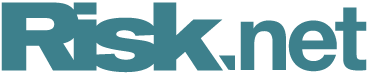
Tech-driven model risk management – Driving value and efficiency

Next-generation model risk management (MRM) tools such as automated model documentation, performance monitoring and powerful network visualisations can improve efficiency by minimising time-consuming tasks and allowing organisations to focus more on high-value activities. A forum of industry leaders discusses the considerations and opportunities of technology-driven MRM, how emerging technologies are changing banks’ approaches to MRM, and the impact of regulation going forward
The panel
- Konstantina Armata, Global Head, Model Risk Management, Barclays
- David Asermely, Global Lead, SAS Model Risk Management
- Javier Calvo Martín, Partner, Management Solutions
- Anne-Cécile Krieg, Deputy Head of Model Risk Management, Societe Generale
- Ashutosh Nawani, Head of Financial Risk Management, PwC
- Slava Obraztsov, Global Head of Model Risk, Nomura
What impact is growth in financial models having on banks?

David Asermely, SAS: It’s an increasingly competitive analytical world, and having better information differentiates top banks from the pack. As a result, financial institutions are relying more on models to make critical business decisions – from assessing risk and capital planning, to investment management and financial reporting. Models also play a critical role in regulatory and accounting compliance processes, such as performing stress tests, calculating expected credit loss – International Financial Reporting Standard 9 and Current Expected Credit Loss – and conducting anti-money laundering investigations. Banks are responsible for developing, supporting and using their valuable models appropriately, so they are spending more resources on modelling activities.
Ashutosh Nawani, PwC: In the case of trading algorithms that result in fast-paced, high-frequency market activity, this can lead to equally swift accumulation of losses. With multiple market participants utilising algorithms that may not have undergone sufficient testing and validation, there is a potential systemic model risk that could result in large-scale market abuse and a financial disaster.
Where these new types of models sneak into the model inventory – and as a result face rigorous model risk policies – there may not be relevant expertise within the model risk function to perform a sufficient review. Or, more often, there may not be capacity or resource to bring them the governance framework as efficiently and as quickly as possible.
Regulators have certainly shown they are keen to try to solve the problem, with the UK Financial Conduct Authority and the Prudential Regulation Authority (PRA) conducting reviews across firms and publishing guidance and supervisory statements earlier this year regarding algorithmic trading.
First, a firm is expected to explicitly approve the governance framework, bodies, controls and policies for algorithmic trading. This includes the identification and empowerment of the specific management body to manage the model risk of such models with resources with suitable expertise. The next step should be to reassess the definition of models within the bank to ensure these new model types are covered. These new categories of models should be listed in a dedicated model inventory, including associated controls and other mitigants – for example, kill-switch procedures.
Slava Obraztsov, Nomura: Defining the scope of the MRM process has always been quite a difficult challenge. Definition in SR 11‑7 is quite broad and, for example, the Prudential Regulation Authority’s (PRA’s) SS3/18 MRM principles for stress testing provide only some directions as to how models should be defined. It is therefore important for financial institutions to find a balance between how wide the applied scope should be, while preventing MRM from becoming a box-ticking exercise. Nomura concentrates on models where the definition includes at least one model assumption that could be an expert judgement. Similar approaches are employed by others where banks try to distinguish between models and tools, and different processes are applied in those situations. Recently, some regulators, such as the PRA, have made no significant distinction between models and tools by requiring the risks associated with the implementation of all types of calculations to be adequately understood, controlled and documented. As a result, financial institutions need to adjust their MRM approaches accordingly.
Anne-Cécile Krieg, Societe Generale: The main change I can see regarding traditional financial models such as asset-liability management (ALM), valuation or credit is a cultural shift in the way they are approached. First, models are not left mostly to quants any more, as the shift now involves all the stakeholders in the model value chain, including IT personnel and bankers; second, all phases of the model lifecycle are under scrutiny, with an increased focus on robustness in stressed conditions. Most of the growth in models is coming from non-traditional areas such as back-office processes, which increasingly rely on automation and compliance.
Konstantina Armata, Barclays: Banks typically use a few thousand different models as part of their operations. Examples include models used in the front office to price transactions or to determine borrowers’ creditworthiness; models used to calculate risk-weighted assets (RWAs), liquidity, stress or provisions by risk and finance; models used by compliance to detect fraudulent activity; and even models used by researchers to assist stock evaluation. The sheer number of models and their varied nature requires extensive MRM capability and skill set, as well as a pragmatic approach to determine prioritisation and depth of coverage. The end-goal is the development of a holistic and coherent risk management framework that includes a view of model risk across the organisation that can be clearly communicated to senior stakeholders and drive decisions.
What are the main emerging risks in this area?
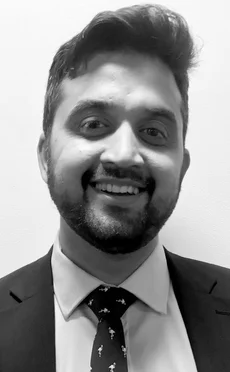
Ashutosh Nawani: The emerging risks underpinning model risk in the next two years are a direct function of the reshaping of the regulatory landscape – the Fundamental Review of the Trading Book, interbank offered rates and the standardised approach to counterparty credit risk regulation – and the adoption of new technologies.
Institutions in the early stages of defining their model risk framework must overcome the primary hurdle of poor clarity on lifecycle roles and responsibilities across the three lines of defence. This increases the risk of incorrect design and consequently a lack of (full) compliance with regulatory requirements.
Once the framework matures into a foundational stage, institutions seek increased compliance and operational costs due to cross-border governance as well as levelling out inefficiency and the cost of human capital sourcing. A key risk to address to avoid stagnation on this suboptimal front is found in the correctness of the implementation of a ‘model validation factory’. This risk will be mitigated by developing standardised model validation procedures and libraries for rapid assessment and model deployment.
Institutions with more robust and established model risk frameworks are being challenged by the risk of a lack of fit technology supporting an enterprise-level centralised data platform as a single source of truth for modelling, validation, inventory, monitoring and reporting.
Slava Obraztsov: There have been only a few new product developments in the past several years. The model universe is changing, but models are applied to broadly the same product sets, and the same products continue to cause the greatest challenges. For example, constant maturity swap (CMS) spread corridor options, which caused significant losses in 2008 due to euro curve inversion, are becoming popular again. This time, the activity is related to the flattening of the US dollar curve, where the swaps and options market is much bigger, but challenges with managing CMS spread digital risk remain the same as in 2008.
Other examples include collateralised loan obligations, which are difficult to manage from a modelling perspective, and more complexity in so‑called ‘simple’ products. Increasing regulatory and industry attention continues to be paid to the treatment of the general wrong-way risk and the development of valuation adjustments – collectively known as XVAs.
Model interconnectedness is another issue where model risk in one area could be propagated to models in other areas. It is especially relevant to so-called ‘feeder models’ – scenario-generation models in stress testing and proxy time series used in capital and exposure calculations. For a proper analysis of model interconnectedness, it is important to have a robust model inventory, including a detailed record of the applications of all model outputs.
David Asermely: Regulations and business requirements are driving banks to develop and support more models. As their model inventories grow, banks may struggle to understand the context within which their models interact; likewise, the increasing level of interconnectedness between models makes it difficult for banks to assess their true model risk. Banks are also deploying more complex models – including machine learning techniques – that are less transparent. Model volume, interconnectedness and complexity are stressing many MRM teams and systems.
Anne-Cécile Krieg: Many actors are focusing on artificial intelligence (AI) and machine learning. These are key technologies that should be specifically managed, but consistently, within the overarching framework. Another emerging risk is the potential for ‘rogue modellers’ and the difficulty of identifying all types of models that should be brought under an MRM framework. It’s becoming easier to develop models, especially with open-source code. Even a non-specialist can develop one, so there is a risk these models remain outside the reach of the MRM process. This is mitigated by strict controls of the model inventory process.
Whose responsibility is MRM?
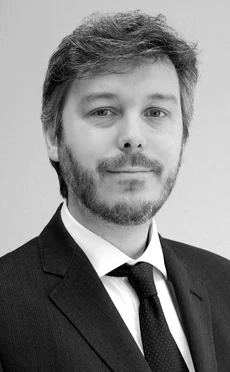
Javier Calvo Martín, Management Solutions: It is a common misconception in some banks that MRM is exclusively the responsibility of internal validation (IV) or, in a slightly broader view, risk areas.
Indeed, risk and IV carry a relevant share of the responsibility, but it is essential to acknowledge that model risk is very transversal – just like operational risk, for example – in terms of stakeholders and model lifecycle coverage.
This means the entire organisation should be involved in the appropriate management of model risk. In best-practice banks, this is achieved through the allocation of responsibilities across three lines of defence, where the first line of defence (1LoD) contains model ownership, development, monitoring, implementation and use; the second line of defence (2LoD) holds model governance and model validation; and the third line of defence (3LoD) is model audit, controlling the entire model lifecycle. Interestingly, in some countries, banking supervisors – such as the European Central Bank (ECB) or the US Federal Reserve – are referred to as the fourth line of defence and external auditors as the fifth line of defence.
This implies a deep cultural change, especially when it comes to the model owner role. Model owners are typically close to or within the business, and may sometimes be model users. In many cases, it is not at all straightforward to raise their awareness of how critical their role is for an appropriate MRM, especially in terms of responsibility and accountability. So, while the 2LoD is usually more mature in this respect, there is typically more work to be done in the 1LoD.
Management Solutions is already observing a steady increase in awareness of the transversal quality of model risk in banks, notably in non-US countries, and alongside a consistent increase in responsibility and accountability regarding MRM.
Anne-Cécile Krieg: In theory, this hasn’t changed: all three lines of defence are responsible for MRM, but operationally the mindset has shifted. In the past, it tended to be left to the 2LoD, but now there are specific roles allocated across the three lines, which are fully embraced and embedded.
In particular, within the 1LoD, all roles are better identified – from the designer of the model and the person implementing it, to the users and those tasked with surveillance, with increasing emphasis on the model owner role.
Slava Obraztsov: The aim of MRM is to develop a framework around the full model lifecycle, rather than concentrating on validation, as was previously the case. It’s a fundamental change, but who is responsible for it now?
MRM has introduced a relatively new concept of ‘model owners’, but allocating that ownership and its associated responsibilities could be a difficult task. In my experience, nobody volunteers to be a model owner, but it’s an important part of the process. There’s also a shift towards widening the responsibilities of model development areas, especially around model documentation, standards of testing and model risk analysis. The current requirements are very different from what was happening 10–15 years ago.
There is more pressure on validation teams in terms of the completeness of the model validation process, including documentation and additional analysis, attention to governance processes and involvement of senior management. Firms’ leaders don’t need to be modelling experts, but they need to be aware of major model risks. Well-designed model risk quantitative and qualitative metrics would help senior management make strategic business decisions or prioritise some model developments if model risk becomes excessive.
David Asermely: Most banks employ a multi-level approach to MRM. Model owners, developers and validators comprise the 1LoD and are responsible for producing and supporting well-functioning models. Typically, the 2LoD is the MRM group, which is responsible for crafting and enforcing policy, aggregate reporting and assessing model interconnectedness risk. Internal auditors provide an independent check to confirm the firm’s policy enforcement. Ultimately, the board of directors is responsible for understanding and properly managing model risk. The Fed’s SR 15‑18 states: “The board should direct senior management to provide information about a firm’s estimation approaches, model overlays and assessments of model performance” for all models within the capital planning process. Other regulations make similar assertions.
Konstantina Armata: As with all risk stripes, responsibility for MRM lies both with the 1LoD – mode users/developers – and the 2LoD – MRM.
How are banks’ approaches to MRM evolving?
Javier Calvo Martín: There are different starting points and varying regulatory pressure depending on region – the US versus the European Union, for example.Acknowledging this as common ground, Management Solutions has found that banks’ MRM frameworks are evolving along several dimensions:
- Formalising MRM governance, organisation and policies – specific model risk committees are being set up, MRM areas are being created and are absorbing IV units, and the whole MRM framework is being formalised in policies and procedures approved at the appropriate levels up to board level.
- Inventorying all models and deploying a model governance tool – best-practice banks have already inventoried all models, not only regulatory ones, and have deployed an MRM tool – whether a vendor system or internally developed – which acts as the backbone of the MRM process.
- Widening scope of the MRM framework – banks are trying to avoid a ‘big bang’ and are thus progressively incorporating all model types under the MRM scope (starting with regulatory models) and all business units (starting with holding and major subsidiaries). Lastly, and especially in the US, major banks incorporate non-models within the MRM scope.
- Developing model risk quantification and appetite – while still open in terms of methodology, most banks agree that measuring model risk is necessary, both in terms of model governance key performance indicators (KPIs) (for example, the number of non-validated Tier 1 models) and inherent model risk KPIs (for example, confidence interval on model outcome). This links strongly with model risk appetite, which is commonly based on these KPIs.
- Deepening the scope of the IV review – IV reviews the entire model lifecycle (data, development, implementation, monitoring and usage),
- and its reviewing techniques and tests are wider and deeper.
- Strengthening the link between models and data – the interdependency of models and data leads to a parallel development of the MRM framework and the data governance framework, including the Basel Committee on Banking Supervision’s standard 239. In practice, this results in parallel governance structures and fluent communication between them.
- Increasing concern about advanced machine learning and AI models – due to their difficult-to-interpret nature, these models pose unique challenges to their MRM, especially regarding IV activity. Banks are developing specific procedures and techniques such as enhanced sensitivity analyses to address them.
Above and beyond this, probably the major achievement in the evolution of MRM frameworks is deploying a model risk culture across the organisation, involving the whole range of stakeholders across the three lines of defence, the board and senior management. If we were to choose a single acid test of MRM evolution, the effective implementation of a sound model risk culture across the organisation would be it.
Anne-Cécile Krieg: Banks want to address both their internal risk management needs and supervisory requirements, so there is a clear need for an industrialised approach with a focus on addressing these expectations in a modern way. What is striking is the increasing awareness of model risk among senior management.
Konstantina Armata: Most banks have established a holistic MRM function as the bank’s 2LoD risk management capability for this type of risk that covers all models in the bank, although the level of maturity of this function may differ across the industry. This is a long way ahead of the starting point, where banks viewed MRM simply as one-off model validation, and even that was only for a couple of model types such as valuation models and, later, capital models. Further evolution will involve model risk being assessed in a holistic manner and ideally quantified in metrics that are well understood and, thus, enable decision-making.

Slava Obraztsov: Currently, one of the biggest challenges is quantification of model risk – at an individual level and in aggregate. For individual models, this technical assessment should include dependence on modelling assumptions and uncertainty around opaque parameters, model stability, and so on. For senior management reporting, there should be some type of model risk aggregation across different model types. How that could be achieved is hotly debated, but aggregation shouldn’t be applied to all model types. For example, aggregation of model risk across pricing models and retail models may not be very meaningful. Instead, model risk should be aggregated across relevant types of models, such as pricing models or capital models, with a set of numbers to be checked against model risk appetite and presented to senior management.
Another trend is for building a robust process for model performance monitoring. It’s important to have the right set of tools and indicators to monitor model performance under current market conditions and a portfolio composition to proactively identify and mitigate model limitations.
David Asermely: Historically, firms have undertaken MRM at an individual team level – often with tools such as Excel spreadsheets, PowerPoints, and governance, risk and compliance solutions. We are seeing, as the number of models increases, inventories growing more complex and organisations dedicating expensive resources to the development and support of these high-value assets, a shift to modernised MRM solutions. These platforms offer many efficiencies that reduce the burden on modelling teams while enforcing best-practice governance. A good example of this is performance monitoring. Regulators require financial organisations to understand how well a model is performing. With models and data changing more frequently – especially with some machine learning techniques – organisations must conduct performance monitoring more frequently. Previously, MRM teams did these types of backtesting manually. Now, however, MRM solutions provide automated performance monitoring with threshold alerts to streamline these activities and allow for better transparency.
Ashutosh Nawani: Model risk’s fast-paced journey from a tertiary and seldom-considered risk to a strategic one of equal importance to credit, market, liquidity and other strategic risks has only just begun.
Long-term focused initiatives are under way at leading institutions to transform the MRM’s structure and strategy to create a flexible, adaptable and efficient MRM function.
A constantly evolving area in model risk is the process and policy changes space. The aim is twofold. First, to more efficiently manage models based on their complexity and materiality, and avoid excessive oversight and governance driven by models’ risk profile and characteristics. Second, to simplify through optimisation and centralisation model development and validation activities such as group-level refined standard methodologies, templates and documentation.
Depth focus is currently put on automation as institutions need to move towards a ‘model validation factory’ and to industrialise standard routine and repetitive tasks for model calibration, monitoring and reporting.
Last but not least, it is critical for financial institutions to select the most appropriate framework to employ fitting technology supporting an enterprise-level centralised data platform as a single source of truth for the functions I previously mentioned and to implement workflow management tools to connect MRM components for enhanced and transparent governance and oversight.
How effective have regulatory initiatives such as SR 11‑7 and the Targeted Review of Internal Models (Trim) been in improving standards?
David Asermely: MRM regulations have forced many organisations to define and execute comprehensive policies that encompass robust model development, validation, implementation and usage. To comply with regulators, banks need a reliable MRM structure to ensure all risk categories related to models are identified, monitored and controlled. Regulators want to know whether organisations can answer these simple questions about each of their models:
- Has it been reviewed for conceptual soundness?
- What was it designed to answer?
- What are its limitations and assumptions?
- How is it being used?
- Is it performing well?
- Does the organisation have sound and appropriate documentation?
Konstantina Armata: SR 11‑7 established model risk as equivalent to other risk types such as market and credit risk, requiring a comprehensive framework for ongoing management of the risk against defined limits and risk appetite. This is very different to the pre-SR 11‑7 era.
Javier Calvo Martín: Although some banks have seen beyond regulation when launching internal MRM programmes, it is fair to say SR 11‑7 has triggered most of the banks’ initial efforts in this domain in the US and also abroad. From Management Solutions’ perspective, the Fed and the Office of the Comptroller of the Currency (OCC) are carrying out essential work in responding to industry concerns – through industry conferences, for example. Furthermore, the influence of SR 11‑7 and SR 15‑18 in other countries’ regulation – for example, the Polish Financial Supervision Authority’s Recommendation W – and in banks’ best practices is remarkable.
Meanwhile, in the EU, MRM is being approached in a different manner, though not as specifically as in the US. SR 11‑7 has no equivalent and the ECB’s guide to internal models only states that “institutions should have an MRM framework in place that allows them to identify, understand and manage their model risk for internal models across the group”. The guide provides seven items that need to be covered, and has so far proceeded no further. In practice, the Trim project reviews many areas belonging to MRM frameworks – for example, model governance, organisation, documentation and policies – for Pillar 1 models.
Also noteworthy is the British Prudential Regulation Authority’s (PRA’s) success in enhancing MRM standards in the UK through a principles-based regulation on MRM, for now aimed at stress-testing models.
In other words, it is unquestionable that regulatory initiatives are effectively improving – or even triggering – MRM standards in banks. However, this is being undertaken from different perspectives: a top-down approach in the US and UK, starting with the need for an overall MRM framework and drilling down to specific components; and a bottom-up approach in continental Europe, with the Trim project assessing many MRM-related components, departing from each individual model under inspection, which should ultimately lead to building up a holistic MRM framework.
Slava Obraztsov: Since the introduction of SR 11‑7 in 2011, significant progress has been made in the development of MRM processes. This includes extending the scope of covered models, strengthening requirements for the quality of model development and validation documentations, introducing firm‑wide model governance frameworks – such as committees, policies, and so on – establishing model inventories, and supporting model management workflows.
Initially, MRM requirements mainly affected large firms regulated by the Fed and the OCC. However, other regulators – such as the ECB with Trim, and the PRA with SS3/18 – have gradually aligned their model requirements to the major elements of SR 11‑7. In some cases, regulators have adopted even stricter requirements.
I’ve found SR 11‑7 and Trim requirements slightly different. While the former is based on high-level principles around the three lines of defence and major MRM requirements, ECB requirements are more specific. The scope is narrower and applied only to different types of risk models, but there are more specific expectations of what types of analysis must be performed or what reporting provided. Despite those requirements being not yet fully finalised, some European regulators have started to apply them in their inspections of financial institutions.
Global financial organisations face quite a challenge to satisfy the requirements of different regulators, which are not always fully consistent. It is clear regulators would often like to see more region-specific approaches as this would allow them to have more control over applications of MRM framework. On the other hand, if MRM approaches become too fragmented, it would become impossible for international institutions to run them efficiently and consistently.
Anne-Cécile Krieg: SR 11-7 is a historical cornerstone of MRM and Trim is a relevant exercise, so both have proved to be the basis for internal review and improvement of our set-ups.
Which MRM areas offer the greatest potential to drive value and efficiency?

Konstantina Armata: The well-established ones such as MRM for valuation and RWA models. The know-how of these areas can be shared across other model types and testing automation can enable better utilisation of resources and cost reduction.
Ashutosh Nawani: As with any other strategic risk, the lifecycle of MRM offers opportunities for creation, enhancements and optimisation. Breaking it into granular components, organisations are thinking of the following key elements:
1. Globally, institutions are looking to re-examine their MRM function; the current operating model is deemed unsustainable, considering the ever-growing number of models, their increasing complexity and heightened regulatory expectations. In particular, the greatest areas to drive value and efficiency can be grouped into the following three categories. Banks want to embrace an intelligent risk-based approach to MRM operations. A risk-based MRM framework and related methodological approaches enable tailored oversight, rigour and levels of effort linked to the model materiality, complexity and the relative impact on the business. This forms the foundation for simplifying processes, eliminating excessive governance and enabling a more cost-effective, scalable operation.
2. Institutions aim to leverage technology to maximise automation opportunities. Industrialised model management processes that are conducive to automation and strive towards centralisation by implementing comprehensive workflow systems to manage MRM, model validation and development, and interactions across the model lifecycle to enable efficient alignment of MRM standards and practices across the organisation.
3. Institutions’ goals are shifting to create a sourcing talent strategy for capacity and scalability. They aim to create a talent strategy that minimises irregular workloads, scarcity of resources and mismatch of skill sets by building centres of excellence or shared services teams, complemented by managed services, to allow resource allocation synergies while maintaining consistent MRM practices.
Anne-Cécile Krieg: Basically, managing model risk avoids losses. More importantly, managing model risk paves the way for the future: with the acceleration of the digital transformation comes the management of the risks involved with new technologies.
David Asermely: SAS recently held an MRM customer connection event. Clients identified automated model documentation assistance as a major opportunity to improve efficiency. Implementing automated documentation requires a single source of truth and consistency across an organisation. Such an effort takes considerable time and resources. Reducing those manual efforts – performed by many PhD‑level individuals – immediately increases value and efficiency. Other potential areas for improvement include robust performance monitoring, automated model usage capture, flexible reporting and powerful network visualisations. These next-generation MRM tools minimise repetitive mundane tasks and allow organisations to dedicate more resources to high-value activities. They also provide important insight on how models are used and in what context. This information is becoming increasingly valuable as many model risk practitioners and regulators shift their focus from individual models to model ecosystems.
Slava Obraztsov: A proper MRM process around the full model lifecycle – including model design, development, validation, approval and reporting – should be able to significantly optimise model management and use, and should thus be considered a benefit, not a cost.
In particular, model quantification and model interconnectedness can drive value. In addition to my previous comments on these topics, I’d add that model interconnectedness should impact the whole model approval process. For example, consider a change of pricing model or a model introduced for a new product type: in this case it is not sufficient simply to understand how it would affect the value of the product or its hedging, but also the impact on other areas such as capital and exposure calculations, independent price verification processes in the finance area or liquidity risk management in the treasury. It should therefore be properly understood how changes to or the limitations of one model affect others. All of these elements should be combined into a single comprehensive product approval process. As previously discussed, robust model inventory and product taxonomy are key to the management of model interconnectedness.
To what extent have banks been able to take advantage of new technology and analytics such as machine learning?
David Asermely: Machine learning models are proving very valuable in model validation, feature selection and benchmarking. Yes, models validating models, or even models validating themselves. Of course, this complexity and lack of transparency adds another layer of risk, including the potential of survivor bias to propagate models that ‘lie’ about everything being fine. However, there are opportunities to better automate model validation and identify risks that humans would not be able to see.
Slava Obraztsov: Technological development as a whole has been very important for MRM. Some areas are becoming quite intensive and simultaneously customised – some elements of validation, reporting or monitoring, for example. With the proliferation of models, more tools need to be put in place just to run proper processes and controls. It includes appropriate inventory, building tools for performance monitoring and model risk analysis, and automation of generating model development and validation documentation.
There are many applications in MRM where machine learning can be deployed, typically for processing large and less-structured datasets. In particular, machine learning can improve the quality and consistency of input data. It could also be an important tool for stress testing, defining stress scenarios and propagating those scenarios across all underlying risk factors. If propagation is undertaken in a simplistic way, it may lead to inconsistent building of interest rate curves and volatility surfaces, which would lead to the breakdown of many underlying pricing models and an unreliable stress test output.
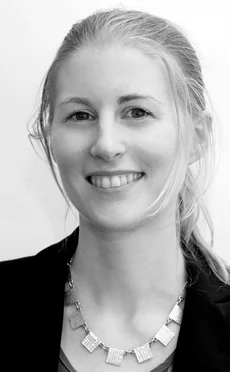
Anne-Cécile Krieg: New technology and analytics currently have two main applications. First, to develop models to optimise internal processes and some compliance-related topics. Second, to challenge existing models, particularly in terms of performance.
Ashutosh Nawani: Technology is at the heart of MRM. This is widely observed in different activities varying from the aim of a universal data system for enterprise MRM activities – development, validation and governance – to the consolidation of model risk assessment and issues management systems. The ultimate objective is optimised key processes across model risk capability areas with integrated data taxonomies for process, products, risks and controls. In other words, robotics process automation in monitoring, validation and testing, and reporting – and banks leveraging technologies and analytics in remediation processing.
Konstantina Armata: This is a very promising area, but is still in its infancy.
What are the main considerations in adopting these technologies?
Anne-Cécile Krieg: First and foremost, the MRM framework needs to be applied to all models, regardless of the technique – from classic statistical or stochastic approaches to new AI methodologies. This all begins with identifying the models and registering them within the model inventory. Regarding machine learning, specific considerations such as ‘over-fitting’ and black-box risks must be overseen and carefully monitored over time.
David Asermely: Machine learning models need governance just like any other models – only more so. Some machine learning models improve automatically through experience. This is a desirable feature, but it also exacerbates an organisation’s model risk. Thus, there is an increased need to define operating controls on inputs (data) and outputs (model results). The dynamic nature of these technologies requires more frequent performance monitoring, constant data review and benchmarking, better contextual model inventory understanding, and well thought out and actionable contingency plans. Machine learning models are rapidly moving toward broad financial industry adoption. These models offer better predictability potential in the face of a global, multidimensional marketplace and the increasing volume and complexity of data, but this complexity and lack of transparency threaten to overload traditional model risk guardrails and governance practices.
Konstantina Armata: It’s a paradigm shift. New skill-set and mind-set adaptation is required, as well as a functioning and efficient IT infrastructure.
Slava Obraztsov: It is often a matter of funding and resources. But these new technologies are unavoidable and will play an increasingly important role in financial modelling, including capital optimisation and algorithmic trading.
There are currently no industry standards for model validation and performance monitoring of machine learning models, and even regulatory requirements are not specific and are usually covered by general concepts such as ‘effective challenge’. Undoubtedly, we are going to witness active application of machine learning approaches to MRM in future, so I think more refined regulatory standards – but not necessarily new regulation – will need to be developed soon.
The questionnaire respondents were speaking in a personal capacity. The views expressed by the panel do not necessarily reflect or represent the views of their respective institutions.
Model risk management – Special report 2019
Read more
Sponsored content
Copyright Infopro Digital Limited. All rights reserved.
As outlined in our terms and conditions, https://www.infopro-digital.com/terms-and-conditions/subscriptions/ (point 2.4), printing is limited to a single copy.
If you would like to purchase additional rights please email info@risk.net
Copyright Infopro Digital Limited. All rights reserved.
You may share this content using our article tools. As outlined in our terms and conditions, https://www.infopro-digital.com/terms-and-conditions/subscriptions/ (clause 2.4), an Authorised User may only make one copy of the materials for their own personal use. You must also comply with the restrictions in clause 2.5.
If you would like to purchase additional rights please email info@risk.net