Journal of Risk Model Validation
ISSN:
1753-9579 (print)
1753-9587 (online)
Editor-in-chief: Steve Satchell
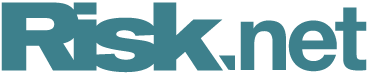
Beyond the contract: client behavior from origination to default as the new set of the loss given default risk drivers
Need to know
- This paper introduces a new set of client behavior based predictors.
- Parametric and non-parametric models are prepared for the recovery rate estimation.
- Adding client related variables reduces the errors and improve discrimination.
- The effect is more visible for fractional regression rather than regression tree.
Abstract
Modeling loss given default has increased in popularity as it has become a crucial parameter for establishing capital buffers under Basel II and III and for calculating the impairment of financial assets under the International Financial Reporting Standard 9. The most recent literature on this topic focuses mainly on estimation methods and less on the variables used to explain the variability in loss given default. In this paper, we expand this part of the modeling process by constructing a set of client-behavior-based predictors that can be used to construct more precise models, and we investigate the economic justifications empirically to examine their potential usage. The main novelty introduced in this paper is the connection between loss given default and the behavior of the contract owner, not just the contract itself. This approach results in the reduction of the values of selected error measures and progressively improves the forecasting ability. The effect is more visible in a parametric method (fractional regression) than in a nonparametric method (regression tree). Our findings support incorporating client-oriented information into loss given default models.
Copyright Infopro Digital Limited. All rights reserved.
As outlined in our terms and conditions, https://www.infopro-digital.com/terms-and-conditions/subscriptions/ (point 2.4), printing is limited to a single copy.
If you would like to purchase additional rights please email info@risk.net
Copyright Infopro Digital Limited. All rights reserved.
You may share this content using our article tools. As outlined in our terms and conditions, https://www.infopro-digital.com/terms-and-conditions/subscriptions/ (clause 2.4), an Authorised User may only make one copy of the materials for their own personal use. You must also comply with the restrictions in clause 2.5.
If you would like to purchase additional rights please email info@risk.net