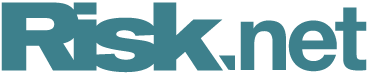
Quant of the year: Artur Sepp
Risk Awards 2024: ‘Robust’ stochastic volatility model came along just when it was needed

Stochastic volatility models have been a major focus of quantitative research for more than two decades. Those days may be over.
In August, Artur Sepp and Parviz Rakhmonov published a paper in Risk.net that seemed to tackle almost every major problem with stochastic volatility models. Their approach for pricing swaptions captures the yield curve in a flexible way, fits the market data precisely and holds under even the most extreme market scenarios.
Alexei Kondratyev, quantitative research and development lead at the Abu Dhabi Investment Authority, believes it could be the last word on the subject. “There are Risk papers that open an era of research,” he says, citing Vladimir Piterbarg’s 2010 paper on derivatives funding that spawned a new body of research on valuation adjustments. “And [there are] papers that close an era, like this one did with stochastic volatility.”
That may strike some as hyperbolic, but other prominent quants either agree with that assessment or concede it is not far from the truth – at least when it comes to interest rates.
“It is one of the important papers dealing with how to build the yield curve in a tractable way,” says Alex Lipton, global head of quant research at Adia.

Even those that see avenues for further research on stochastic volatility – and that includes Sepp, who is working on extending the paper – concede it moves the field forward.
Dominique Bang, a managing director in fixed income quantitative modelling at Bank of America in Paris, says that while the model is not perfect or without limitations, it still “offers some advantages and might be a source of inspiration to enhance our own approaches”.
A key feature of Sepp and Rakhmonov’s model is that it allows the correlation between volatility and an underlying asset to be positive – a radical departure from what has come before. Standard quant models – such as the local volatility model – assume this relationship is always negative. That was disproven in the current interest rate cycles. As central banks waged an unusually aggressive battle against inflation, volatility and interest rates rose in lockstep – a dislocation that commonly used models could not handle.
For Sepp, the paper is the culmination of more than a decade of research. It extends the beta stochastic volatility model for equities that he developed with Piotr Karasinski and published in Risk.net in 2012. The paper also reflects many of the concepts and techniques that have defined Sepp’s career – including the use of integral transforms and his pursuit of universal models free of artificial constraints.
Lipton, who has followed Sepp’s work for nearly two decades, says the model’s throwbacks to past research should inspire quants at a time when markets seem to be going back to old habits. “It is the time to revisit some of our old fixed-income armoury and this is a very helpful addition to the arsenal quants have.”
Broken rates models
Standard options pricing models use a local volatility component to capture the skewness of the implied volatility curve, combined with a stochastic volatility component to produce the curvature of the smile. The correlation between the volatility and variations in the underlying is assumed to be zero, which makes the framework more tractable. Just as well, considering the local volatility model is mathematically incapable of reproducing a positive correlation between volatility and the underlying. But this hardly seemed to matter in a world where correlations were always negative.
But not all worlds are the same. By 2021, Sepp was working on cryptocurrencies, which – unlike traditional assets – have a positive correlation to volatility.
Around this time, Sepp received a call from Rakhmonov, who at the time was working as a quantitative analyst at Citi. Rakhmonov had implemented several variations of Sepp and Karasinski’s beta stochastic volatility model – which he considers to be “far superior” to alternatives, such as the Heston model – for pricing bespoke derivatives. “I had questions about the analytical tractability of his model,” he tells Risk.net. But the conversation soon veered into crypto and whether the model could be extended to assets that were positively correlated to volatility.
We realised that, using our beta stochastic volatility model with the quadratic drift, we can propose elegant and robust dynamics for interest rate derivatives
Artur Sepp
Rakhmonov and Sepp eventually solved the problem by making the drift of the volatility process quadratic. This resulted in a universal model that works for assets with both positive and negative volatility betas.
It also introduced some complexities. When the drift of the volatility is considered as a quadratic term, it falls behind the well-established results for linear models. Rakhmonov and Sepp had to prove rigorously that the volatility dynamics are strictly positive and do not explode, which is important for Monte Carlo simulations. They also had to revise the classic Feynman-Kac formula used to derive yield curves and term structures to prove that the solution for options prices was finite and can be computed by solving the corresponding partial differential equation. Finally, they provided the first and second order expansion to compute the moment-generating functions for the model and used it to price inverse and vanilla crypto options.
“We collaborated remotely during the pandemic,” Rakhmonov recalls. “For almost a year, we had calls every Saturday to discuss the progress – sometimes two or three times a week. We broke the problem into smaller pieces and we addressed them one by one, and we fully implemented it in Python.”
By the time they finished, the next step was staring them in the face. “Last year, I heard that quant models for fixed income started to work poorly after rates increased significantly along with rates volatility,” says Sepp. “We realised that, using our beta stochastic volatility model with the quadratic drift, we can propose elegant and robust dynamics for interest rate derivatives.”
Rakhmonov and Sepp quickly extended the results of their paper on pricing crypto options to interest rates and derived a closed-form solution for the valuation and calibration of market implied volatilities of swaptions.
“We wanted to introduce a single factor yield-curve model that is well specified and robust,” says Rakhmonov.
Their ‘robust’ stochastic volatility model for interest rates is designed to work in all market environments. It can handle positive and negative volatility correlations, captures the yield curve accurately, and works quickly to price swaptions.
The paper was widely read on Risk.net and has been positively received by practitioners. “What Sepp and Rakhmonov suggest here is to go back to the idea of introducing correlation to control the skew, and thus remove the local vol component that often leads to explosive paths,” says Bang at Bank of America. “From a financial perspective, assuming correlation between underlying and stochastic vol makes lots of sense.”
An Estonia’s throw from Wall Street
Sepp’s journey to quant prominence began at Tallinn University in his native Estonia, where he completed his undergraduate degree in economics. He specialised in finance, delving into the work of Markowitz and Black-Scholes and even building his own portfolio optimisation tool in Visual Basic. He then enrolled at the University of Tartu, earning a masters in statistics and another in financing engineering before taking his PhD in probability theory – while simultaneously earning a third masters from Northwestern University, this time in industrial engineering. His PhD adviser Kalev Parna also encouraged Sepp to attend classes in numerical calculus and mathematical physics, which were beyond the curriculum requirements, setting him on a course that eventually led to Wall Street.
Sepp’s PhD thesis was based on Alex Lipton’s 2002 paper, Assets with jumps, which inserted a jump diffusion process into a pricing model to account for sudden and extreme market moves. The analytical treatment of a jump-diffusion process as it crosses a barrier was and remains a difficult problem to solve. Sepp extended Lipton’s solution, using two jumps and two barriers instead of one. Lipton was impressed and helped get a paper based on his thesis published in 2004.
Sepp joined Bear Stearns for an internship in New York in 2006. The following year, he met Lipton in person for the first time. Lipton immediately asked him to join his team at Merrill Lynch. “Of course I agreed,” says Sepp. He joined Merrill in 2007 as a credit derivatives strategist and had a front row seat for the 2008 global financial crisis.

The experience proved formative. Credit spreads widened to levels never seen before and risk models ceased to function. “I saw it in action. I saw how models may stop working. You could not compute risk and you had to find some patches to fix them,” he says. “In the end I learned that models should be relatively simple and should account for as many scenarios as possible. And I learned the importance of reliable data to fit the models.”
Sepp moved to Bank of America after it acquired Merrill in late 2008. The following year, his focus switched to equity derivatives. He began working on structured products and volatility models – specifically, stochastic and local volatility. An early research project with Lipton turned into a paper – Filling the gaps, published in Risk in 2011 – that introduced a method to calibrate local volatility to vanilla options even when data is sparse.
His next major project was prompted by a chance encounter at a conference at Imperial College London. After Sepp gave a presentation, he was approached by Karasinski, a renowned rates and volatility quant who at the time was at the European Bank for Reconstruction and Development. The conversation turned to the assumptions and limitations of standard volatility models. The common approach – the Heston model – is difficult to simulate with Monte Carlo processes because its variance, which should be strictly positive, can hit zero. That in turn creates instabilities when running Monte Carlo simulations.
[Lipton] did me a big favour. He gave me his book on blockchain and distributed ledgers to proofread. I was immediately interested because I saw the mathematics I know could be applied to that market too
Artur Sepp
Sepp and Karasinski designed an alternative to the Heston model, called the beta stochastic volatility model, and published it in Risk in 2012. The model assumes volatility is log normal with a linear drift and negative correlation to the returns of the underlying asset. The correlation parameter was dubbed ‘volatility beta’ and gave the model its name. The model was relatively easy to implement and intuitively captured the relationship between volatility and returns. And despite being rooted in equities, a decade later, it would form the basis of Sepp’s work on interest rates volatility.
But first, Sepp decided to try something different. In 2015, he moved to the buy side, joining Julius Baer in Zurich to build trading strategies and an asset management platform for investors. Then, in 2018, he moved to Quantica Capital, a Swiss hedge fund where he got more hands-on with developing quantitative methods to detect trading signals.
In 2021, Sepp reconnected with Lipton, who at the time was researching cryptocurrencies. “He did me a big favour,” says Sepp. “He gave me his book on blockchain and distributed ledgers to proofread. I was immediately interested because I saw the mathematics I know could be applied to that market too.”
Later that year, Sepp joined Sygnum, a digital asset banking group in Switzerland, which gave him the opportunity to do just that. He began working on applying the beta stochastic volatility model to crypto options. Then, out of the blue, Rakhmonov called.
Not over yet
In conversations with Risk.net, many of Sepp’s peers pointed to his versatility as one of his greatest attributes. Most quants spend their entire careers working with a single asset class. Sepp has made meaningful contributions to the academic literature on credit, equities, rates and, more recently, cryptocurrencies, while working across the buy and sell side. He continues to wear many hats, without losing sight of the bigger picture and the need for universal models that work in all markets and scenarios.
Sepp’s recent research has mostly focused on cryptocurrencies, which he considers not only “an asset class on their own with their properties” but also “a good diversifier for broad portfolios with equities, bonds and alternatives”. His most recent paper, published in Risk.net in late 2023, presents empirical evidence that it is optimal to include at least a small allocation to bitcoin and ether in a diversified portfolio.
As long as there are interesting challenges, I don’t think crypto will ever become boring
Artur Sepp
He has also continued to collaborate with Lipton on the applications of blockchain technology. In 2022, they designed an automated market-making framework to execute on-chain transactions of central bank digital currencies consistently with off-chain currencies, de facto eliminating the need for intermediaries.
That paper led to his latest role at Clearstar Labs, a Zurich-based family office specialising in digital assets, where he is the head of quantitative strategies – a move that has brought him closer to his roots. “At Clearstar Labs, I feel more like a quant because we do market-neutral strategies by hedging our risk with trading in delta and in options,” he says.
Asked if he is in the crypto industry for the long-term, Sepp says: “As long as there are interesting challenges, I don’t think it will ever become boring.”
Fortunately, Sepp isn’t done with traditional assets yet – nor does he consider his paper on rates volatility to be the last word on the subject. He is currently working with Rakhmonov to extend the model under the general Heath-Jarrow-Morton framework introduced by Andrei Lyashenko and Yevgeny Goncharov in a paper published in Risk.net in June. In that paper, the evolution of the yield curve is captured by multiple factors – namely level, slope and convexity – that describe the relationship between rates and volatility. That gives the model high flexibility while maintaining robust results.
Sepp and Rakhmonov’s closed-form expansion generalises to the case of multi-factor dynamics. The final framework allows for the joint valuation and risk management of swaptions and options on rates futures across multiple tenors and expiries. “We show that our expansion is very accurate and it enables fast calibration of models to market data,” says Sepp.
An even better model for rates volatility may be just around the corner.
Only users who have a paid subscription or are part of a corporate subscription are able to print or copy content.
To access these options, along with all other subscription benefits, please contact info@risk.net or view our subscription options here: http://subscriptions.risk.net/subscribe
You are currently unable to print this content. Please contact info@risk.net to find out more.
You are currently unable to copy this content. Please contact info@risk.net to find out more.
Copyright Infopro Digital Limited. All rights reserved.
As outlined in our terms and conditions, https://www.infopro-digital.com/terms-and-conditions/subscriptions/ (point 2.4), printing is limited to a single copy.
If you would like to purchase additional rights please email info@risk.net
Copyright Infopro Digital Limited. All rights reserved.
You may share this content using our article tools. As outlined in our terms and conditions, https://www.infopro-digital.com/terms-and-conditions/subscriptions/ (clause 2.4), an Authorised User may only make one copy of the materials for their own personal use. You must also comply with the restrictions in clause 2.5.
If you would like to purchase additional rights please email info@risk.net
More on Awards
Joining the dots: banks leverage tech advancements for the future of regulatory reporting
The continued evolution of regulatory frameworks is creating mounting challenges for capital markets firms in achieving comprehensive and cost-effectiveawa compliance reporting. Regnology discusses how firms are starting to use a synthesis of emerging…
Markets Technology Awards 2024 winners' review
Vendors spy opportunity in demystifying and democratising – opening up markets and methods to new users
Derivatives house of the year: JP Morgan
Risk Awards 2024: Response to regional banking crisis went far beyond First Republic
Risk Awards 2024: The winners
JP Morgan wins derivatives house, lifetime award for El Karoui, Barclays wins rates
Best product for capital markets: Murex
Asia Risk Awards 2023
Technology vendor of the year: Murex
Asia Risk Awards 2023
Best structured products support system: Murex
Asia Risk Awards 2023
Energy Risk Asia Awards 2023: the winners
Winning firms demonstrate resiliency and robust risk management amid testing times