Journal of Risk
ISSN:
1465-1211 (print)
1755-2842 (online)
Editor-in-chief: Farid AitSahlia
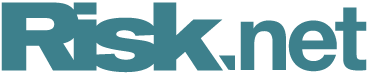
Genetic algorithm-based portfolio optimization with higher moments in global stock markets
Need to know
- The distributional characteristics of the stock market returns are analysed.
- The importance of higher moments in emerging as well as in developed markets are investigated
- Higher moment’s model outperforms the traditional mean variance model especially in emerging markets.
Abstract
Markowitz’s mean–variance portfolio model is widely used in the field of investment management. The changing dynamics of markets have resulted in higher uncertainties surrounding returns. Returns have often been found to be skewed and extreme events observed to be frequent. These characteristics are measured by skewness and kurtosis, which need to be accommodated in the definition of risk. They should also be included in the portfolio optimization process. The purpose of this paper is to investigate the impact of including higher moments in the estimation of risk in the process of international portfolio diversification. Our study is based on a sample of thirty-three globally traded stock market indexes, including emerging as well as developed markets, for the period between 2000 and 2012. Our inclusion of skewness and kurtosis makes portfolio optimization a nonlinear, nonconvex and multi-objective problem; this has been solved with the use of a genetic algorithm. Empirical results demonstrate that the higher moments model outperforms the traditional mean–variance model across the time period. The results of this study may be useful to fund managers, portfolio managers and investors, aiding them in understanding the behavior of the stock market and in selecting an optimal portfolio model among various alternative portfolio models.
Copyright Infopro Digital Limited. All rights reserved.
As outlined in our terms and conditions, https://www.infopro-digital.com/terms-and-conditions/subscriptions/ (point 2.4), printing is limited to a single copy.
If you would like to purchase additional rights please email info@risk.net
Copyright Infopro Digital Limited. All rights reserved.
You may share this content using our article tools. As outlined in our terms and conditions, https://www.infopro-digital.com/terms-and-conditions/subscriptions/ (clause 2.4), an Authorised User may only make one copy of the materials for their own personal use. You must also comply with the restrictions in clause 2.5.
If you would like to purchase additional rights please email info@risk.net