there hundreds of times faster.
Worst-of autocallables
Our second example is an exotic instrument, a four-underlying version of the popular worst-of autocallable trade, in a more complicated model, a collection of four correlated local volatility models à la Dupire:
where . The example is relevant not only due to popularity, but also because of the tendency of path dependence, barriers or massive final digitals, which are notoriously hard for numerical algorithms. Smoothing was applied so the pathwise differentials are well defined.
We trained the classical network on 32k samples with cross-validation and early stopping, and the twin network on 8k paths with AAD pathwise derivatives. We generated both sets in around half a second using Superfly, Danske Bank’s proprietary derivatives pricing and risk management system.
In figure 3 we show the results for the value on the leftmost chart and the delta with respect to the second underlying in the middle chart for 128 independent examples, with correct numbers on the horizontal axis. We did not have a closed-form solution for reference; instead, we computed ‘correct’ numbers with nested MC simulations. The predicted prices and deltas are displayed on the vertical axis. Performance is measured by distance to the 45 line.
The twin network with only 8k pieces of training data produces a virtually perfect approximation for prices and a decent approximation for deltas. The classical net also approximates values correctly with 64k paths, although not in a straight line; this may cause problems in applications where correct ordering is critical, such as expected loss or FRTB. Its deltas are essentially random, which disqualifies it from the approximation of risk, eg, from Simm-MVA. Once again, the test error with the differential net is of a similar order to that of a nested MC. The root MSE for the classical net, with four times the training size, is three times larger for values and an order of magnitude larger for differentials.
Derivatives trading books
For our final example, we picked a real netting set from Danske Bank’s portfolio, including single and cross-currency swaps and swaptions in 10 different currencies, eligible for XVA, CCR or other regulated capital computations. We simulated paths in Danske Bank’s model of everything (‘the beast’), with interest rates simulated using four-factor term structure models correlated with both one another and forex rates.
This example is representative of how we want to apply twin nets in the real world. It is also a stress test for neural networks. The Markov dimension of the four-factor non-Gaussian Cheyette model is 16 per currency: this amounts to 160 inputs, 169 including forexes, and over 1k with all the path dependencies. Of course, the value effectively only depends on a small number of factors, which is something a neural net is supposed to identify. In reality, the extraction of risk factors is considerably more effective in the presence of differential labels (see appendix 2 online).
The rightmost chart of figure 3 shows the values predicted by a twin network trained on 8k samples with AAD pathwise derivatives being compared with a vanilla network trained on 64k paths. Performance improvement is evident. The differential net produces a virtually perfect approximation. As with the previous example, the vertical axis displays the predicted values for an independent set of 128 samples, with correct values, obtained by nested MC, on the horizontal axis. The entire training process for the twin network (on an entry-level GPU), including the generation of the 8k examples (on a multi-threaded CPU), took a few seconds on a standard workstation.
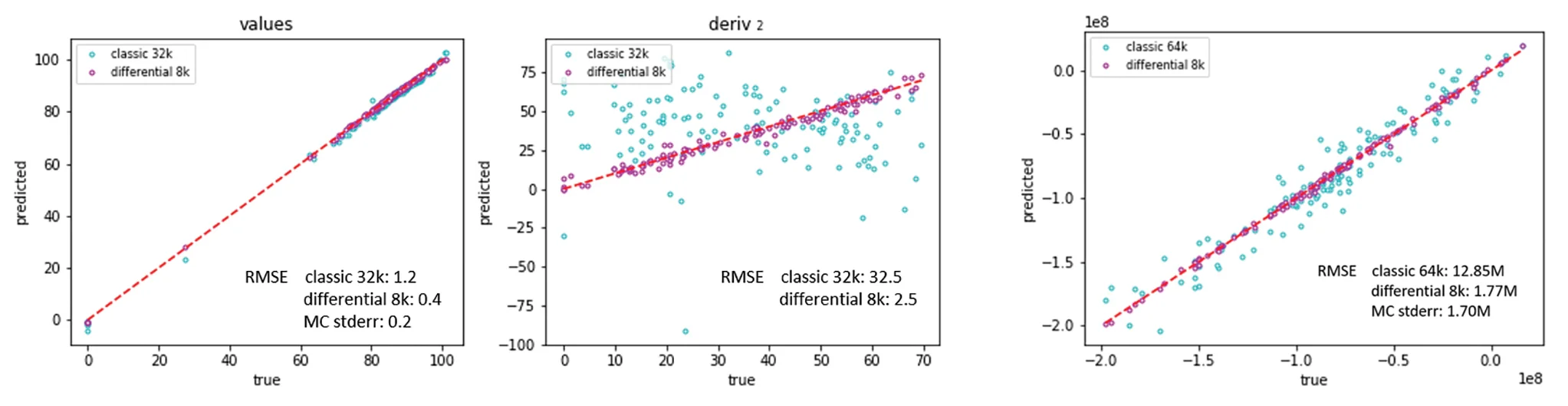
We also verified that the differential approximation error is, again, similar to the nested MC error, while the classical deep learning error is an order of magnitude larger.
Extensions
We have presented algorithms in the context of single value prediction to avoid confusion and too much notation. To conclude, we discuss two advanced extensions allowing the network to predict multiple values and higher-order derivatives simultaneously.
Multiple outputs
Horvath et al (2019) predict call prices of multiple strikes and expiries in a single network, exploiting correlation and shared factors, and encouraging the network to learn global features like no-arbitrage conditions. We can combine our approach with this idea by an extension of the twin network to compute multiple predictions, meaning and . The adjoints are no longer well defined as vectors. So, we define them as directional differentials with respect to some specified linear combination of the outputs , where has the coordinates of the desired direction in a row vector:
Given a direction , all the previous equations apply identically, except that the boundary condition for in the backpropagation equations is no longer 1 but rather the row vector . For example, means that adjoints are defined as derivatives of the first output . We can repeat this for to compute the derivatives of all the outputs with respect to all the inputs , ie, the Jacobian matrix. Written in matrix terms, the boundary is the identity matrix , and the backpropagation equations are written as follows:
where . In particular, is (indeed) the Jacobian matrix . To compute a full Jacobian, the theoretical order of calculations is times the vanilla network. However, the implementation of the multiple backpropagation in the matrix form above on a system like TensorFlow automatically benefits from CPU or GPU parallelism. Therefore, the additional computation complexity will be experienced as sublinear.
Higher-order derivatives
The twin network can also predict higher-order derivatives. For simplicity, we revert to the single prediction case where . The twin network predicts as a function of the input . The neural network, however, does not know anything about derivatives. It just computes numbers by a sequence of equations. Hence, we might as well consider the prediction of differentials as multiple outputs.
As previously, in what is now considered a multiple prediction network, we can compute the adjoints of the outputs in the twin network. These are now the adjoints of the adjoints:
in other words, the Hessian matrix of the value prediction . The original activation functions must be for this computation. The computation of the full Hessian is of order times the original network. These additional calculations generate a lot more data, one value, derivatives and second-order derivatives for the cost of times the value prediction alone. In a parallel system like TensorFlow, the experience also remains sublinear. We can extend this argument to arbitrary order , with the only restriction that the (original) activation functions are .
Conclusion
Throughout our analysis, we have seen that learning the correct shape from pathwise differentials makes a critical difference in the performance of regression models, including neural networks, in computational tasks such as the pricing and risk approximation of complex derivatives trading books. The unreasonable effectiveness of what we call differential ML allows us to reliably train accurate ML models on a small number of simulated examples in real time so they are suitable for online learning. Differential networks apply to real-world problems, including regulations and risk reports with multiple scenarios. Twin networks predict prices and Greeks with almost analytic speed, and their empirical test errors remain of comparable magnitude to those of nested MCs even though nested MCs are orders of magnitude slower to calculate.
Differential training also appears to stabilise the calibration of neural networks. We consistently observed a greatly improved resilience to hyperparameters such as the network architecture, the seeding of weights or the learning-rate schedule. Explaining why is a topic for further research.
Differential machine learning is similar to data augmentation in computer vision, which creates multiple labelled images out of a single image through cropping, zooming, rotating or recolouring. In addition to extending the training set for negligible cost, data augmentation encourages the ML model to learn important invariances. Data augmentation has been immensely successful in computer vision applications. Similarly, derivatives labels not only increase the amount of information in the training set, but also teach the model the shape of the pricing function.
Brian Huge and Antoine Savine are quantitative researchers with Superfly Analytics at Danske Bank.
Email: brian.huge@danskebank.dk,
antoine.savine@danskebank.dk.
References
- Carriere JF, 1996
Valuation of the early-exercise price for options using simulations and nonparametric regression
Insurance: Mathematics and Economics 19(1), pages 19–30 - Dixon M, 2019
A mathematical argument in support of deep learning
Available at https://github.com/differential-machine-learning/appendices/blob/master/dixon.pdf - Ferguson R and AP Green, 2018
Deeply learning derivatives
Preprint, arXiv:1809.02233 - Giles M and P Glasserman, 2006
Smoking adjoints: fast evaluation of Greeks in Monte Carlo calculations
Risk January, pages 92–96 - Hagan PS, D Kumar, AS Lesniewski and DE Woodward, 2002
Managing smile risk
Wilmott Magazine 1, pages 84–108 - Hornik K, M Stinchcombe and H White, 1989
Multilayer feedforward networks are universal approximators
Neural Networks 2(5), pages 359–366 - Horvath B, A Muguruza and M Tomas, 2019
Deep learning volatility
Preprint, available at https://papers.ssrn.com/sol3/papers.cfm?abstract_id=3322085 - Huge BN and A Savine, 2020
Differential machine learning
Preprint, available at https://papers.ssrn.com/sol3/papers.cfm?abstract_id=3591734 - Lapeyre B and J Lelong, 2019
Neural network regression for Bermudan option pricing
Preprint, available at https://arxiv.org/pdf/1907.06474.pdf - Longstaff FA and ES Schwartz, 2001
Valuing American options by simulation: a simple least-square approach
Review of Financial Studies 14(1), pages 113–147 - McGhee WA, 2018
An artificial neural network representation of the SABR stochastic volatility model
Preprint, available at https://papers.ssrn.com/sol3/papers.cfm?abstract_id=3288882
Only users who have a paid subscription or are part of a corporate subscription are able to print or copy content.
To access these options, along with all other subscription benefits, please contact info@risk.net or view our subscription options here: http://subscriptions.risk.net/subscribe
You are currently unable to print this content. Please contact info@risk.net to find out more.
You are currently unable to copy this content. Please contact info@risk.net to find out more.
Copyright Infopro Digital Limited. All rights reserved.
You may share this content using our article tools. Printing this content is for the sole use of the Authorised User (named subscriber), as outlined in our terms and conditions - https://www.infopro-insight.com/terms-conditions/insight-subscriptions/
If you would like to purchase additional rights please email info@risk.net
Copyright Infopro Digital Limited. All rights reserved.
You may share this content using our article tools. Copying this content is for the sole use of the Authorised User (named subscriber), as outlined in our terms and conditions - https://www.infopro-insight.com/terms-conditions/insight-subscriptions/
If you would like to purchase additional rights please email info@risk.net
More on Banking
Volatility shape-shifters: arbitrage-free shaping of implied volatility surfaces
Manipulating implied volatility surfaces using optimal transport theory has several applications
Infrequent MtM reduces neither value-at-risk nor backtesting exceptions
Frequency of repricing impacts volatility and correlation measures
SABR convexity adjustment for an arithmetic average RFR swap
A model-independent convexity adjustment for interest rate swaps is introduced
Joint S&P 500/VIX smile calibration in discrete and continuous time
An arbitrage-free model for exotic options that captures smiles and futures is presented
The carbon equivalence principle: minimising the cost to carbon net zero
A method to align incentives with sustainability in financial markets is introduced
Leveraged wrong-way risk
A model to assess the exposure to leveraged and collateralised counterparties is presented
Neural joint S&P 500/VIX smile calibration
A one-factor stochastic local volatility model can solve the joint calibration problem
Most read
- Breaking out of the cells: banks’ long goodbye to spreadsheets
- Too soon to say good riddance to banks’ public enemy number one
- Industry calls for major rethink of Basel III rules