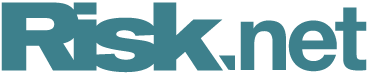
CECL models may leave banks ill-prepared for next downturn
Mortgage backtest study shows some loan-loss models miss the mark
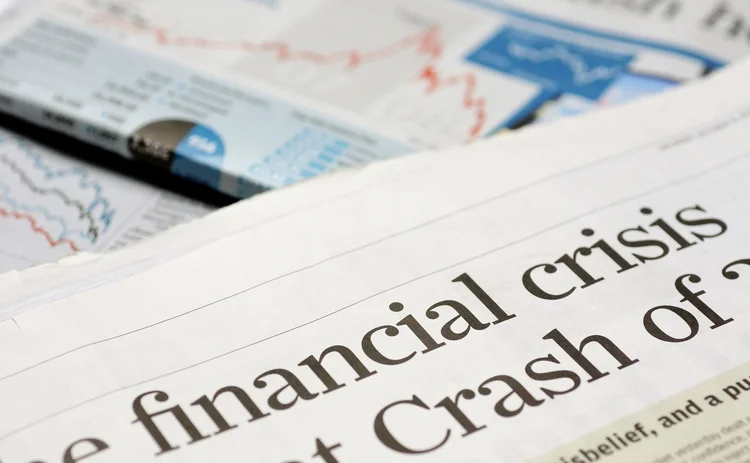
Some models used by US lenders to set loan-loss provisions under new accounting rules fail to produce accurate estimates of credit risk during economic downturns, research reveals. The study backtested six modelling methods with mortgage data from the financial crisis of 2008 and showed that most models were unable to equip banks with appropriate levels of reserves.
The results raise questions for banks as they adapt to the Current Expected Credit Loss (CECL) regime, a new set of standards that
Only users who have a paid subscription or are part of a corporate subscription are able to print or copy content.
To access these options, along with all other subscription benefits, please contact info@risk.net or view our subscription options here: http://subscriptions.risk.net/subscribe
You are currently unable to print this content. Please contact info@risk.net to find out more.
You are currently unable to copy this content. Please contact info@risk.net to find out more.
Copyright Infopro Digital Limited. All rights reserved.
You may share this content using our article tools. Printing this content is for the sole use of the Authorised User (named subscriber), as outlined in our terms and conditions - https://www.infopro-insight.com/terms-conditions/insight-subscriptions/
If you would like to purchase additional rights please email info@risk.net
Copyright Infopro Digital Limited. All rights reserved.
You may share this content using our article tools. Copying this content is for the sole use of the Authorised User (named subscriber), as outlined in our terms and conditions - https://www.infopro-insight.com/terms-conditions/insight-subscriptions/
If you would like to purchase additional rights please email info@risk.net
More on Risk management
Clear warning on escape hatch for optimisation trades
CCPs fear Emir clearing mandate carve-out for portfolio rebalancing could be abused
One year on, regulators still want a cure for bank runs
Broad support for higher outflow assumptions on uninsured deposits, but that won’t save insolvent banks
Falling T2 balances bode well for eurozone’s stability
Impact of fragmentation would be less severe today than in 2010s, says Marcello Minenna
For a growing number of banks, synthetics are the real deal
More lenders want to use SRTs to offload credit risk, but old hands say they have a long road ahead
Did Fed’s stress capital buffer blunt CCAR?
Experts fear flagship test’s use as a capital top-up has undermined its role in risk management
How Ally found the key to GenAI at the bottom of a teacup
Risk-and-tech chemistry – plus Microsoft’s flexibility – has seen US lender leap from experiments to execution
Industry urges focus on initial margin instead of intraday VM
CPMI-Iosco says scheduled variation margin is better than ad hoc calls by clearing houses
Consortium backs BGC’s effort to challenge CME
Banks and market-makers – including BofA, Citi, Goldman, Jump and Tower – will have a 26% stake in FMX
Most read
- Breaking out of the cells: banks’ long goodbye to spreadsheets
- Too soon to say good riddance to banks’ public enemy number one
- Basel Committee reviewing design of liquidity ratios