Journal of Risk
ISSN:
1465-1211 (print)
1755-2842 (online)
Editor-in-chief: Farid AitSahlia
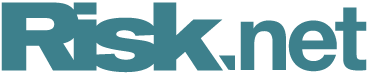
A two-component realized exponential generalized autoregressive conditional heteroscedasticity model
Xinyu Wu, Michelle Xia and Huanming Zhang
Need to know
- A two-component realized EGARCH model is proposed for modelling and forecasting volatility.
- The model is capable of capturing the long memory property of volatility.
- The model is easy to implement using the maximum likelihood method.
- The model as well as a restricted version of the model outperform many competitor models in both in-sample fit and out-of-sample forecast.
Abstract
This paper proposes a two-component realized exponential generalized autoregressive conditional heteroscedasticity (EGARCH) model – an extension of the realized EGARCH model – for the joint modeling of asset returns and realized measures of volatility. The proposed model assumes that the volatility of asset returns consists of two components: a long-run component and a short-run component. The model’s unique ability to capture the long-memory property of volatility distinguishes it from the standard realized EGARCH model. The model is convenient to implement within the framework of maximum likelihood estimation. We apply the two-component realized EGARCH model and a restricted version of the model (the two-component realized EGARCH model with only short-run leverage) to four stock indexes: the Standard & Poor’s 500 index, the Hang Seng index, the Nikkei 225 index and the Deutscher Aktienindex. The empirical study suggests that the two-component realized EGARCH model and its restricted version outperform the realized GARCH model, the realized EGARCH model and the realized heterogeneous autoregressive GARCH model in terms of in-sample fit. Further, an out-of-sample predictive analysis demonstrates that the two-component realized EGARCH model and its restricted version yield more accurate volatility forecasts than the alternatives.
Copyright Infopro Digital Limited. All rights reserved.
As outlined in our terms and conditions, https://www.infopro-digital.com/terms-and-conditions/subscriptions/ (point 2.4), printing is limited to a single copy.
If you would like to purchase additional rights please email info@risk.net
Copyright Infopro Digital Limited. All rights reserved.
You may share this content using our article tools. As outlined in our terms and conditions, https://www.infopro-digital.com/terms-and-conditions/subscriptions/ (clause 2.4), an Authorised User may only make one copy of the materials for their own personal use. You must also comply with the restrictions in clause 2.5.
If you would like to purchase additional rights please email info@risk.net