Journal of Risk
ISSN:
1465-1211 (print)
1755-2842 (online)
Editor-in-chief: Farid AitSahlia
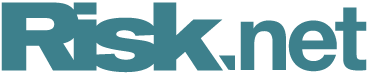
Nonparametric estimation of copulas for time series
Jean-David Fermanian, Olivier Scaillet
Abstract
ABSTRACT
We consider a nonparametric method to estimate copulas, ie, functions linking joint distributions to their univariate margins. We derive the asymptotic properties of kernel estimators of copulas and their derivatives in the context of a multivariate stationary process satisfying strong mixing conditions. Monte Carlo results are reported for a stationary vector autoregressive process of order one with Gaussian innovations. An empirical illustration containing a comparison with the independent, comotonic and Gaussian copulas is given for European and US stock index returns.
Copyright Infopro Digital Limited. All rights reserved.
As outlined in our terms and conditions, https://www.infopro-digital.com/terms-and-conditions/subscriptions/ (point 2.4), printing is limited to a single copy.
If you would like to purchase additional rights please email info@risk.net
Copyright Infopro Digital Limited. All rights reserved.
You may share this content using our article tools. As outlined in our terms and conditions, https://www.infopro-digital.com/terms-and-conditions/subscriptions/ (clause 2.4), an Authorised User may only make one copy of the materials for their own personal use. You must also comply with the restrictions in clause 2.5.
If you would like to purchase additional rights please email info@risk.net