Journal of Financial Market Infrastructures
ISSN:
2049-5404 (print)
2049-5412 (online)
Editor-in-chief: Manmohan Singh
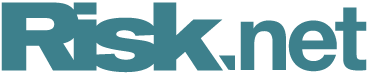
How much liquidity would a liquidity-saving mechanism save if a liquidity-saving mechanism could save liquidity? A simulation approach for Canada’s large-value payment system
Need to know
- Canada is replacing its current wholesale payment system, the LVTS, with a real-time gross settlement (RTGS) system
- We simulate the impact of moving to an RTGS system with one or more LSMs
- The results show that LSMs have the potential to improve the trade-off between intraday liquidity and settlement delay
Abstract
Canada’s Large Value Transfer System (LVTS) is in the process of being replaced by a real-time gross settlement (RTGS) system. A pure RTGS system typically requires participants to hold substantial amounts of intraday liquidity in order to settle their payment obligations. Implementing one or more liquidity-saving mechanisms (LSMs) can reduce the amount of liquidity that participants need to hold. This paper investigates how much liquidity requirements can be reduced with the implementation of different LSMs in the Financial Network Analytics simulation engine using LVTS transaction data from 2018. These LSMs include (1) bilateral offsetting, (2) FIFO–bypass, (3) multilateral offsetting and (4) a combination of all LSMs. We simulate two different scenarios at varying levels of the upper bound of liquidity. In the first scenario, all payments from Tranche 1, which are considered time critical, are settled in a pure RTGS payment stream, while less time-critical Tranche 2 payments are settled in a payment stream with LSMs. In the second scenario, we settle all payments (Tranches 1 and 2) in the LSM stream. Our results show that when there is ample liquidity available in the system, there is minimal benefit from LSMs, as payments are settled without much delay: the effectiveness of LSMs increases as the amount of intraday liquidity decreases. A combination of LSMs shows a reduction in liquidity requirements that is larger than any one individual LSM.
Copyright Infopro Digital Limited. All rights reserved.
You may share this content using our article tools. Printing this content is for the sole use of the Authorised User (named subscriber), as outlined in our terms and conditions - https://www.infopro-insight.com/terms-conditions/insight-subscriptions/
If you would like to purchase additional rights please email info@risk.net
Copyright Infopro Digital Limited. All rights reserved.
You may share this content using our article tools. Copying this content is for the sole use of the Authorised User (named subscriber), as outlined in our terms and conditions - https://www.infopro-insight.com/terms-conditions/insight-subscriptions/
If you would like to purchase additional rights please email info@risk.net