Journal of Operational Risk
ISSN:
1744-6740 (print)
1755-2710 (online)
Editor-in-chief: Marcelo Cruz
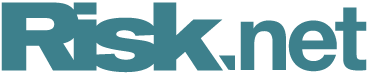
Estimation of value-at-risk for conduct risk losses using pseudo-marginal Markov chain Monte Carlo
Peter Mitic and Jiaqi Hu
Need to know
- If the largest loss is an aggregation of an unknown number of multiple smaller losses, and is extremely large, VaR is inflated unacceptably.
- The Pseudo Marginal method is a way to estimate VaR by partitioning the largest loss in order to simulate its constituent components.
- As the number of partitions increases, the VaR estimate approaches a limit, usually reached between 3000 and 5000 partitions.
- The calculations are sensitive to initial conditions and can take time. For 1000 partitions or fewer the time taken to estimate VaR is about a few minutes, but for 4000 or more partitions it can exceed 2 hours.
Abstract
We propose a model for conduct risk losses, in which conduct risk losses are characterized by having a small number of extremely large losses (perhaps only one) with more numerous smaller losses. It is assumed that the largest loss is actually a provision from which payments to customers are made periodically as required. We use the pseudo-marginal (PM) Markov chain Monte Carlo method to decompose the largest loss into smaller partitions in order to estimate 99.9% value-at-risk. The par-titioning is done in a way that makes no assumption about the size of the partitions. The advantages and problems of using this method are discussed. The PM procedures were run on several representative data sets. The results indicate that, in cases where using approaches such as calculating a Monte Carlo-derived loss distribution yields a result that is not consistent with the risk profile expressed by the data, using the PM method yields results that have the required consistency.
Copyright Infopro Digital Limited. All rights reserved.
You may share this content using our article tools. Printing this content is for the sole use of the Authorised User (named subscriber), as outlined in our terms and conditions - https://www.infopro-insight.com/terms-conditions/insight-subscriptions/
If you would like to purchase additional rights please email info@risk.net
Copyright Infopro Digital Limited. All rights reserved.
You may share this content using our article tools. Copying this content is for the sole use of the Authorised User (named subscriber), as outlined in our terms and conditions - https://www.infopro-insight.com/terms-conditions/insight-subscriptions/
If you would like to purchase additional rights please email info@risk.net