Journal of Operational Risk
ISSN:
1744-6740 (print)
1755-2710 (online)
Editor-in-chief: Marcelo Cruz
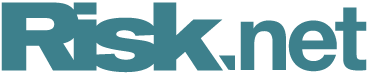
Preventing the unpleasant: fraudulent financial statement detection using financial ratios
Michail Pazarskis, Grigorios Lazos, Andreas G. Koutoupis and George Drogalas
Need to know
- The proposed model achieves accuracy in the prediction of fraudulent financial statements with an accuracy rate of over 78%.
- The proposed model contains two ratios that could serve as “red flags” in an audit process: “collection period” and “gearing”.
- The companies without falsification show better results in their financial ratios than those companies that present falsification in their financial statements.
- The proposed model could be used as an effective tool to detect fraudulent financial statements by the banking system, internal and external auditors, and tax authorities.
Abstract
The aim of this study is to investigate financial fraud in companies listed on the Athens Stock Exchange during the period 2008–18, in which a major economic crisis took place in Greece. Based on 30 financial indicators resulting from the analysis of financial statements, several statistical tests are applied to the primary sample and the control sample in order to create a model that uses the indicators as “forecasts” to detect possible fraud. The data used in the research were obtained from the financial statements of the listed companies, the reviews in the auditors’ reports and the available data and information from the reports of the Athens Stock Exchange. The proposed model is able to correctly classify the total sample with an accuracy of 78.4%. The results of the research show that the model works effectively in detecting fraudulent financial statements when the economy is operating in crisis conditions. By using financial ratios, this model signals red flags for the audit process, and it could be used as an effective tool by the banking system, internal and external auditors, tax authorities and other government authorities.
Copyright Infopro Digital Limited. All rights reserved.
As outlined in our terms and conditions, https://www.infopro-digital.com/terms-and-conditions/subscriptions/ (point 2.4), printing is limited to a single copy.
If you would like to purchase additional rights please email info@risk.net
Copyright Infopro Digital Limited. All rights reserved.
You may share this content using our article tools. As outlined in our terms and conditions, https://www.infopro-digital.com/terms-and-conditions/subscriptions/ (clause 2.4), an Authorised User may only make one copy of the materials for their own personal use. You must also comply with the restrictions in clause 2.5.
If you would like to purchase additional rights please email info@risk.net