Journal of Risk
ISSN:
1465-1211 (print)
1755-2842 (online)
Editor-in-chief: Farid AitSahlia
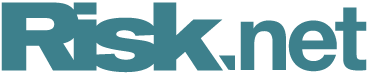
Covariance estimation for risk-based portfolio optimization: an integrated approach
Need to know
- We provide an integrated prediction and optimization (IPO) framework for covariance model parameter estimation.
- We consider minimum-variance, maximum-diversification and equal-risk-contribution portfolio models.
- IPO covariance models are compared to traditional "predict, then optimize" models based on OLS and maximum-likelihood.
- IPO models explicitly minimize decision error and can result in lower out-of-sample nominal costs and improved economic outcomes.
Abstract
Many problems in quantitative finance involve both predictive forecasting and decision-based optimization. Traditionally, covariance forecasting models are optimized with unique prediction-based objectives and constraints, and users are therefore unaware of how these predictions would ultimately be used in the context of the models’ final decision-based optimization. We present a stochastic optimization framework for integrating time-varying factor covariance models in a risk-based portfolio optimization setting. We make use of recent advances in neural-network architecture for efficient optimization of batch convex programs. We consider three risk-based portfolio optimizations: minimum variance, maximum diversification and equal risk contribution, and we provide a first-order method for performing the integrated optimization. We present several historical simulations using US industry and stock data, and we demonstrate the benefits of the integrated approach in comparison with the decoupled alternative.
Copyright Infopro Digital Limited. All rights reserved.
You may share this content using our article tools. Printing this content is for the sole use of the Authorised User (named subscriber), as outlined in our terms and conditions - https://www.infopro-insight.com/terms-conditions/insight-subscriptions/
If you would like to purchase additional rights please email info@risk.net
Copyright Infopro Digital Limited. All rights reserved.
You may share this content using our article tools. Copying this content is for the sole use of the Authorised User (named subscriber), as outlined in our terms and conditions - https://www.infopro-insight.com/terms-conditions/insight-subscriptions/
If you would like to purchase additional rights please email info@risk.net