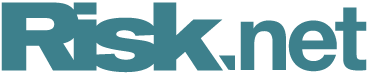
Machine learning will create new sales-bots – UBS’s Nuti
Technologists working to automate indications of interest from trading desks
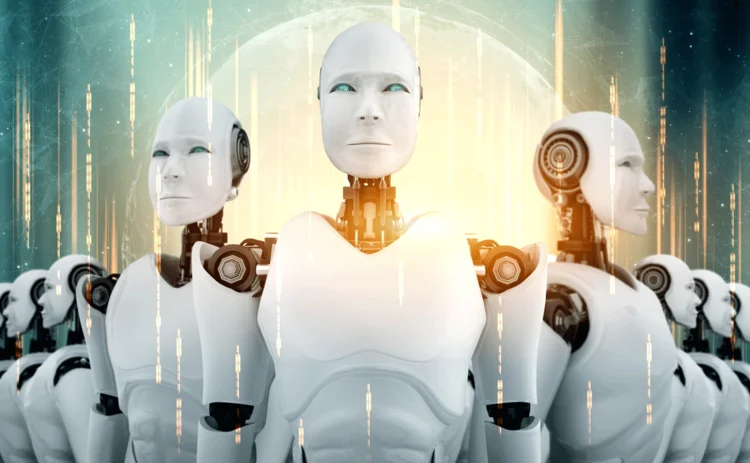
Trading is moving to a point where there is almost no human involvement, as market participants employ algorithms that generate – and respond to – indications of interest (IOIs), according to Giuseppe Nuti, head of machine learning at UBS.
“I imagine that in the next few years, we’re going to see a lot more approaches coming up. So much so that it will even be algorithmic-to-algorithmic IOIs, almost removing the human component,” Nuti said.
Market-makers issue IOIs, or ‘axes’, to signal
Only users who have a paid subscription or are part of a corporate subscription are able to print or copy content.
To access these options, along with all other subscription benefits, please contact info@risk.net or view our subscription options here: http://subscriptions.risk.net/subscribe
You are currently unable to print this content. Please contact info@risk.net to find out more.
You are currently unable to copy this content. Please contact info@risk.net to find out more.
Copyright Infopro Digital Limited. All rights reserved.
You may share this content using our article tools. Printing this content is for the sole use of the Authorised User (named subscriber), as outlined in our terms and conditions - https://www.infopro-insight.com/terms-conditions/insight-subscriptions/
If you would like to purchase additional rights please email info@risk.net
Copyright Infopro Digital Limited. All rights reserved.
You may share this content using our article tools. Copying this content is for the sole use of the Authorised User (named subscriber), as outlined in our terms and conditions - https://www.infopro-insight.com/terms-conditions/insight-subscriptions/
If you would like to purchase additional rights please email info@risk.net
More on Technology
Dismantling the zeal and the hype: the real GenAI use cases in risk management
Chartis explores the advantages and drawbacks of GenAI applications in risk management – firmly within the well-established and continuously evolving AI landscape
Chartis RiskTech100® 2024
The latest iteration of the Chartis RiskTech100®, a comprehensive independent study of the world’s major players in risk and compliance technology, is acknowledged as the go-to for clear, accurate analysis of the risk technology marketplace. With its…
T+1: complacency before the storm?
This paper, created by WatersTechnology in association with Gresham Technologies, outlines what the move to T+1 (next-day settlement) of broker/dealer-executed trades in the US and Canadian markets means for buy-side and sell-side firms
Empowering risk management with AI
This webinar explores how artificial intelligence (AI) can strip out the overheads and effort of rapidly modelling, monitoring and mitigating risk
Core-Payments for business leaders: why real-time access to payment data is key to long‑term business success
Business leaders require easy access to timely, reliable and complete information across post-trade processes. Aside from the usual requirements of senior managers to optimise for risk, revenues and costs, they increasingly need to demonstrate to their…
Risk applications and the cloud: driving better value and performance from key risk management architecture
Today's financial services organisations are increasingly looking to move their financial risk management applications to the cloud. But, according to a recent survey by Risk.net and SS&C Algorithmics, many risk professionals believe there is room for…
Machine learning models: the validation challenge
Machine learning models are seeing increasing demand across the capital markets spectrum. But how can firms improve their chances of gaining internal and regulatory approval for these type of models?
Most read
- Breaking out of the cells: banks’ long goodbye to spreadsheets
- Too soon to say good riddance to banks’ public enemy number one
- Basel Committee reviewing design of liquidity ratios