Journal of Risk
ISSN:
1465-1211 (print)
1755-2842 (online)
Editor-in-chief: Farid AitSahlia
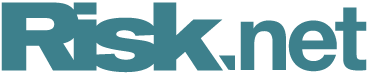
Standard errors of risk and performance estimators for serially dependent returns
Need to know
- Classical standard errors (SE’s) of risk and performance estimators are often quite negatively biased, and confidence interval error rates are correspondingly too high, when returns have seemingly small positive serial correlation.
- The above problem can be avoided by using a new frequency domain method of accurately computing standard errors when returns are serially dependent, as well as when they are independent. The method makes novel use of statistical influence functions borrowed from robust statistics, combined with regularized generalized linear model (GLM) fitting for exponential distributions.
- The efficacy of the method is demonstrated by application to hedge funds data, and by Monte Carlo confirmation of accurate Sharpe ratio SE’s and correspondingly on-target confidence interval error rates.
- The method has been implemented in the risk and performance estimator standard errors open source R package RPESE available at https://cran.r-project.org/.
Abstract
In this paper, a new method for computing the standard errors (SEs) of returns-based risk and performance estimators for serially dependent returns is developed. The method uses both the fact that any such estimator can be represented as the mean of returns that are transformed using the estimator’s influence function (IF), and the fact that the variance of such a sum can be estimated by estimating the zero-frequency value of the spectral density of the IF transformed returns. The spectral density is estimated by fitting a polynomial to the periodogram using a generalized linear model for exponential distributions, with elastic net regularization. We study the use of the new SEs method with and without prewhitening. Applications to computing the SE of Sharpe ratio (SR) estimators for a collection of hedge funds, whose returns have varying degrees of serial dependence, show that the new methods are a considerable improvement on SE methods based on assumed independent and identically distributed returns, and that the prewhitening version performs better than the one without prewhitening. Monte Carlo simulations are conducted to study (i) the mean-squared error performance of the SE methods for a number of commonly used risk and performance estimators for first-order autoregression and GARCH(1,1) returns models; and (ii) the SR confidence interval error rate performance for first-order autoregression models with normal and t-distribution innovations. The results show that our new method is a considerable improvement on both earlier frequency domain methods and the Newey–West heteroscedasticity and autocorrelation consistent (HAC) SE method.
Copyright Infopro Digital Limited. All rights reserved.
You may share this content using our article tools. Printing this content is for the sole use of the Authorised User (named subscriber), as outlined in our terms and conditions - https://www.infopro-insight.com/terms-conditions/insight-subscriptions/
If you would like to purchase additional rights please email info@risk.net
Copyright Infopro Digital Limited. All rights reserved.
You may share this content using our article tools. Copying this content is for the sole use of the Authorised User (named subscriber), as outlined in our terms and conditions - https://www.infopro-insight.com/terms-conditions/insight-subscriptions/
If you would like to purchase additional rights please email info@risk.net