Journal of Investment Strategies
ISSN:
2047-1238 (print)
2047-1246 (online)
Editor-in-chief: Ali Hirsa
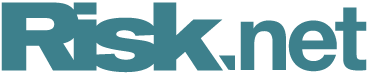
Option strategies based on semiparametric implied volatility surface prediction
Francesco Audrino and Dominik Colangelo
Abstract
ABSTRACT
This paper investigates whether a more sophisticated technique that is able to accurately forecast the future movements of the implied volatility surface can help to improve the performance of basic option strategies. To this end, we construct a set of strategies using predicted option returns for a forecasting period of ten trading days and form profitable hold-to-expiration, equally weighted, zerocost portfolios with one-month at-the-money options. The accurate predictions of the implied volatility surface dynamics are obtained using a statistical machinelearning procedure based on regression trees. These forecasts assist in obtaining reliable option returns used as trading signals in our strategies.We test the performance of the proposed strategies on options on the S&P 100 and its constituents between 2002 and 2006, obtaining positive annualized returns of up to more than 50%. Comparing such a performance with those obtained without using any complex model for the implied volatility surface, we show that, in most cases, differences are small.
Copyright Infopro Digital Limited. All rights reserved.
You may share this content using our article tools. Printing this content is for the sole use of the Authorised User (named subscriber), as outlined in our terms and conditions - https://www.infopro-insight.com/terms-conditions/insight-subscriptions/
If you would like to purchase additional rights please email info@risk.net
Copyright Infopro Digital Limited. All rights reserved.
You may share this content using our article tools. Copying this content is for the sole use of the Authorised User (named subscriber), as outlined in our terms and conditions - https://www.infopro-insight.com/terms-conditions/insight-subscriptions/
If you would like to purchase additional rights please email info@risk.net