Journal of Credit Risk
ISSN:
1744-6619 (print)
1755-9723 (online)
Editor-in-chief: Linda Allen and Jens Hilscher
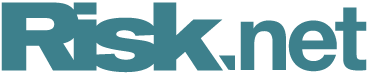
An effective credit rating method for corporate entities using machine learning
Hansheng Sun, Roy H. Kwon, Binbin Dai and Pubudu Premawardena
Need to know
- A new approach to design credit risk rating models for corporate entities is introduced. This approach allows a bank to make accurate and cost-effective rating decisions by maximizing risk adjusted return under model uncertainty.
- We propose a meta-algorithm, which effectively utilizes ordinal information embedded in the expert assigned credit ratings to accurately rank the customers. Then, a cost-sensitive rating assignment method is used to reduce the impact of model uncertainty on the bank’s risk adjusted return.
- We provide detailed discussions on specifying the cost matrix under the Basel Regulatory Framework. Empirical results on North American medium and large sized companies show strong performance of the proposed approach.
Abstract
In this study we introduce a new approach to designing credit risk rating models for corporate entities. This approach allows a bank to make accurate and cost-effective rating decisions by maximizing risk-adjusted returns under model uncertainty. We propose a meta-algorithm, which exploits the ordinal information embedded in the expert-assigned credit ratings in order to accurately rank customers. Then, a costsensitive rating-assignment method is used to reduce the impact of model uncertainty on the bank’s risk-adjusted return. We provide detailed discussions on specifying the cost matrix under the Basel regulatory framework. Empirical results for North American large and medium-sized companies show strong performance from the proposed approach.
Copyright Infopro Digital Limited. All rights reserved.
As outlined in our terms and conditions, https://www.infopro-digital.com/terms-and-conditions/subscriptions/ (point 2.4), printing is limited to a single copy.
If you would like to purchase additional rights please email info@risk.net
Copyright Infopro Digital Limited. All rights reserved.
You may share this content using our article tools. As outlined in our terms and conditions, https://www.infopro-digital.com/terms-and-conditions/subscriptions/ (clause 2.4), an Authorised User may only make one copy of the materials for their own personal use. You must also comply with the restrictions in clause 2.5.
If you would like to purchase additional rights please email info@risk.net