Journal of Risk
ISSN:
1465-1211 (print)
1755-2842 (online)
Editor-in-chief: Farid AitSahlia
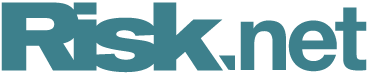
Risk measures: a generalization from the univariate to the matrix-variate
María A. Arias-Serna, Francisco J. Caro-Lopera and Jean-Michel Loubes
Need to know
- This paper proposes a method to calculate matrix-variate value-at-risk.
- This paper develops a method for estimating the value-at-risk and the conditional value-at-risk when the underlying risk factors follow a beta distribution in a univariate and matrix-variate setting.
- Analytical expressions of the risk measures are developed.
- A numerical solution for the risk measures for any parameterization of beta distributed loss variables is presented.
- Of fundamental importance is the application of computer-based algorithms for solving classically analytic problems in financial risk management. The data we acquired from Colombian financial institutions are considered using both algorithmic and analytic methods. Our results demonstrate a correspondence between the two. Although our results are motivated by problems in finance, we believe that our methods may well more general applications as well.
Abstract
This paper develops a method for estimating value-at-risk and conditional value-at-risk when the underlying risk factors follow a beta distribution in a univariate and a matrix-variate setting. For this purpose, we connect the theory of the Gaussian hypergeometric function of matrix argument and integration over positive definite matrixes. For certain choices of the shape parameters, a and b, analytical expressions of the risk measures are developed. More generally, a numerical solution for the risk measures for any parameterization of beta-distributed loss variables is presented. The proposed risk measures are finally used for quantifying the potential risk of economic loss in credit risk.
Copyright Infopro Digital Limited. All rights reserved.
As outlined in our terms and conditions, https://www.infopro-digital.com/terms-and-conditions/subscriptions/ (point 2.4), printing is limited to a single copy.
If you would like to purchase additional rights please email info@risk.net
Copyright Infopro Digital Limited. All rights reserved.
You may share this content using our article tools. As outlined in our terms and conditions, https://www.infopro-digital.com/terms-and-conditions/subscriptions/ (clause 2.4), an Authorised User may only make one copy of the materials for their own personal use. You must also comply with the restrictions in clause 2.5.
If you would like to purchase additional rights please email info@risk.net