Podcast: Piterbarg and Antonov on alternatives to neural networks
Two novel approximation techniques can overcome the curse of dimensionality
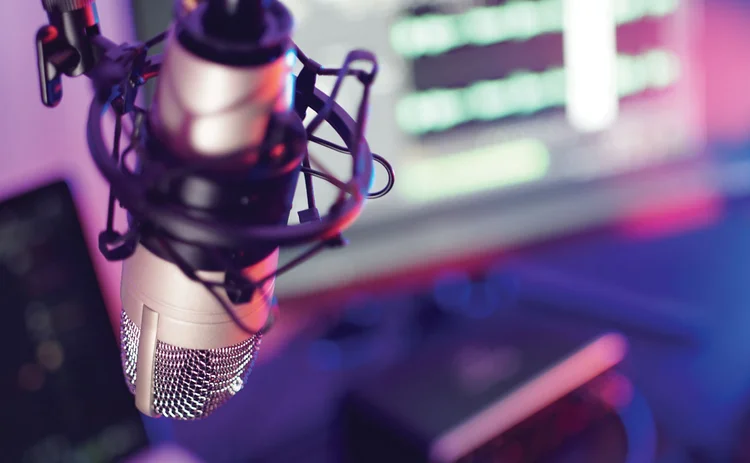
The rise of neural networks in finance has been rapid and nearly uncontested. The idea that this type of artificial intelligence is the best solution for all manner of problems is almost casually accepted by quants. But there are some sceptics – one such being Vladimir Piterbarg, head of quantitative analytics and development at NatWest Markets. Piterbarg jokes that his warnings about poorly thought-out applications of neural networks, which he has compared to a hammer in search of nails, have given him a bad reputation in the industry.
Despite many successful applications in finance and especially non-financial areas, Piterbarg argues quants too often turn a blind eye to the drawbacks of neural networks, such as training time, data availability, and the predictability and interpretability of outputs.
He has spent the past two years working with Alexandre Antonov, quantitative research and development lead at the Abu Dhabi Investment Authority, on alternative approaches to solving quantitative problems that do not have the same drawbacks.
In this episode of Quantcast, Piterbarg and Antonov discuss the two methods they developed to approximate functions that are cumbersome to calculate – for instance, because they involve lengthy simulations – and to compute conditional expectations.
“We’ve come up with much better and much faster methods for the typical problems that we see in finance,” says Piterbarg.
The two methods are called generalised stochastic sampling (GSS) and functional tensor train (FTT). Antonov describes GSS as “a parametric representation of the function”. The method involves setting up a grid of randomly distributed bell-shaped points to represent the multi-dimensional space of the function. Randomly selected points are then used to reconstruct the function. The approach mimics image reconstruction and helps avoid the curse of dimensionality that classic parametric models would encounter in a multi-dimensional space.
“If the function is relatively smooth, then our method’s precision is higher [than that of neural networks],” explains Antonov. “All the problems we have described for neural networks are more or less overcome.”
FTT reduces the dimensionality of a function. Inspired by a 2011 paper by Ivan Oseledets, this method allows a 10-dimensional function to be decomposed into the product of two-dimensional functions. “That gives structure, explainability, and much faster performance,” says Piterbarg
The two methods can be combined to “translate the problem from the function being sampled on a stochastic into a linear tensor train problem”, says Piterbarg. That lightens the problem considerably, while still allowing for multi-dimensional functions.
These methods can be applied to any problem described by functions that are slow to calculate, as well as problems related to the computation of conditional expectations, such as payoffs of complex financial products. The computation of derivatives valuation adjustments, which require the calculation of a large number of present values of simulated paths, is another use case.
It’s too early to know how widely this approach will be adopted in the industry. “NatWest Markets is looking at it,” says Piterbarg, adding the caveat that the research has only recently been published and it takes time for banks to decide whether to switch to a new model.
But he’s optimistic that the industry will see the value of GSS and FTT. “For a certain class of problems that are important in finance, this method beats neural networks hands down, there’s no doubt in my mind about it.”
Index
00:00 Intro
01:48 The drawbacks of neural networks
08:00 The need for alternative approaches
10:38 Generalised stochastic sampling
17:40 Functional tensor train
24:00 Combining the methods in practice
30:10 Comparing GSS and FTT to neural networks
31:40 Next steps
To hear the full interview, listen in the player above, or download. Future podcasts in our Quantcast series will be uploaded to Risk.net. You can also visit the main page here to access all tracks, or go to the iTunes store, Spotify or Google Podcasts to listen and subscribe.
Only users who have a paid subscription or are part of a corporate subscription are able to print or copy content.
To access these options, along with all other subscription benefits, please contact info@risk.net or view our subscription options here: http://subscriptions.risk.net/subscribe
You are currently unable to print this content. Please contact info@risk.net to find out more.
You are currently unable to copy this content. Please contact info@risk.net to find out more.
Copyright Infopro Digital Limited. All rights reserved.
You may share this content using our article tools. Printing this content is for the sole use of the Authorised User (named subscriber), as outlined in our terms and conditions - https://www.infopro-insight.com/terms-conditions/insight-subscriptions/
If you would like to purchase additional rights please email info@risk.net
Copyright Infopro Digital Limited. All rights reserved.
You may share this content using our article tools. Copying this content is for the sole use of the Authorised User (named subscriber), as outlined in our terms and conditions - https://www.infopro-insight.com/terms-conditions/insight-subscriptions/
If you would like to purchase additional rights please email info@risk.net
More on Cutting Edge
Podcast: Olivier Daviaud on P&L attribution for options
JP Morgan quant discusses his alternative to Greeks decomposition
Rethinking P&L attribution for options
A buy-side perspective on how to decompose the P&L of index options is presented
Volatility shape-shifters: arbitrage-free shaping of implied volatility surfaces
Manipulating implied volatility surfaces using optimal transport theory has several applications
Georgios Skoufis on RFRs, convexity adjustments and Sabr
Bloomberg quant discusses his new approach for calculating convexity adjustments for RFR swaps
Infrequent MtM reduces neither value-at-risk nor backtesting exceptions
Frequency of repricing impacts volatility and correlation measures
Dynamic margining long/short equity trading strategies
A repo haircut model extends a previous solution for long-only strategies
SABR convexity adjustment for an arithmetic average RFR swap
A model-independent convexity adjustment for interest rate swaps is introduced
Joint S&P 500/VIX smile calibration in discrete and continuous time
An arbitrage-free model for exotic options that captures smiles and futures is presented
Most read
- Podcast: Olivier Daviaud on P&L attribution for options
- For a growing number of banks, synthetics are the real deal
- SG trader dismissals shine spotlight on intraday limit controls