Journal of Energy Markets
ISSN:
1756-3607 (print)
1756-3615 (online)
Editor-in-chief: Kostas Andriosopoulos
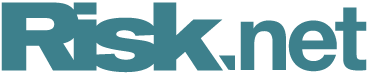
The relationship between oil prices, global economic policy uncertainty and financial market stress
Need to know
- The NARDL technique confirms the long-run asymmetric relationship between global economic policy uncertainty, gold prices and oil prices.
- Monetary policy influence on oil prices limited to negative impacts of it in the long-run
- The results indicate long- and short-run relationships between negative OP and financial stress index
- Oil prices inversely impact the financial stress index in the short and long runs
Abstract
This paper introduces two models. The first model analyzes the impacts of global economic policy uncertainty (EPU), gold prices (GPs) and three-month US Treasury bill (TB) rates on oil prices (OPs) between 1997 and 2020. The second model examines the effects of OPs and US TB rates on the Püttmann Financial Stress Indicator (FSI) between 1979 and 2016. To capture the long- and short-run relationships between independent and dependent variables, an asymmetric nonlinear autoregressive distributed lag model is employed for both models. The results indicate that the effects of the independent variables are long term only. The results confirm the significant impact of global EPU and GPs on OPs in the long run. For the second model, OPs are the determining variable to explain financial stress in both the long run and the short run. Although the negative fluctuations in OPs have a positive effect on the FSI in the short run, their impacts on the FSI turn negative in the long run. The overall results underscore how OPs are affected by uncertainty and how they influence the FSI.
Copyright Infopro Digital Limited. All rights reserved.
As outlined in our terms and conditions, https://www.infopro-digital.com/terms-and-conditions/subscriptions/ (point 2.4), printing is limited to a single copy.
If you would like to purchase additional rights please email info@risk.net
Copyright Infopro Digital Limited. All rights reserved.
You may share this content using our article tools. As outlined in our terms and conditions, https://www.infopro-digital.com/terms-and-conditions/subscriptions/ (clause 2.4), an Authorised User may only make one copy of the materials for their own personal use. You must also comply with the restrictions in clause 2.5.
If you would like to purchase additional rights please email info@risk.net