Journal of Risk
ISSN:
1465-1211 (print)
1755-2842 (online)
Editor-in-chief: Farid AitSahlia
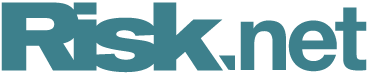
Explainable artificial intelligence for credit scoring in banking
Borger Melsom, Christian Bakke Vennerød, Petter Eilif de Lange, Lars Ole Hjelkrem and Sjur Westgaard
Need to know
- This study uses XAI methods in banking, exploring how these methods can be applied to improve the interpretability and reliability of state-of-the-art ML models.
- A LightGBM model is combined with SHAP, which enables the interpretation of explanatory variables affecting the predictions.
- it is proposed that LightGBM models may outperform logistic regression models for credit scoring both in terms of predictive performance and explainability.
Abstract
This paper proposes an explainable machine learning model for predicting credit default using a real-world data set provided by a Norwegian bank. We combine a Light Gradient Boosting Machine (LightGBM) model with Shapley additive explanations (SHAP), an explainable artificial intelligence (XAI) framework that enables the interpretation of explanatory variables affecting the predictions. Using the Light- GBM model, we achieve a 1% increase in the area under the receiver operating characteristic curve and a 19% increase in the area under the precision recall curve compared with an industry-standard logistic regression model. An empirical analysis using SHAP on the explanatory variables is also conducted. Our main contribution is the exploration of how the implementation of XAI methods can be applied in banking to improve the interpretability and reliability of state-of-the-art machine learning models. We specifically find that LightGBM models may outperform logistic regression models for credit scoring in terms of both predictive performance and explainability.
Copyright Infopro Digital Limited. All rights reserved.
You may share this content using our article tools. Printing this content is for the sole use of the Authorised User (named subscriber), as outlined in our terms and conditions - https://www.infopro-insight.com/terms-conditions/insight-subscriptions/
If you would like to purchase additional rights please email info@risk.net
Copyright Infopro Digital Limited. All rights reserved.
You may share this content using our article tools. Copying this content is for the sole use of the Authorised User (named subscriber), as outlined in our terms and conditions - https://www.infopro-insight.com/terms-conditions/insight-subscriptions/
If you would like to purchase additional rights please email info@risk.net