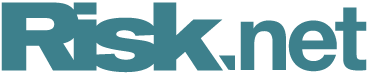
QMA’s Dyson: AI a ‘wonderful marketing concept’
Quant firm sceptical about using artificial intelligence to seek out tradable strategies
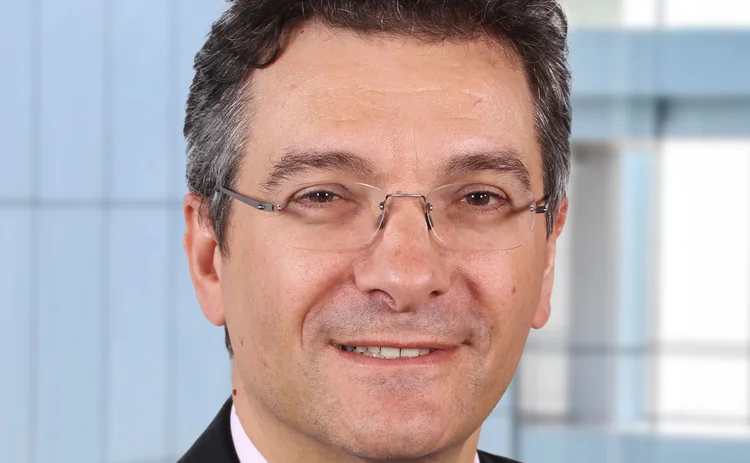
A signal generated by an artificial intelligence algorithm that automatically reads earnings transcripts was the best-performing indicator of stock returns last year at $121.8 billion quantitative equity and global multi-asset manager QMA Asset Management.
Still, Andrew Dyson, chief executive at the quant shop owned by PGIM, the $1 trillion global investment management business of Prudential Financial, is less than enthusiastic about the asset management industry’s recent fascination with AI.
QMA is exploring the use of the technology to finesse its trading algorithms “at the margins” but is less likely to use it to derive trading signals, Dyson says.
“We are testing artificial intelligence in a number of scenarios, but we’re very skeptical of its value. There may be places where you can improve your trading process that we are actively considering. But as a decision-making tool we’re not using it and not by accident. We remain very wary of AI. It is a wonderful marketing concept. But, I think it’s easy to get carried away and overstate its value at the investment level,” Dyson says.
AI is being used to optimise everything from golf clubs and swings to the flavour of beer. But while it is reasonable to believe that repeating something like a golf swing, for example, will produce the same outcome, it is contested whether finance has similar repeatable patterns or laws of nature that machines can learn.
Dyson is one of several voices in the quant world to question whether the patterns of financial markets can be learned at all, given their inherently volatile nature.
“I can at least intuitively believe that you could design a better golf club using AI, but I can’t test and learn in that same independent way in finance. I can mine the historic data, but the future is completely different,” Dyson says. “We can test and learn within a particular single cycle, but we can’t have any confidence that the next time, it will be repeated.”
From a marketing point of view, people are making AI sound like a transformative story. I don’t think it is
Andrew Dyson
QMA manages systematic quantitative equity and global multi-asset strategies. Last year, it strengthened its quant credentials with the acquisition of Wadhwani Asset Management (WAM), a London-based quantitative macro-focused investment management firm. At the core of the firm’s equity platform is a dynamic multifactor quant strategy that diversifies across a range of more than a dozen signals, loosely grouped around value and growth.
Underlying Dyson’s skepticism about AI is the firm’s core philosophy that all signals must have some understandable rationale, whether economic or behavioural. One of the biggest dangers with AI is that models become impossible to follow. At the same time, all statistical models are also notoriously vulnerable to finding patterns in the data that are meaningless – a problem known as overfitting. AI, Dyson says, makes matters worse.
“Overfitting is fundamentally a problem of having too many variables and deriving a spurious correlation, particularly, if those underlying variables are connected to one another. The problem with AI is you are taking in many more variables and you’ve actually got no way of knowing whether they are even connected to one another. So you’re creating the conditions where you could see more overfitting and it becomes harder to diagnose as a result,” Dyson says.
This is not to say QMA rejects AI entirely. Dyson says data is an integral tool for any progressive manager, quant or fundamental. Funds lacking data science skills are “handicapping” themselves, he says, and here – with the use of natural language processing – the firm does see a use for AI techniques.
Much of QMA’s data science efforts to date have focused on finding indicators of stock returns orthogonal to its existing signals. Dyson says the risk management of the fund comes directly from this diversification across multiple signals.
“Big data-driven signals are actually quite appealing to us, because they’re likely to add something that’s different to what we have, whereas for another type of signal, the odds are we are already capturing that information,” he says. “A signal based on news scrubbing, for example, is totally different from some sort of accounting-derived valuation metric.”
QMA is currently adding a news-scraping signal that uses NLP to its portfolios. The firm is also using the same approach to gather insights from earnings call transcripts to fill information gaps in emerging markets where it can be harder to get hard financial data.
“We’ve been using NLP for some time. We found it additive to our existing signals. We’re happy to consider individual signals that have a big data or unstructured data element if they pass our normal evidential sort of tests,” Dyson adds.
But that is quite different from using AI to root out new trading strategies, he is clear. “From a marketing point of view, people are making AI sound like a transformative story,” Dyson says. “I don’t think it is.”
Only users who have a paid subscription or are part of a corporate subscription are able to print or copy content.
To access these options, along with all other subscription benefits, please contact info@risk.net or view our subscription options here: http://subscriptions.risk.net/subscribe
You are currently unable to print this content. Please contact info@risk.net to find out more.
You are currently unable to copy this content. Please contact info@risk.net to find out more.
Copyright Infopro Digital Limited. All rights reserved.
You may share this content using our article tools. Printing this content is for the sole use of the Authorised User (named subscriber), as outlined in our terms and conditions - https://www.infopro-insight.com/terms-conditions/insight-subscriptions/
If you would like to purchase additional rights please email info@risk.net
Copyright Infopro Digital Limited. All rights reserved.
You may share this content using our article tools. Copying this content is for the sole use of the Authorised User (named subscriber), as outlined in our terms and conditions - https://www.infopro-insight.com/terms-conditions/insight-subscriptions/
If you would like to purchase additional rights please email info@risk.net
More on Asset management
Pension schemes prep facilities to ‘repo’ fund units
Schroders, State Street and Cardano plan new way to shore up pension portfolios against repeat of 2022 gilt crisis
Fears of runaway risk on offshore reinsurance
Life insurers catch the eye of UK regulator for pension buyout financing trick
Hot topic: SEC climate disclosure rule divides industry
Proposal likely to flounder on First Amendment concerns, lawyers believe
‘Brace, brace’: quants say soft landing is unlikely
Investors should prepare for sticky inflation and volatile asset prices as central banks grapple with turning rates cycle
Trend following struggles to return to vogue
Macro outlook for trend appears to be favourable, but 2023’s performance flop gives would-be investors pause for thought
Start-up bond platform OpenYield prepares to launch
Start-up aims to give retail brokers the same electronic liquidity used by the professionals
Can machine learning help predict recessions? Not really
Artificial intelligence models stumble on noisy data and lack of interpretability
How patchy liquidity is stymieing systematic credit
…and what investors like AllianceBernstein, Man Numeric and Acadian are doing about it