Journal of Operational Risk
ISSN:
1744-6740 (print)
1755-2710 (online)
Editor-in-chief: Marcelo Cruz
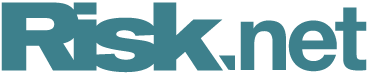
The use of business intelligence and predictive analytics in detecting and managing occupational fraud in Nigerian banks
Chioma N. Nwafor, Obumneme Z. Nwafor and Chris Onalo
Need to know
- Analysis of the frauds and forgeries returns reported by Nigeria Banks show that 2008 and 2016 had the highest frequency of frauds. Both years recorded 16,783 and 16,751 cases of frauds respectively. In terms of the severity, 2009 recorded the highest loss at
N37,179,898.00 followed by 2008 with a loss severity ofN34,311,721.00.
- Although the banking industry has witnessed a downward trajectory in loss severity during the past four years, the increasing frequency of fraud occurrence suggests the existence of a ‘fraud path’- i.e. loophole in the detection and prevention systems of banks.
- Despite the fidelity bond insurance cover taken by deposit money banks to address the challenges of occupational frauds committed by staff, and the use of manual transactions monitoring approaches, available evidence suggests rising cases of occupational frauds in the industry.
- The current study proposes a two-fold approach to tackling occupational frauds within the Nigeria banking landscape- the use of data mining/business intelligence (outlier detection system) which aims to learn from historical transaction information in order to retrieve patterns that allow differentiating between normal and abnormal activities and predictive analytics techniques along with continuous monitoring of transactional data.
Abstract
The problem of occupational fraud is one of the most wide-reaching operational risk event types in the Nigerian banking system. This event type spans many departments, roles, processes and systems and causes significant financial and reputational damage to banks. As a result, fraud presents banks with a real challenge in terms of knowing where to start. One of the main aims of this paper is to use stochastic probability models to predict aggregate fraud severity and fraud frequency within the Nigerian banking sector using historical data. Another objective is to describe how banks can develop and deploy business intelligence (BI) outlier-based detection models to recognize internal fraudulent activities. As the volume of transaction data grows and the industry focuses more closely on fraud detection, BI has evolved to provide proactive, real-time insights into fraudulent behaviors and activities. We discuss the fraud analytic development process, since it is a central issue in real application domains.
Copyright Infopro Digital Limited. All rights reserved.
As outlined in our terms and conditions, https://www.infopro-digital.com/terms-and-conditions/subscriptions/ (point 2.4), printing is limited to a single copy.
If you would like to purchase additional rights please email info@risk.net
Copyright Infopro Digital Limited. All rights reserved.
You may share this content using our article tools. As outlined in our terms and conditions, https://www.infopro-digital.com/terms-and-conditions/subscriptions/ (clause 2.4), an Authorised User may only make one copy of the materials for their own personal use. You must also comply with the restrictions in clause 2.5.
If you would like to purchase additional rights please email info@risk.net